Concept-modulated model-based offline reinforcement learning for rapid generalization
arxiv(2022)
摘要
The robustness of any machine learning solution is fundamentally bound by the data it was trained on. One way to generalize beyond the original training is through human-informed augmentation of the original dataset; however, it is impossible to specify all possible failure cases that can occur during deployment. To address this limitation we combine model-based reinforcement learning and model-interpretability methods to propose a solution that self-generates simulated scenarios constrained by environmental concepts and dynamics learned in an unsupervised manner. In particular, an internal model of the agent's environment is conditioned on low-dimensional concept representations of the input space that are sensitive to the agent's actions. We demonstrate this method within a standard realistic driving simulator in a simple point-to-point navigation task, where we show dramatic improvements in one-shot generalization to different instances of specified failure cases as well as zero-shot generalization to similar variations compared to model-based and model-free approaches.
更多查看译文
关键词
offline reinforcement learning,reinforcement learning,generalization,concept-modulated,model-based
AI 理解论文
溯源树
样例
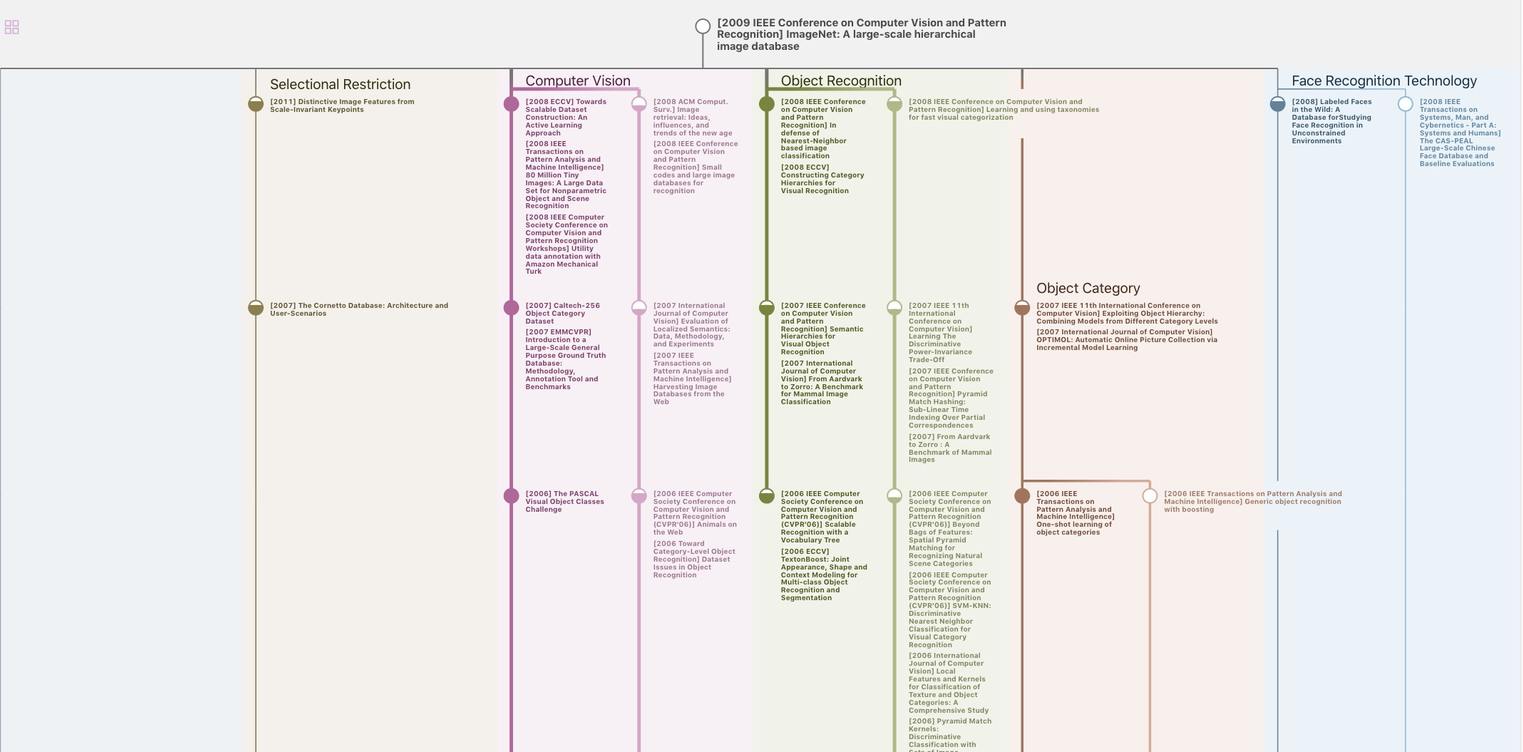
生成溯源树,研究论文发展脉络
Chat Paper
正在生成论文摘要