Non-autoregressive Error Correction for CTC-based ASR with Phone-conditioned Masked LM
Conference of the International Speech Communication Association (INTERSPEECH)(2022)
摘要
Connectionist temporal classification (CTC) -based models are attractive in automatic speech recognition (ASR) because of their non-autoregressive nature. To take advantage of text-only data, language model (LM) integration approaches such as rescoring and shallow fusion have been widely used for CTC. However, they lose CTC's non-autoregressive nature because of the need for beam search, which slows down the inference speed. In this study, we propose an error correction method with phone-conditioned masked LM (PC-MLM). In the proposed method, less confident word tokens in a greedy decoded output from CTC are masked. PC-MLM then predicts these masked word tokens given unmasked words and phones supplementally predicted from CTC. We further extend it to Deletable PC-MLM in order to address insertion errors. Since both CTC and PC-MLM are non-autoregressive models, the method enables fast LM integration. Experimental evaluations on the Corpus of Spontaneous Japanese (CSJ) and TED-LIUM2 in domain adaptation setting shows that our proposed method outperformed rescoring and shallow fusion in terms of inference speed, and also in terms of recognition accuracy on CSJ.
更多查看译文
关键词
asr,non-autoregressive,ctc-based,phone-conditioned
AI 理解论文
溯源树
样例
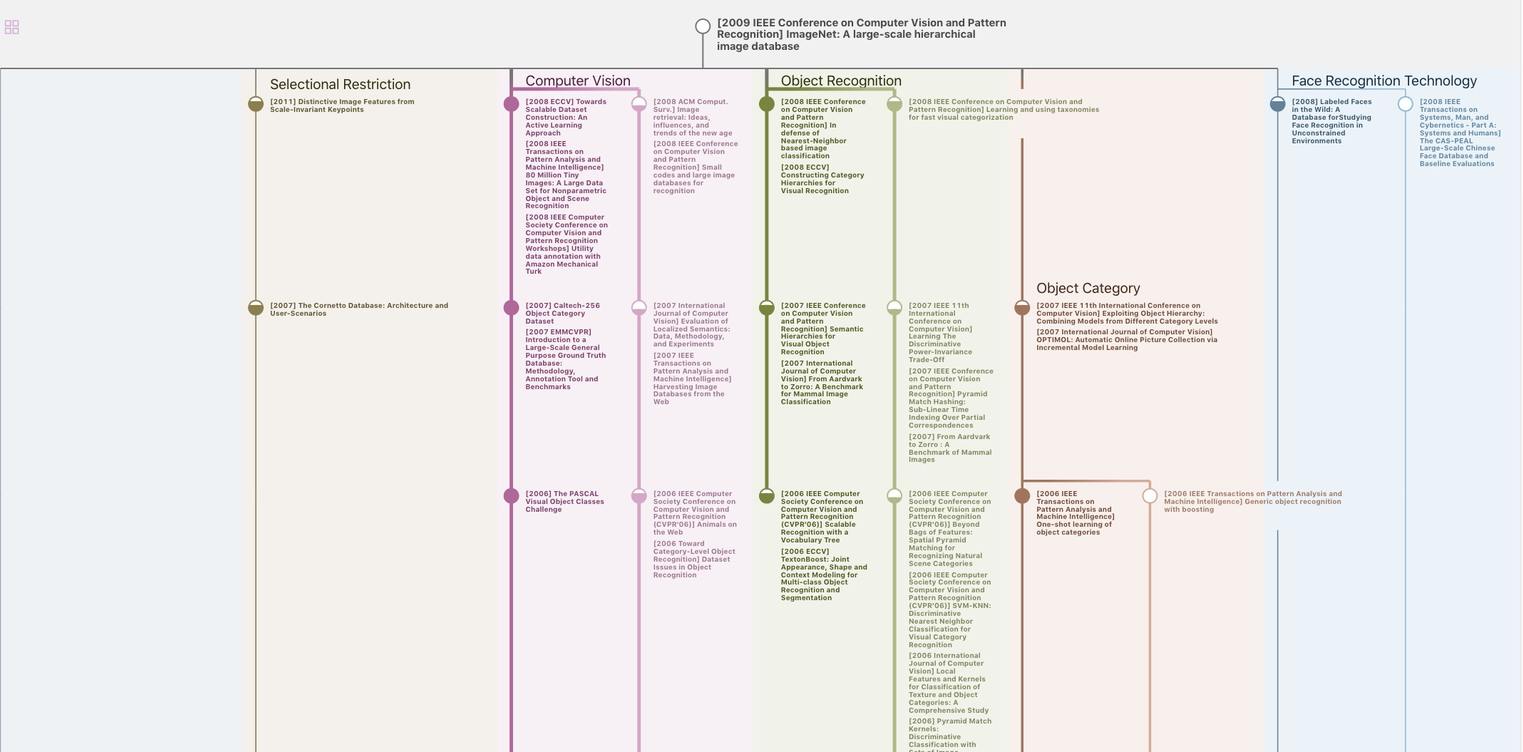
生成溯源树,研究论文发展脉络
Chat Paper
正在生成论文摘要