RNN Based Prediction of Path Loss Fading Distribution by Interval Estimation
2022 16TH EUROPEAN CONFERENCE ON ANTENNAS AND PROPAGATION (EUCAP)(2022)
摘要
We report a method for predicting fading distribution of path loss using GRU (Gated Recurrent Unit), which is a RNN (Recurrent Neural Network) as deep learning. The training data and validation data use path loss measured in Yokosuka City, Kanagawa Prefecture, Japan, and the measurement frequency is 4.7 GHz. By using 100 points of fast fading data about every 0.1 seconds, the median data of path loss and the K factor of Nakagami-Rice distribution after 1 second were predicted. The median data and the K factor are derived using the fast fading data of 100 points (about 10 seconds). According to the prediction method using GRU, the RMSE (Root Mean Squared Error) for the validation data is about 1.7 dB for the median path loss and about 0.5 dB for the K factor. The prediction accuracy was improved by 0.9 and 0.1 dB compared with the case of using latest observed values.
更多查看译文
关键词
Deep learning, RNN, GRU, path loss, Nakagami-Rice distribution, K factor
AI 理解论文
溯源树
样例
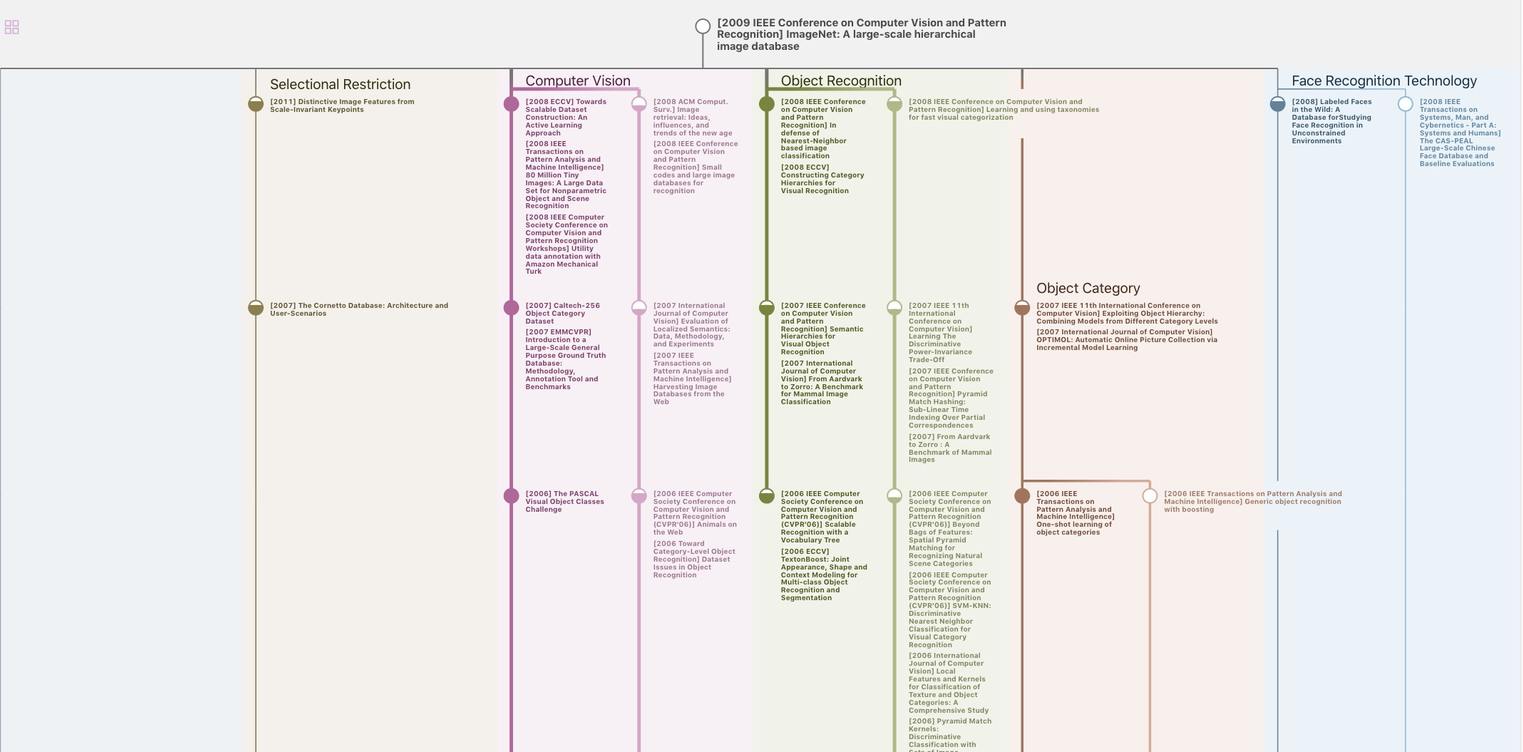
生成溯源树,研究论文发展脉络
Chat Paper
正在生成论文摘要