Acoustic scene analysis using analog spiking neural network
Neuromorphic Computing and Engineering(2022)
摘要
Abstract Sensor nodes in a wireless sensor network (WSN) for security surveillance applications should preferably be small, energy-efficient, and inexpensive with in-sensor computational abilities. An appropriate data processing scheme in the sensor node reduces the power dissipation of the transceiver through the compression of information to be communicated. This study attempted a simulation-based analysis of human footstep sound classification in natural surroundings using simple time-domain features. The spiking neural network (SNN), a computationally low-weight classifier derived from an artificial neural network (ANN), was used to classify acoustic sounds. The SNN and required feature extraction schemes are amenable to low-power subthreshold analog implementation. The results show that all analog implementations of the proposed SNN scheme achieve significant power savings over the digital implementation of the same computing scheme and other conventional digital architectures using frequency-domain feature extraction and ANN-based classification. The algorithm is tolerant of the impact of process variations, which are inevitable in analog design, owing to the approximate nature of the data processing involved in such applications. Although SNN provides low-power operation at the algorithm level, ANN to SNN conversion leads to an unavoidable loss of classification accuracy of ~5%. We exploited the low-power operation of the analog processing SNN module by applying redundancy and majority voting, which improved the classification accuracy, taking it close to the ANN model.
更多查看译文
关键词
acoustic wireless sensor nodes, security surveillance application, spiking neural network, resistive crossbar network, energy efficiency
AI 理解论文
溯源树
样例
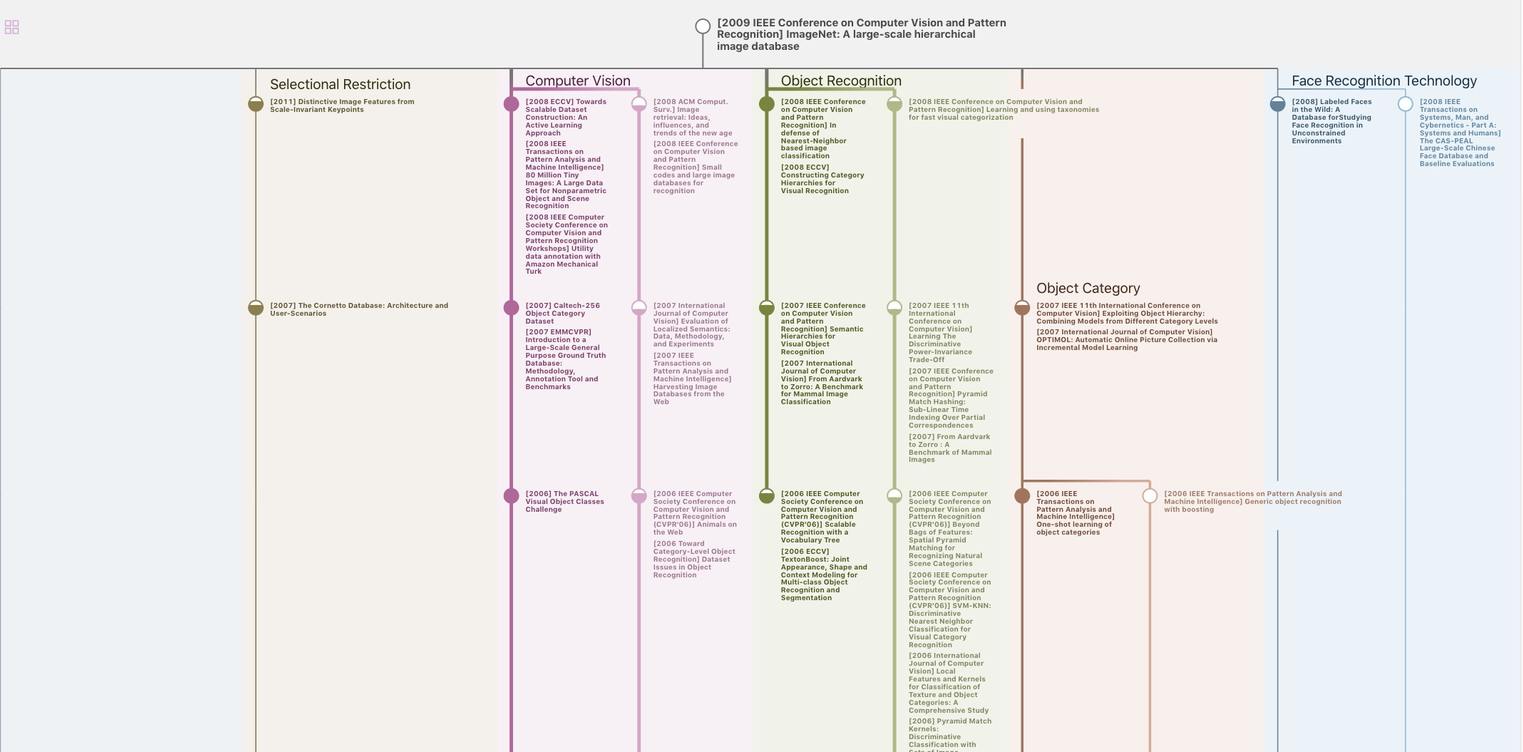
生成溯源树,研究论文发展脉络
Chat Paper
正在生成论文摘要