A 1ynm 1.25V 8Gb 16Gb/s/Pin GDDR6-Based Accelerator-in-Memory Supporting 1TFLOPS MAC Operation and Various Activation Functions for Deep Learning Application
IEEE Journal of Solid-State Circuits(2023)
摘要
In this article, a 1.25-V 8-Gb, 16-Gb/s/pin GDDR6-based accelerator-in-memory (AiM) is presented. A dedicated command (CMD) set for deep learning (DL) is introduced to minimize latency when switching operation modes, and a bank-wide mantissa shift (BWMS) scheme is adopted to minimize calculation delay time, current consumption, and circuit area during multiply-accumulate (MAC) operation. By storing the lookup table (LUT) in the reserved word line in the dynamic random access memory (DRAM) bank cell, it is possible to support various activation functions (AFs), such as Gaussian error linear unit (GELU), sigmoid, and Tanh as well as rectified linear unit (ReLU) and Leaky ReLU. Performance evaluation was conducted by measuring the fabricated chip in ATE and a self-manufactured field-programmable gate array (FPGA)-based system. In the ATE-level evaluation, it operates at 16 Gbps up to a voltage as low as 1.10 V. When evaluated by GEMV and MNIST in the FPGA-based system, it was confirmed that the performance gains of 7.5–10.5 times were possible compared to the HBM2-based or GDDR6-based systems.
更多查看译文
关键词
Accelerator-in-memory (AiM),activation function (AF),adder tree,bank-wide mantissa shift (BWMS),dynamic random access memory (DRAM),GDDR6,multiply-accumulate (MAC),processing-in-memory (PIM)
AI 理解论文
溯源树
样例
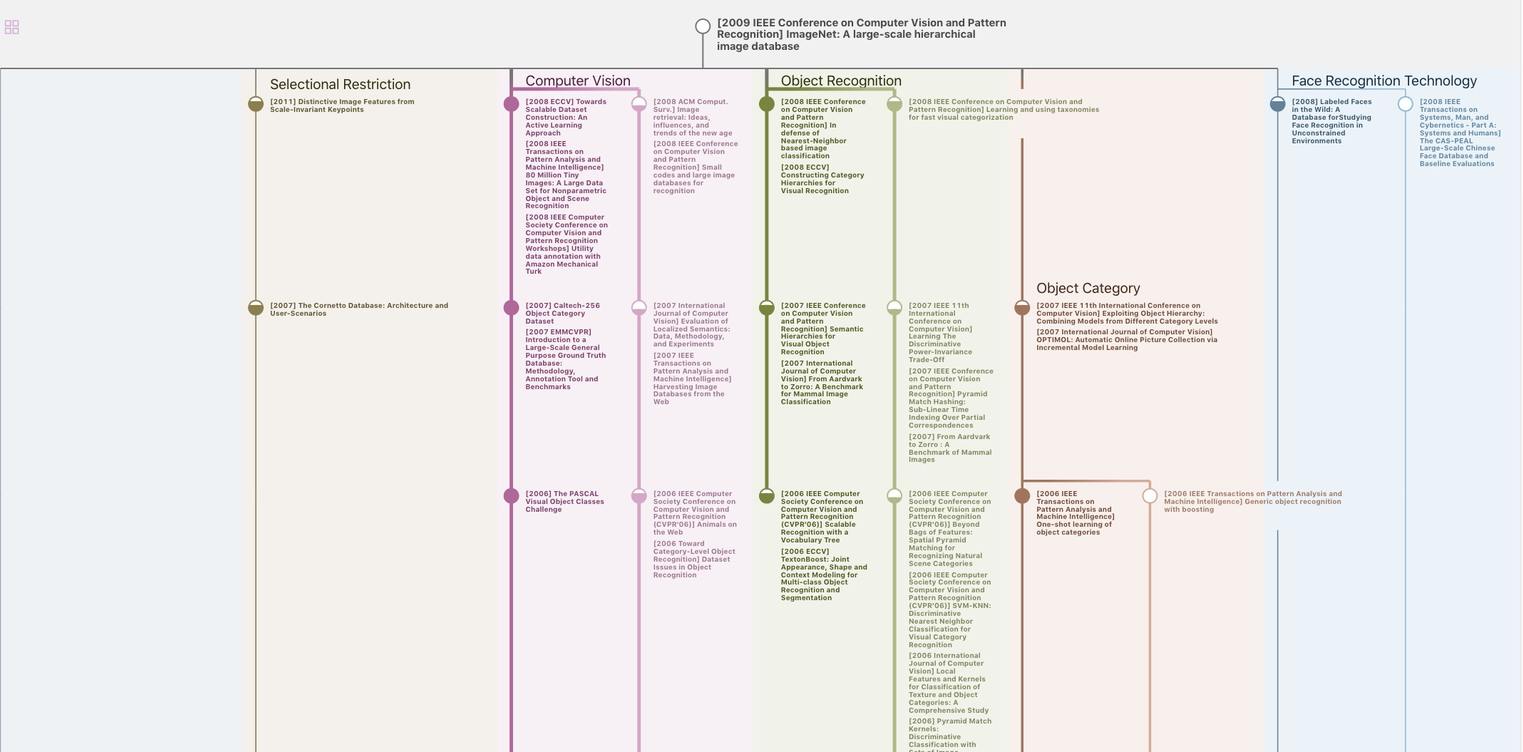
生成溯源树,研究论文发展脉络
Chat Paper
正在生成论文摘要