Fusing Hyperspectral and Multispectral Images via Low-Rank Hankel Tensor Representation
REMOTE SENSING(2022)
摘要
Hyperspectral images (HSIs) have high spectral resolution and low spatial resolution. HSI super-resolution (SR) can enhance the spatial information of the scene. Current SR methods have generally focused on the direct utilization of image structure priors, which are often modeled in global or local lower-order image space. The spatial and spectral hidden priors, which are accessible from higher-order space, cannot be taken advantage of when using these methods. To solve this problem, we propose a higher-order Hankel space-based hyperspectral image-multispectral image (HSI-MSI) fusion method in this paper. In this method, the higher-order tensor represented in the Hankel space increases the HSI data redundancy, and the hidden relationships are revealed by the nonconvex penalized Kronecker-basis-representation-based tensor sparsity measure (KBR). Weighted 3D total variation (W3DTV) is further applied to maintain the local smoothness in the image structure, and an efficient algorithm is derived under the alternating direction method of multipliers (ADMM) framework. Extensive experiments on three commonly used public HSI datasets validate the superiority of the proposed method compared with current state-of-the-art SR approaches in image detail reconstruction and spectral information restoration.
更多查看译文
关键词
hyperspectral super-resolution,Hankel space,Kronecker-basis-representation tensor factorization,image fusion
AI 理解论文
溯源树
样例
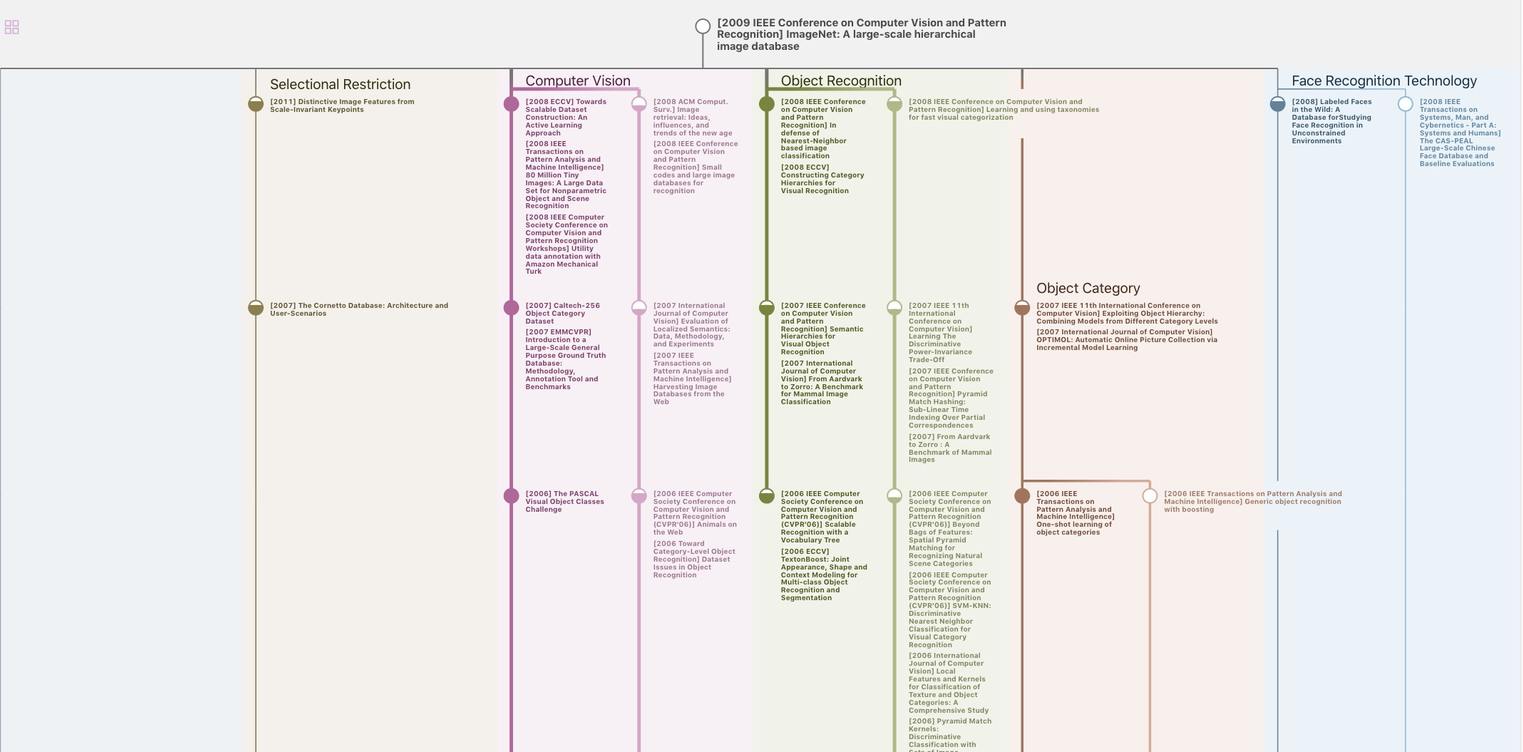
生成溯源树,研究论文发展脉络
Chat Paper
正在生成论文摘要