Identifying patterns in amyotrophic lateral sclerosis progression from sparse longitudinal data
Nature Computational Science(2022)
摘要
The clinical presentation of amyotrophic lateral sclerosis (ALS), a fatal neurodegenerative disease, varies widely across patients, making it challenging to determine if potential therapeutics slow progression. We sought to determine whether there were common patterns of disease progression that could aid in the design and analysis of clinical trials. We developed an approach based on a mixture of Gaussian processes to identify clusters of patients sharing similar disease progression patterns, modeling their average trajectories and the variability in each cluster. We show that ALS progression is frequently nonlinear, with periods of stable disease preceded or followed by rapid decline. We also show that our approach can be extended to Alzheimer’s and Parkinson’s diseases. Our results advance the characterization of disease progression of ALS and provide a flexible modeling approach that can be applied to other progressive diseases. A machine learning approach is presented to identify dominant patterns in disease progression in amyotrophic lateral sclerosis (ALS), Alzheimer’s disease and Parkinson’s disease. The nonlinearity of ALS progression has important clinical implications.
更多查看译文
关键词
Amyotrophic lateral sclerosis,Computational models,Translational research,Computer Science,general
AI 理解论文
溯源树
样例
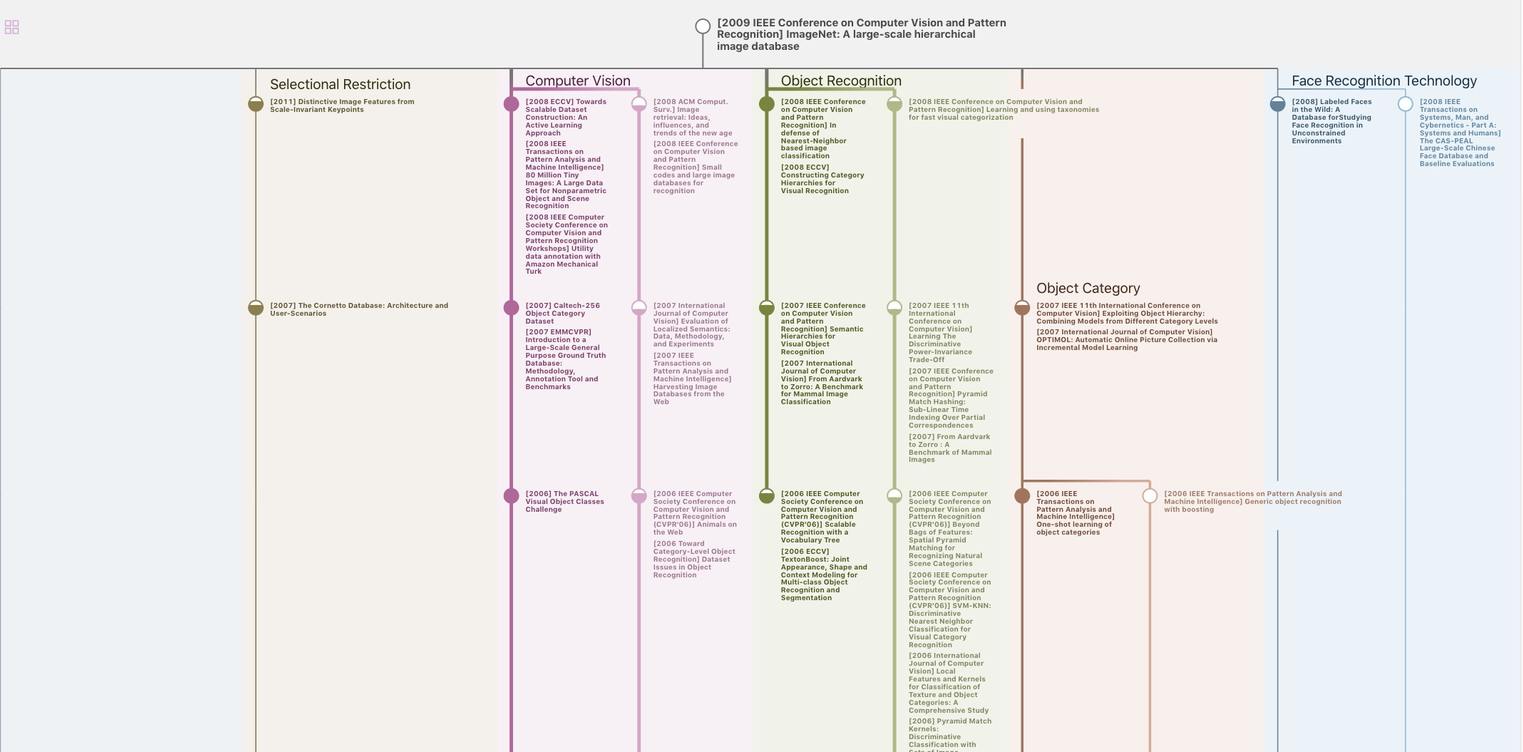
生成溯源树,研究论文发展脉络
Chat Paper
正在生成论文摘要