Class-distinctiveness-based frequency band selection on the Riemannian manifold for oscillatory activity-based BCIs: preliminary results.
Annual International Conference of the IEEE Engineering in Medicine and Biology Society (EMBC)(2022)
摘要
Considering user-specific settings is known to enhance Brain-Computer Interface (BCI) performances. In particular, the optimal frequency band for oscillatory activity classification is highly user-dependent and many frequency band selection methods have been developed in the past two decades. However, it is not well studied whether those conventional methods can be efficiently applied to the Riemannian BCIs, a recent family of BCI systems that utilize the non-Euclidean nature of the data unlike conventional BCI pipelines. In this paper, we proposed a novel frequency band selection method working on the Riemannian manifold. The frequency band is selected considering the class distinctiveness as quantified based on the inter-class distance and the intra-class variance on the manifold. An advantage of this method is that the frequency bandwidth can be adjusted for each individual without intensive optimization steps. In a comparative experiment using a public dataset of motor imagery-based BCI, our method showed a substantial improvement in average accuracy over both a fixed broad frequency band and a popular conventional frequency band selection method. In particular, our method substantially improved performances for subjects with initially low accuracies. This preliminary result suggests the importance of developing new user-specific setting algorithms considering the manifold properties, rather than directly applying methods developed prior to the rise of the Riemannian BCIs.
更多查看译文
关键词
frequency band selection,bcis,riemannian manifold,class-distinctiveness-based,activity-based
AI 理解论文
溯源树
样例
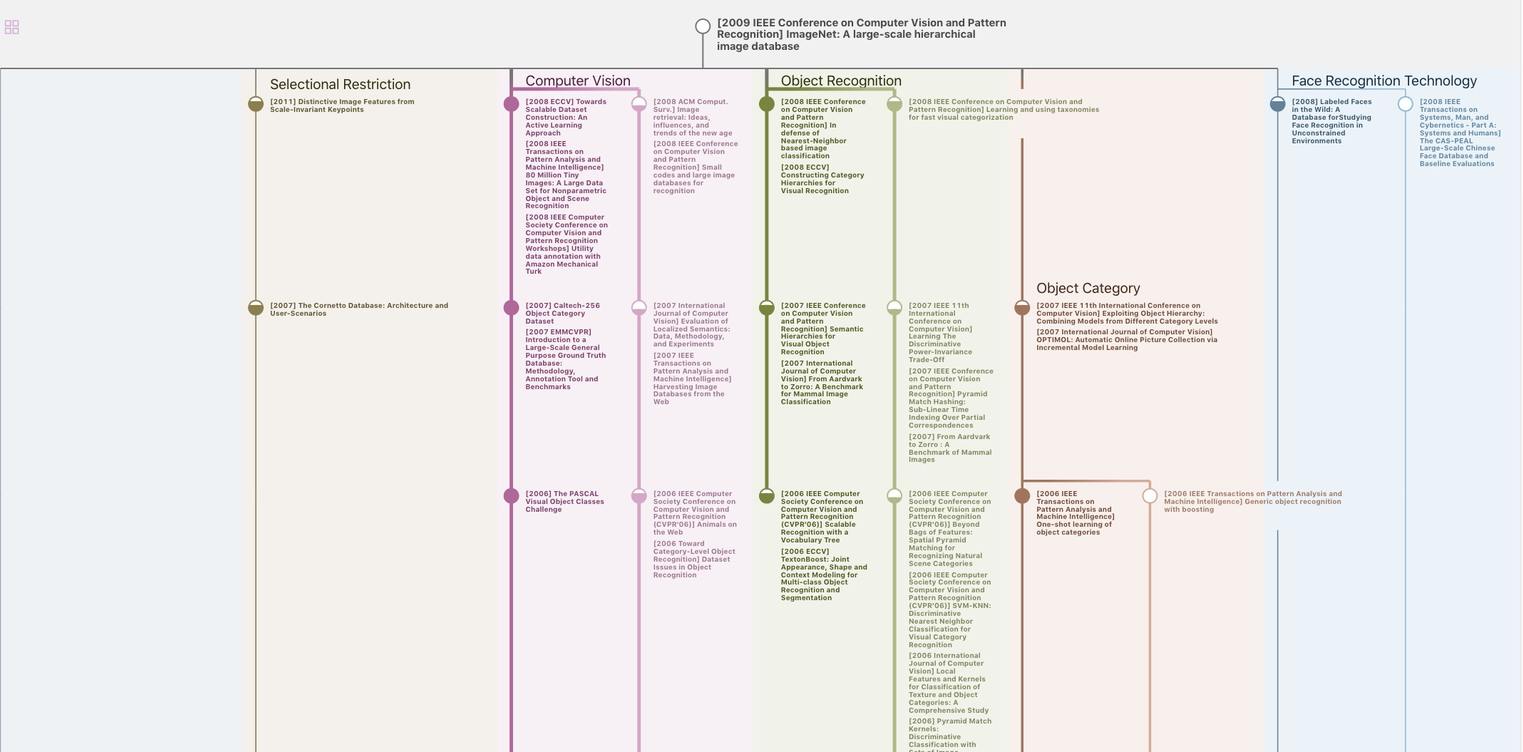
生成溯源树,研究论文发展脉络
Chat Paper
正在生成论文摘要