Joint Event Relation Identification Based on Multiscale Convolutional Neural Network and Sharing Strategy
Wireless Communications and Mobile Computing(2022)
摘要
At present, most of the event relation identification work mainly focuses on the sequential temporal and explicit causal relation between events. These methods usually ignore the role of synchronous temporal and implicit causal relation in sentences, which makes the semantic understanding of the model deviate from the text. In this paper, we propose a joint event relation identification model. The model uses bidirectional GRU and multiscale convolutional neural network to obtain the context semantic features and multiscale local semantic features of text, respectively. Then, these two kinds of features are fused to fully obtain the semantics of the text itself. In addition, we build encoders and decoders of event temporal and causal relation, respectively, to obtain the event temporal and causal semantic features from text. In this process, considering the correlation between event timing and causality, we use three different parameter sharing strategies to realize the interaction between event temporal and causal semantic features. The experimental results on the legal field dataset we constructed show that our model has made significant improvements compared with the baseline model. Through experimental analysis, our method can effectively improve the identification performance of synchronous temporal and implicit causality relation.
更多查看译文
关键词
multiscale convolutional neural network,event,relation,neural network,sharing strategy
AI 理解论文
溯源树
样例
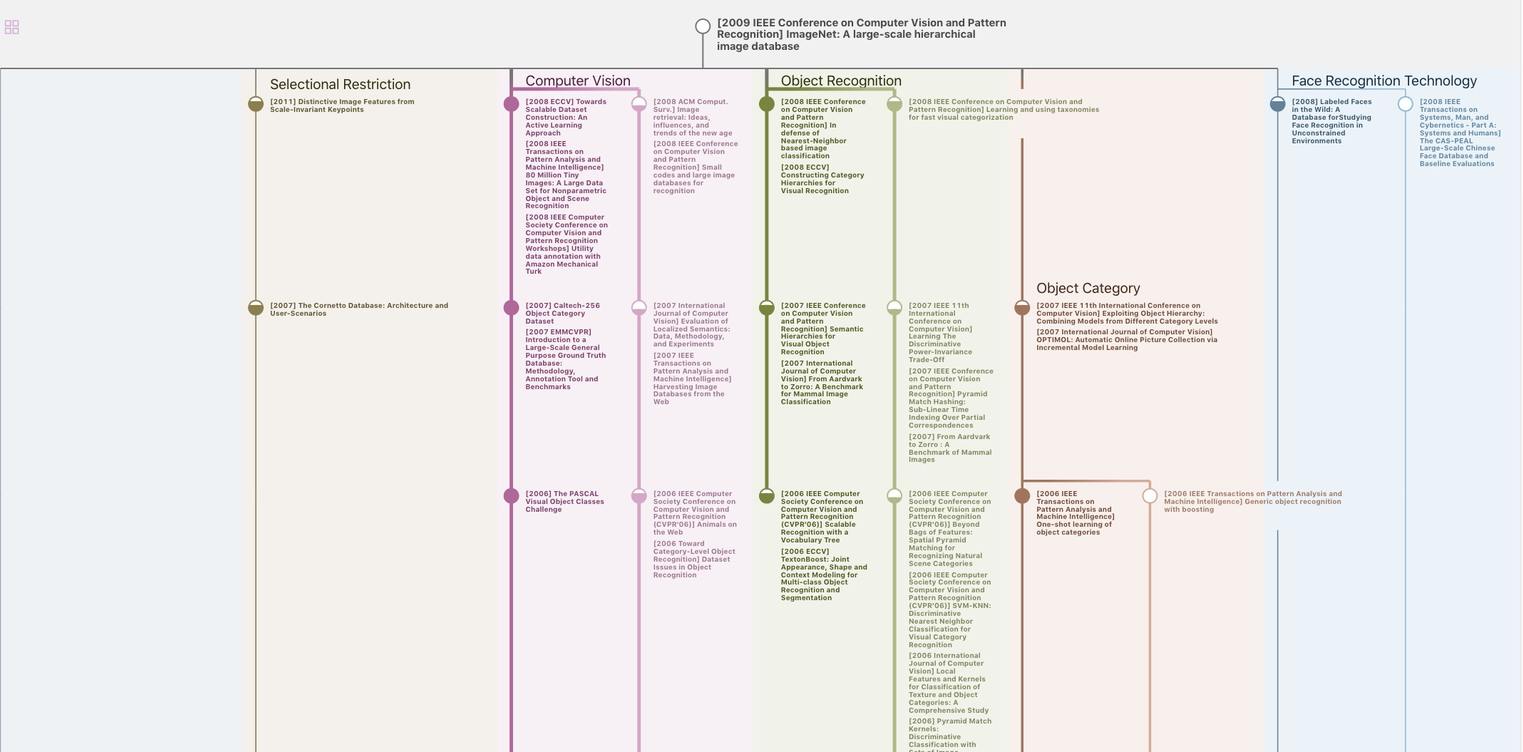
生成溯源树,研究论文发展脉络
Chat Paper
正在生成论文摘要