Graph Polynomial Convolution Models for Node Classification of Non-Homophilous Graphs
arxiv(2022)
摘要
We investigate efficient learning from higher-order graph convolution and learning directly from adjacency matrices for node classification. We revisit the scaled graph residual network and remove ReLU activation from residual layers and apply a single weight matrix at each residual layer. We show that the resulting model lead to new graph convolution models as a polynomial of the normalized adjacency matrix, the residual weight matrix, and the residual scaling parameter. Additionally, we propose adaptive learning between directly graph polynomial convolution models and learning directly from the adjacency matrix. Furthermore, we propose fully adaptive models to learn scaling parameters at each residual layer. We show that generalization bounds of proposed methods are bounded as a polynomial of eigenvalue spectrum, scaling parameters, and upper bounds of residual weights. By theoretical analysis, we argue that the proposed models can obtain improved generalization bounds by limiting the higher-orders of convolutions and direct learning from the adjacency matrix. Using a wide set of real-data, we demonstrate that the proposed methods obtain improved accuracy for node-classification of non-homophilous graphs.
更多查看译文
关键词
node classification,graph,models,non-homophilous
AI 理解论文
溯源树
样例
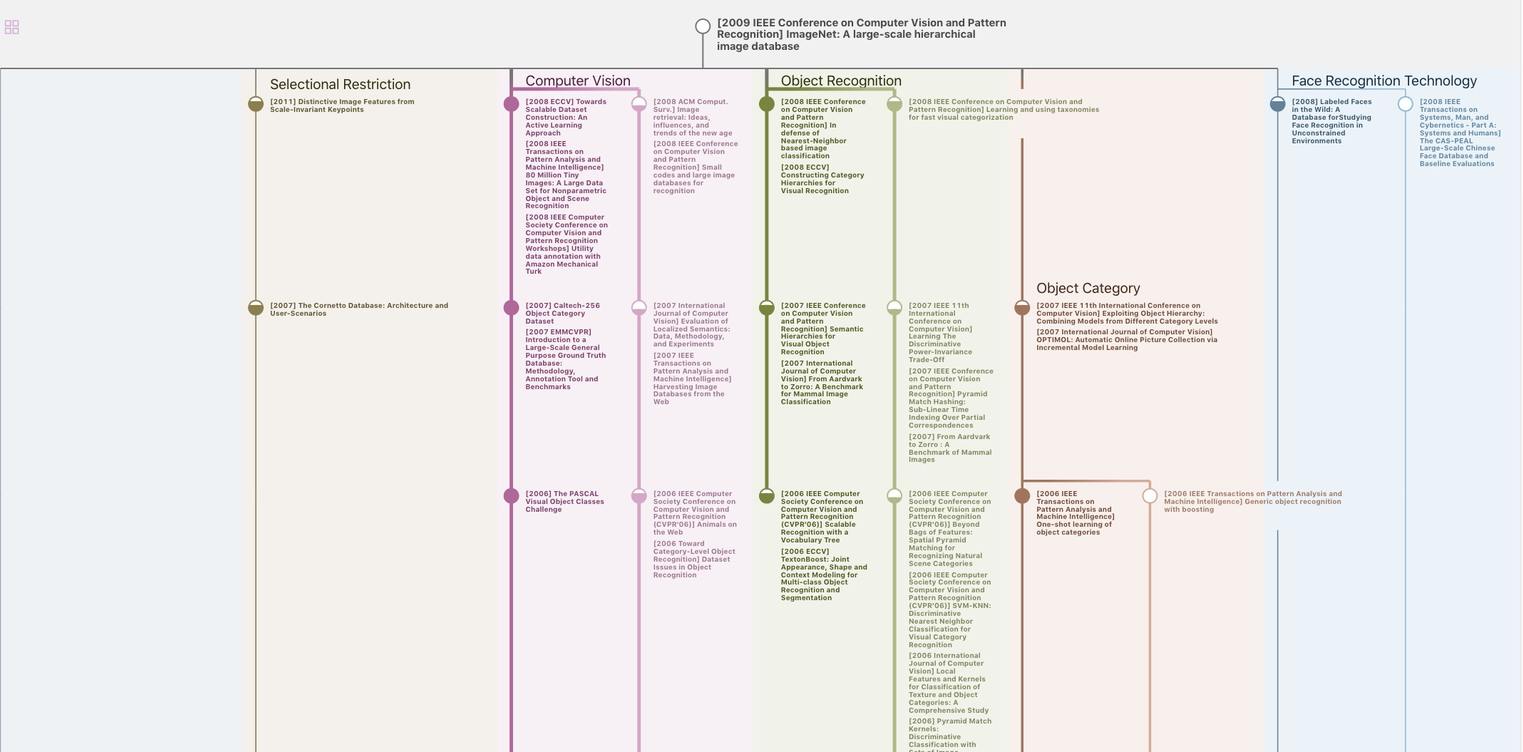
生成溯源树,研究论文发展脉络
Chat Paper
正在生成论文摘要