Blur-Robust Object Detection Using Feature-Level Deblurring via Self-Guided Knowledge Distillation
IEEE ACCESS(2022)
摘要
Images captured from real-world environments often include blur artifacts resulting from camera movement, dynamic object motion, or out-of-focus. Although such blur artifacts are inevitable, most object detection methods do not have special considerations for them; therefore, they may fail to detect objects in blurry images. One possible solution is applying image deblurring prior to object detection. However, this solution is computationally demanding and its performance heavily depends on image deblurring results. In this study, we propose a novel blur-aware object detection framework. First, we construct a synthetic but realistic dataset by applying a diverse set of motion blur kernels to blur-free images. Subsequently, we leverage self-guided knowledge distillation between the teacher and student networks that perform object detection using blur-free and blurry images, respectively. The teacher and student networks share most of their network parameters and jointly learn in a fully-supervised manner. The teacher network provides image features as hints for feature-level deblurring and also renders soft labels for the training of the student network. Guided by the hints and the soft labels from the teacher, the student network learns and expands their knowledge on object detection in blurry images. Experimental results show that the proposed framework improves the robustness of several widely used object detectors against image blurs.
更多查看译文
关键词
Object detection, Feature extraction, Detectors, Image restoration, Training, Kernel, Knowledge engineering, Object detection, deblurring, convolutional neural network, deep learning
AI 理解论文
溯源树
样例
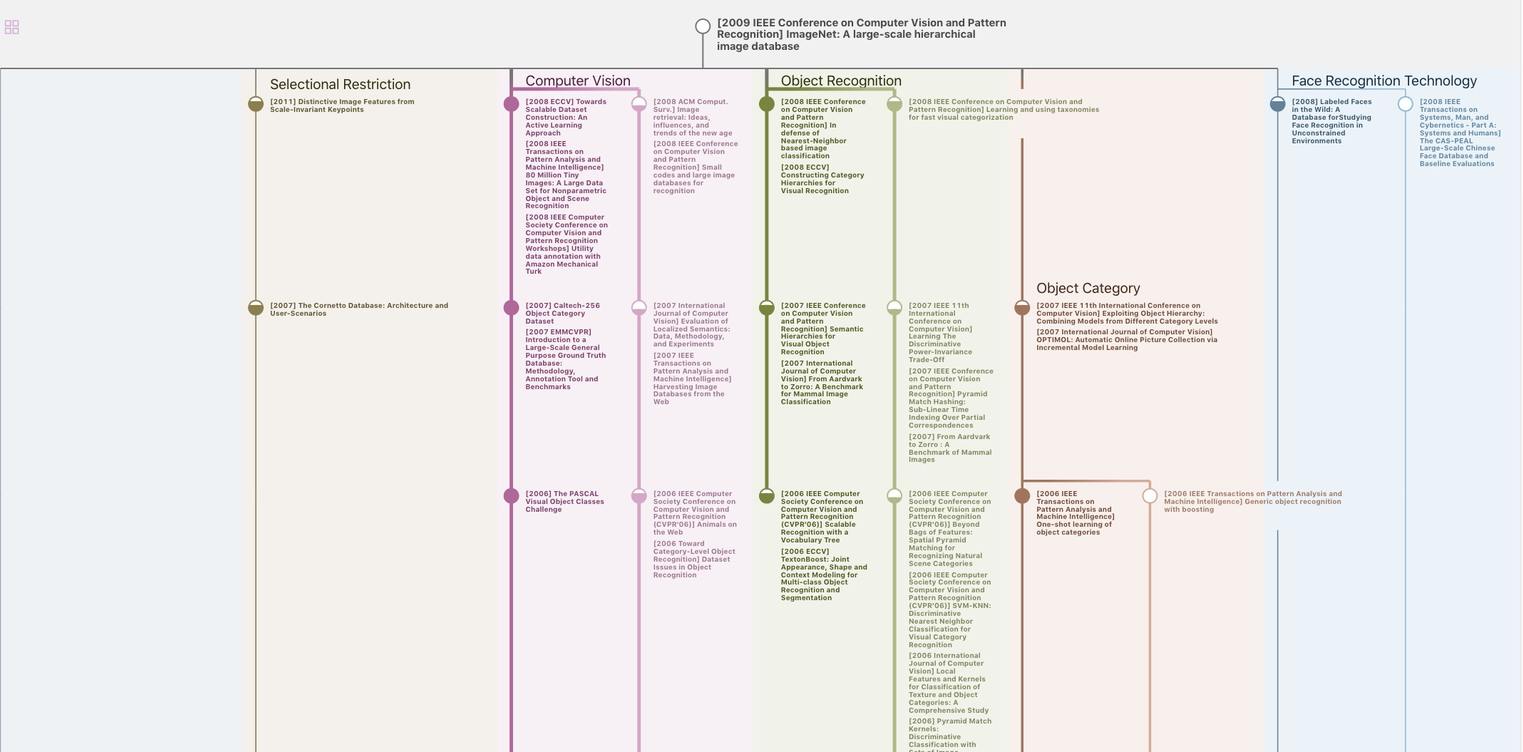
生成溯源树,研究论文发展脉络
Chat Paper
正在生成论文摘要