A Lightweight Specific Emitter Identification Model for IIoT Devices Based on Adaptive Broad Learning
IEEE Transactions on Industrial Informatics(2023)
摘要
Specific emitter identification (SEI) is a technology that extracts subtle features from signals sent by emitters to identify different individuals. It can effectively improve the security of the Industrial Internet of Things (IIoT) by acting on the physical layer of the internet. Recent research on SEI has focused on deep learning (DL) models that can automatically learn effective inherent emitter features from raw signals. Nevertheless, training popular DL models is computationally expensive because of the numerous hyperparameters and nonscalable structures. This limits the application of DL-based SEI models in certain practical IIoT scenarios. To address this concern, we propose an adaptive broad learning (ABL) method to build a lightweight SEI model. In the proposed model, the raw signal samples are mapped to feature nodes, and the emitters are denoted as the output nodes. The hidden nodes are directly connected to the output nodes by a broad network. Through this flat structure, the size and calculation amount of the model can be effectively reduced. To further economize the computational cost, we designed an adaptive node expansion strategy for rapidly obtaining the optimal hyperparameters of the models. The results of experiments on real-world data prove the superiority of ABL over popular state-of-the-art DL-based SEI models.
更多查看译文
关键词
Feature extraction,Adaptation models,Computational modeling,Industrial Internet of Things,Adaptive systems,Security,Training,Broad learning system (BLS),Industrial Internet of Things (IIoT),lightweight,specific emitter identification (SEI)
AI 理解论文
溯源树
样例
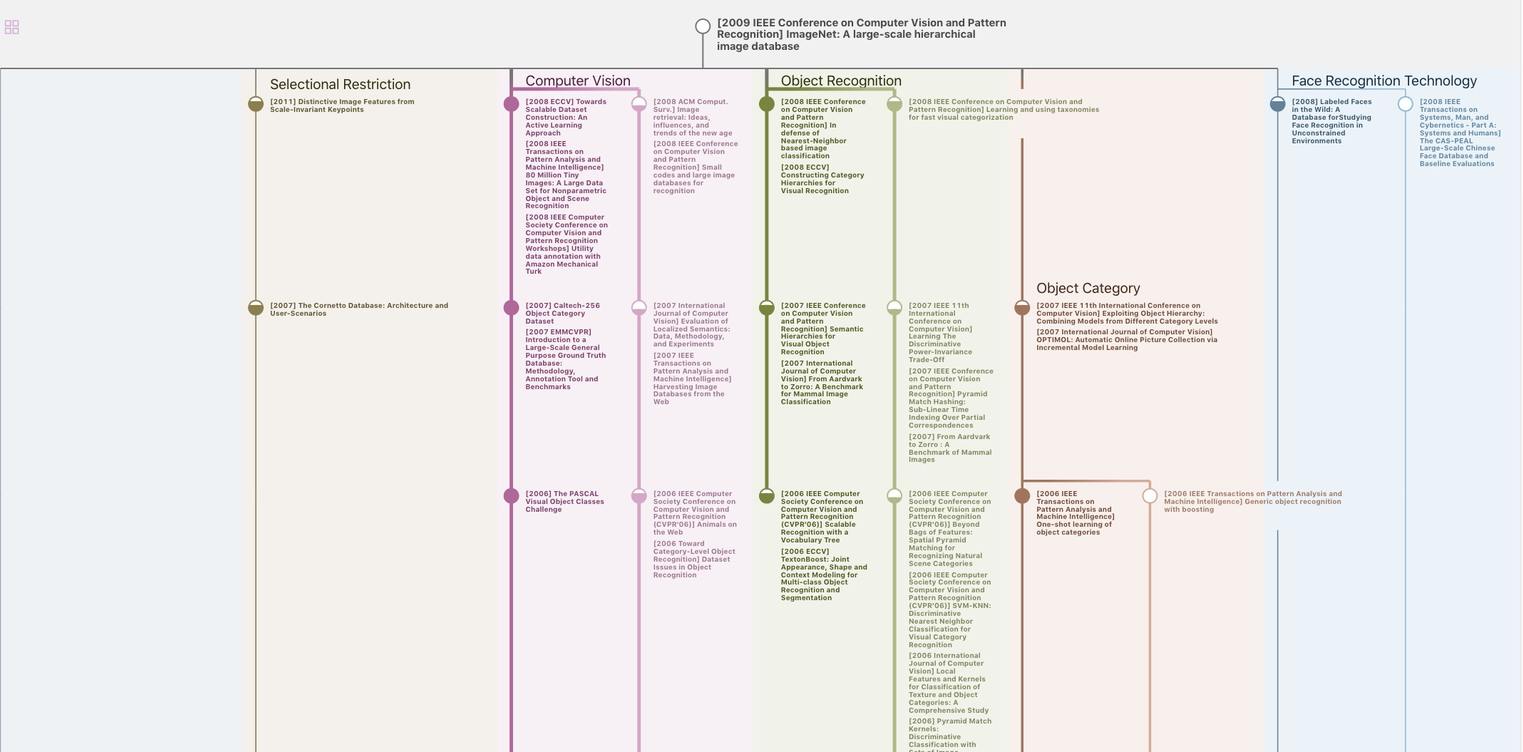
生成溯源树,研究论文发展脉络
Chat Paper
正在生成论文摘要