Estimating Long-term Effects from Experimental Data
Sixteenth ACM Conference on Recommender Systems(2022)
摘要
BSTRACT A/B testing is a powerful tool for a company to make informed decisions about their services and products. A limitation of A/B tests is that they do not easily extend to measure post-experiment (long-term) differences. In this talk, we study a different approach inspired by recent advances in off-policy evaluation in reinforcement learning (RL). The basic RL approach assumes customer behavior follows a stationary Markovian process, and estimates the average engagement metric when the process reaches the steady state. However, in realistic scenarios, the stationary assumption is often violated due to weekly variations and seasonality effects. To tackle this challenge, we propose a variation by relaxing the stationary assumption. We empirically tested both stationary and nonstationary approaches in a synthetic dataset and an online store dataset.
更多查看译文
AI 理解论文
溯源树
样例
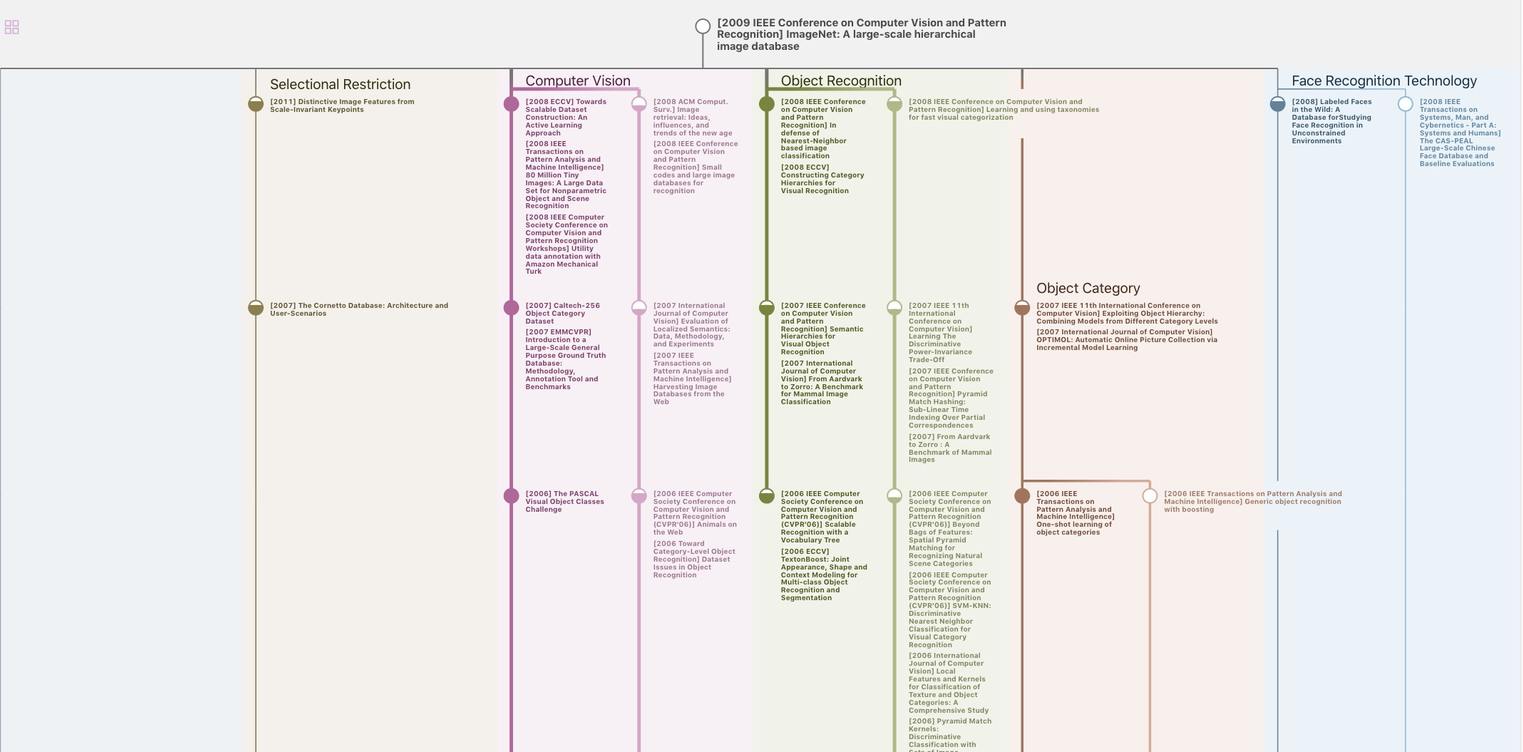
生成溯源树,研究论文发展脉络
Chat Paper
正在生成论文摘要