National Land Use Regression Model for NO 2 Using Street View Imagery and Satellite Observations.
Environmental science & technology(2022)
摘要
Land use regression (LUR) models are widely applied to estimate intra-urban air pollution concentrations. National-scale LURs typically employ predictors from multiple curated geodatabases at neighborhood scales. In this study, we instead developed national NO models relying on innovative street-level predictors extracted from Google Street View [GSV] imagery. Using machine learning (random forest), we developed two types of models: (1) GSV-only models, which use only GSV features, and (2) GSV + OMI models, which also include satellite observations of NO. Our results suggest that street view imagery alone may provide sufficient information to explain NO variation. Satellite observations can improve model performance, but the contribution decreases as more images are available. Random 10-fold cross-validation of our best models were 0.88 (GSV-only) and 0.91 (GSV + OMI)─a performance that is comparable to traditional LUR approaches. Importantly, our models show that street-level features might have the potential to better capture intra-urban variation of NO pollution than traditional LUR. Collectively, our findings indicate that street view image-based modeling has great potential for building large-scale air quality models under a unified framework. Toward that goal, we describe a cost-effective image sampling strategy for future studies based on a systematic evaluation of image availability and model performance.
更多查看译文
关键词
air quality,computer vision,empirical models,exposure assessment,image sampling and processing,machine learning
AI 理解论文
溯源树
样例
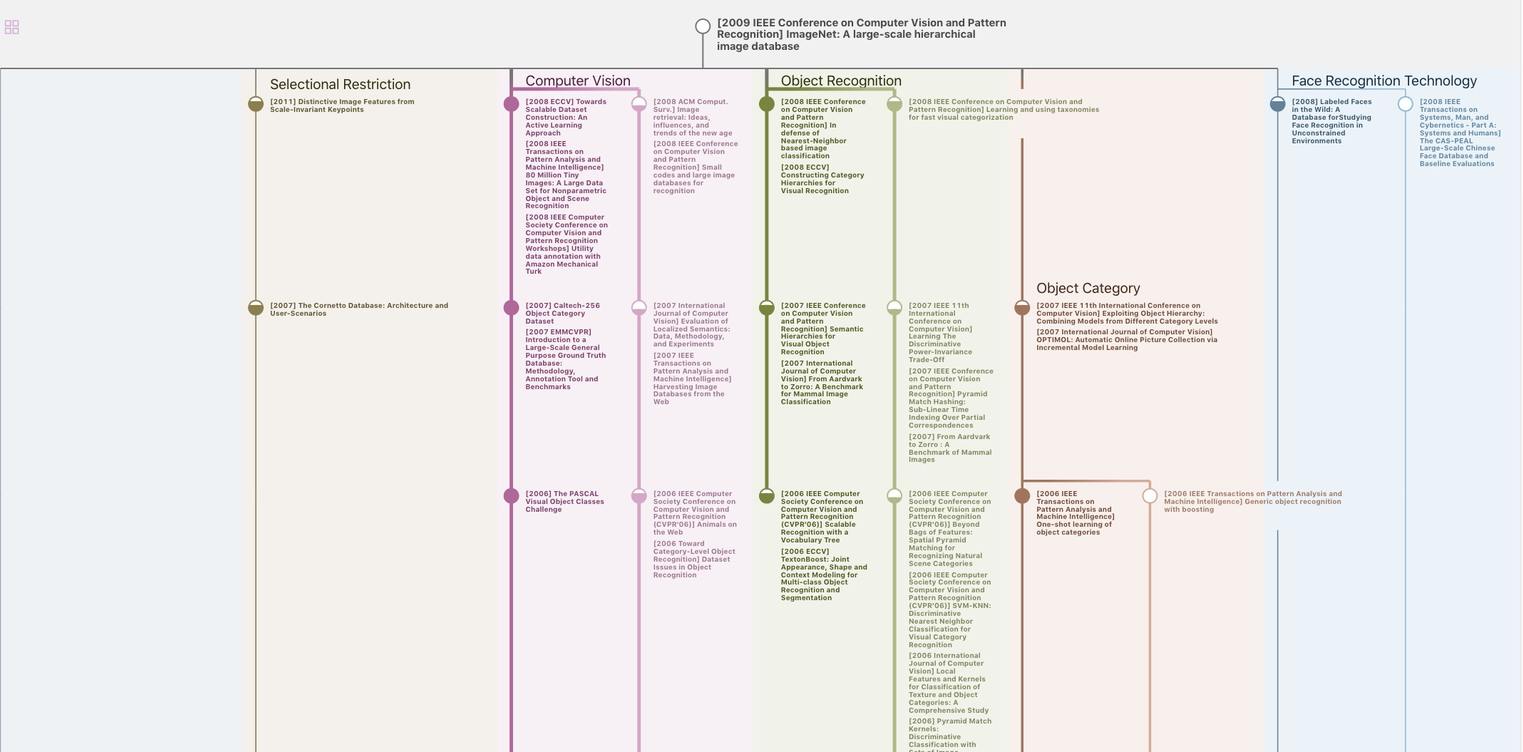
生成溯源树,研究论文发展脉络
Chat Paper
正在生成论文摘要