A Universal Self-Correcting Approach for Abnormal Jump Errors in Absolute Phase Retrieval
IEEE TRANSACTIONS ON INSTRUMENTATION AND MEASUREMENT(2022)
摘要
Codeword-assisted temporal phase unwrapping (TPU) is extensively applied in absolute phase retrieval, in which the fringe order embedded in the temporal sequence identifies the interval of the wrapped phase. However, owing to inevitable image blurring and random noise, the fringe order cannot be aligned with the interval of the wrapped phase at the pixel level and tends to fail easily under high-frequency measurements, thereby yielding abnormal jump errors (JEs). The existing approaches suffer from the limitations of universality, efficiency, and fringe frequency. To this end, this work proposes a universal self-correcting approach to remove abnormal JEs efficiently and accurately. Specifically, the virtual fringe order is introduced by analyzing the intensity changes in the phase-shifted patterns, and then it is combined with the real fringe order to form a complementary fringe order pair. In addition, to enhance the anti-interference ability of the virtual fringe order, the fringe order histogram check technique (FOHCT) is proposed to eliminate fringe order errors. The proposed approach can efficiently conduct various codeword-assisted absolute phase retrieval without introducing additional patterns. Furthermore, benefitting from no error accumulation, our approach is applicable for high-frequency measurements, thereby improving the measurement capability. The measurement results validated the universality and efficiency of the proposed approach. High-frequency measurements demonstrated that the proposed approach outperformed the median filtering and the phase coding approach in terms of error rate (0.009% versus 3.13% versus 1.76%).
更多查看译文
关键词
Encoding,Phase measurement,Measurement uncertainty,Pollution measurement,Histograms,Shape measurement,Cameras,Absolute phase retrieval,histogram check technique,jump errors (JEs),universal self-correcting approach
AI 理解论文
溯源树
样例
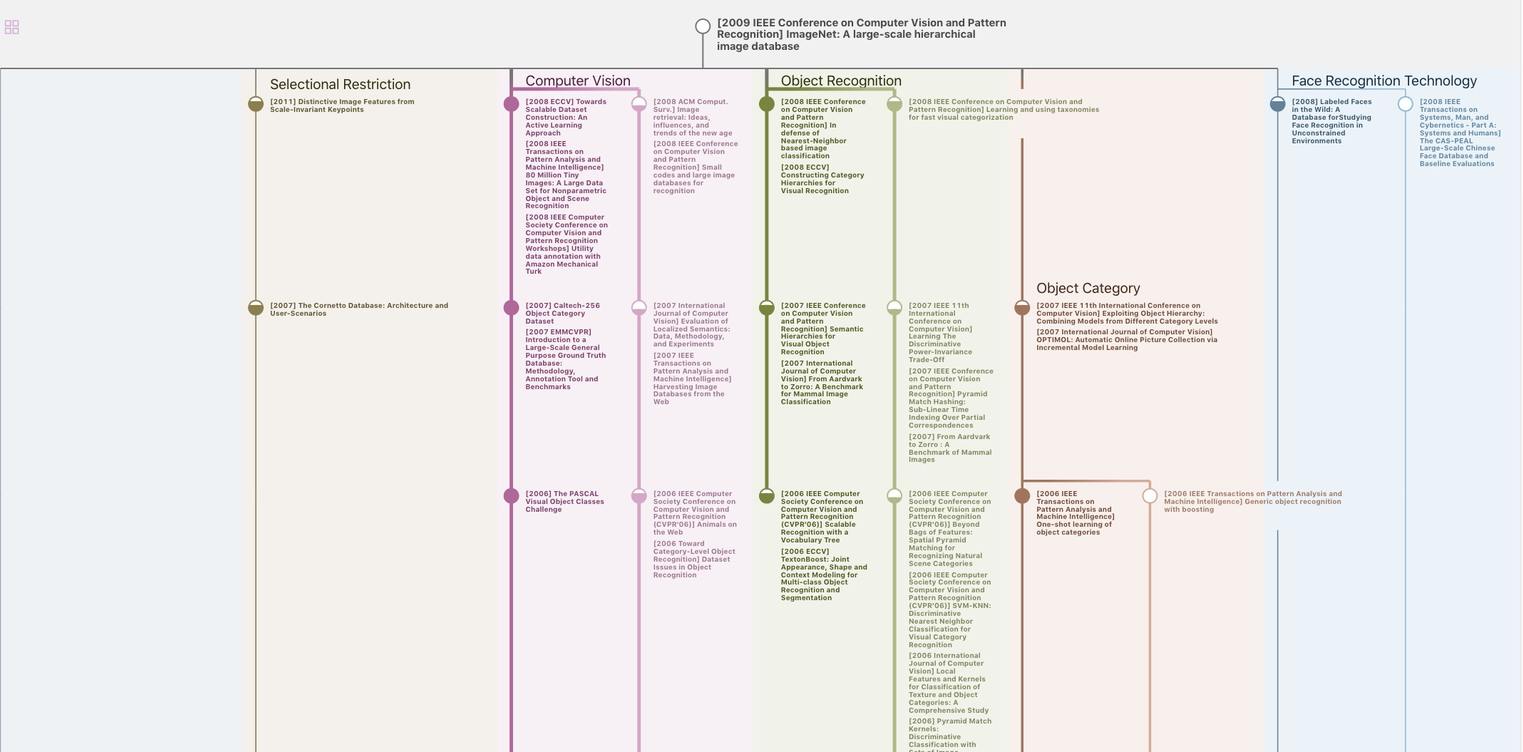
生成溯源树,研究论文发展脉络
Chat Paper
正在生成论文摘要