Quantification of Predictive Uncertainty via Inference-Time Sampling.
UNSURE@MICCAI(2022)
摘要
Predictive variability due to data ambiguities has typically been addressed via construction dedicated models with built-in probabilistic capabilities that are trained to predict uncertainty estimates as variables of interest. These approaches require distinct architectural components and training mechanisms, may include restrictive assumptions and exhibit overconfidence, i.e., high confidence in imprecise predictions. In this work, we propose a post-hoc sampling strategy for estimating predictive uncertainty accounting for data ambiguity. The method can generate different plausible outputs for a given input and does not assume parametric forms of predictive distributions. It is architecture agnostic and can be applied to any feed-forward deterministic network without changes to the architecture nor training procedure. Experiments on regression tasks on imaging and non-imaging input data show the method's ability to generate diverse and multi-modal predictive distributions and how estimated uncertainty correlates with prediction error.
更多查看译文
关键词
Uncertainty quantification,Deep learning,Metropolis-Hastings MCMC
AI 理解论文
溯源树
样例
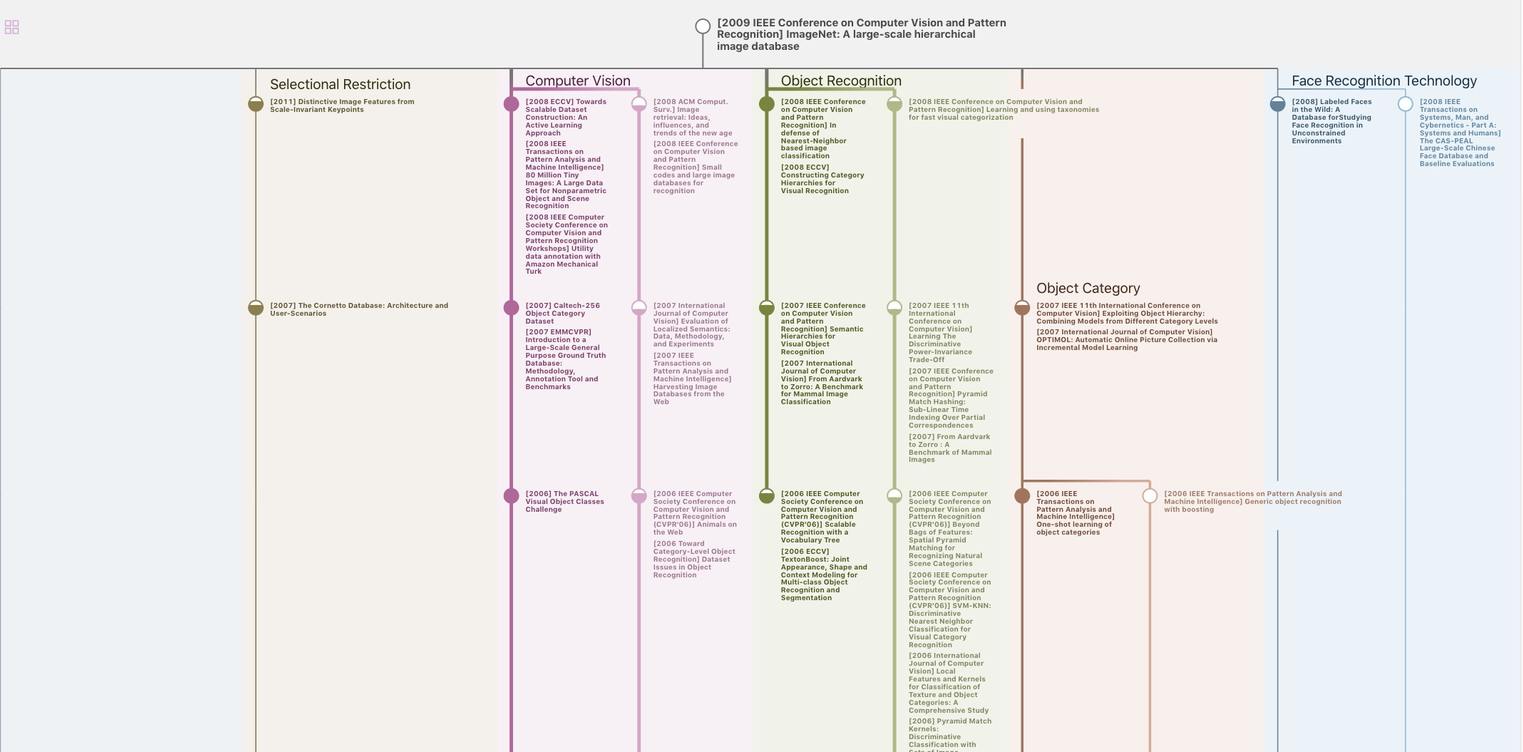
生成溯源树,研究论文发展脉络
Chat Paper
正在生成论文摘要