Estimating Demand for Online Delivery using Limited Historical Observations
arxiv(2022)
摘要
Driven in part by the COVID-19 pandemic, the pace of online purchases for at-home delivery has accelerated significantly. However, responding to this development has been challenging given the lack of public data. The existing data may be infrequent, and a significant portion of data may be missing because of survey participant non-responses. This data paucity renders conventional predictive models unreliable. We address this shortcoming by developing algorithms for data imputation and synthetic demand estimation for future years without the actual ground truth data. We use 2017 Puget Sound Regional Council (PSRC) and National Household Travel Survey (NHTS) data and impute from the NHTS for the Seattle-Tacoma-Bellevue MSA where delivery data is relatively more frequent. Our imputation has the mean-squared error $\mathsf{MSE} \approx 0.65$ to NHTS with mean $\approx 1$ and standard deviation $\approx 3.5$ and provides a similarity matching between the two data sources' samples. Given the unavailability of NHTS data for 2021, we use the temporal fidelity of PSRC data sources (2017 and 2021) to project the resolution onto the NHTS providing a synthetic estimate of NHTS deliveries. Beyond the improved reliability of the estimates, we report explanatory variables that were relevant in determining the volume of deliveries. This work furthers existing methods in demand estimation for goods deliveries by maximizing available sparse data to generate reasonable estimates that could facilitate policy decisions.
更多查看译文
关键词
online delivery,demand,observations
AI 理解论文
溯源树
样例
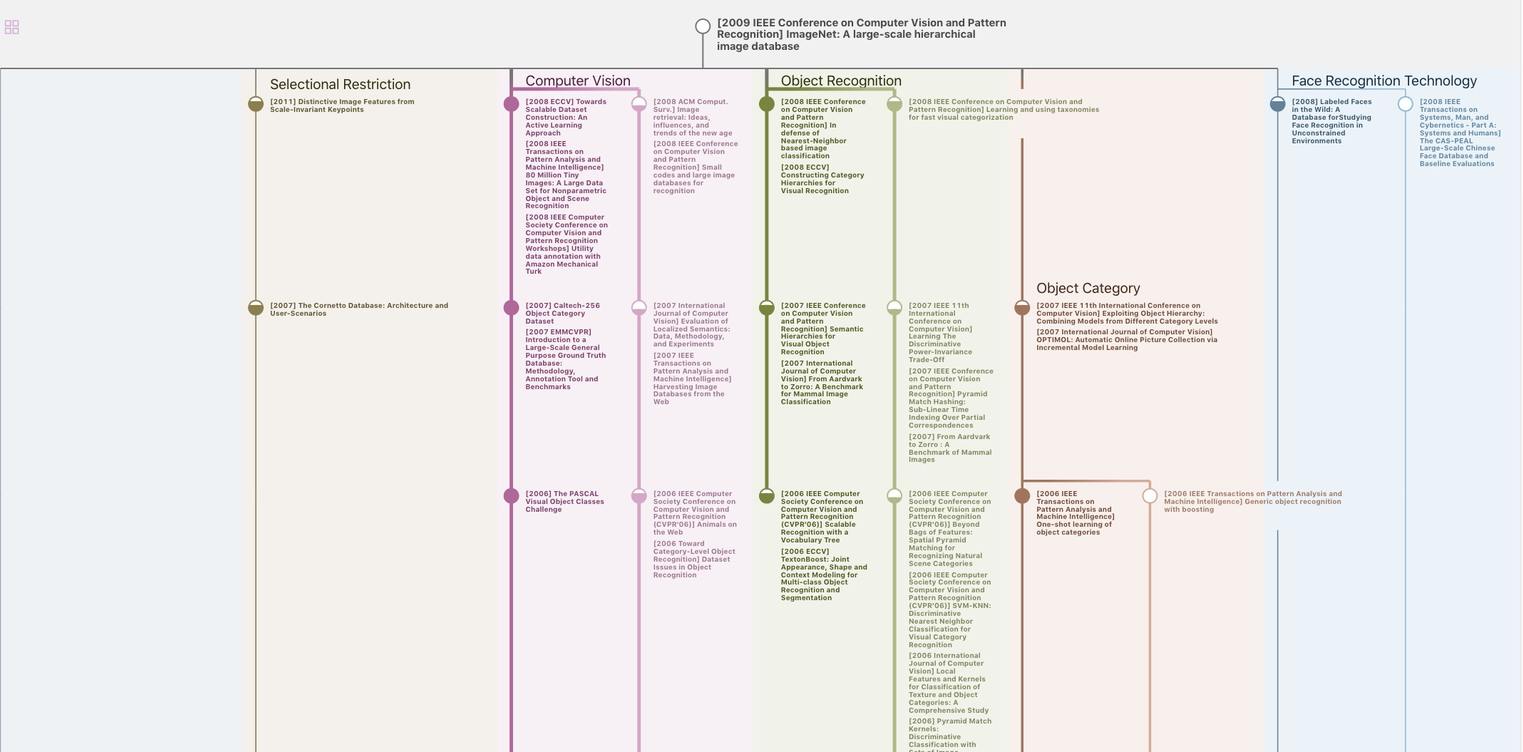
生成溯源树,研究论文发展脉络
Chat Paper
正在生成论文摘要