Incomplete Multiview Nonnegative Representation Learning With Graph Completion and Adaptive Neighbors
IEEE TRANSACTIONS ON NEURAL NETWORKS AND LEARNING SYSTEMS(2024)
摘要
Despite incomplete multiview clustering (IMC) being widely studied in the past decade, it is still difficult to model the correlation among multiple views due to the absence of partial views. Most existing works for IMC only mine the correlation among multiple views from available views and ignore the importance of missing views. To address this issue, we propose a novel Incomplete Multiview Nonnegative representation learning model with Graph completion and Adaptive neighbors (IMNGA), which performs common graph learning, missing graph completion, and consensus nonnegative representation learning simultaneously. In IMNGA, the common graph on all views and the incomplete graph of each view are used to reconstruct the completed graph of the corresponding view, where the common graph satisfies the neighbor constraints of incomplete multiview data and consensus representation. IMNGA gets consensus representation by factorizing completed and incomplete graphs, where consensus representation satisfies the common graph constraint. IMNGA shows its effectiveness by outperforming other state-of-the-art methods.
更多查看译文
关键词
Representation learning,Adaptation models,Task analysis,Sun,Learning systems,Laplace equations,Correlation,Clustering,consensus representation learning,graph completion,multiview learning,nonnegative matrix factorization
AI 理解论文
溯源树
样例
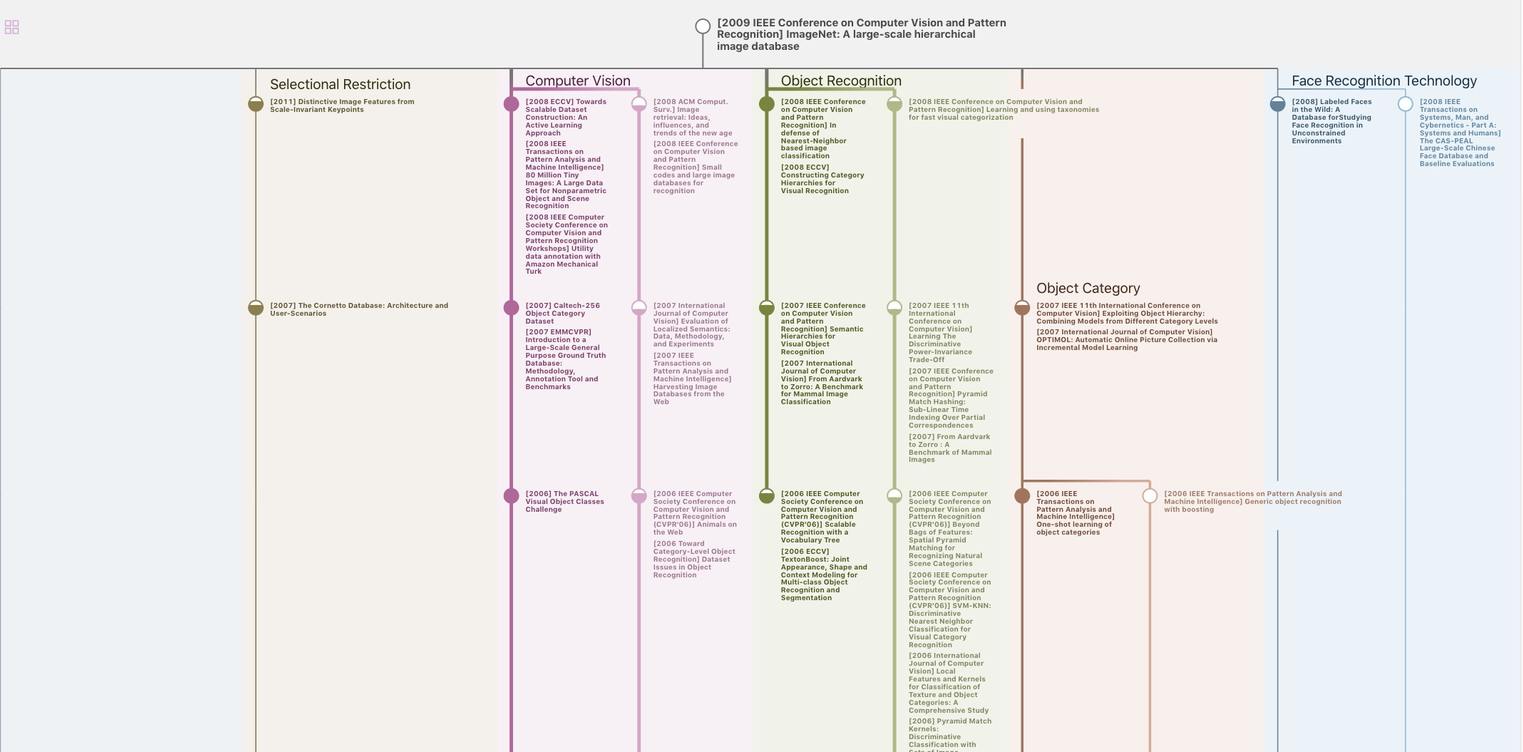
生成溯源树,研究论文发展脉络
Chat Paper
正在生成论文摘要