Collision Warning Algorithm Based on Efficient Federated Learning in Mobile Edge Computing Assisted Intelligent Driving
Journal of Electronics & Information Technology(2023)
摘要
Collision avoidance tasks in intelligent driving have challenges such as extremely high latency requirements and privacy protection. First, a Gated Recurrent Unit_Support Vector Machine (GRU_SVM) collision multi-level warning algorithm based on Semi-asynchronous Federated Learning with Adaptive Adjustment of Parameters (SFLAAP) is proposed. SFLAAP can dynamically adjust two training parameters according to training and resource conditions: the number of local training times and the number of local models participating in aggregation. Then, in order to solve the efficiency problem of collaborative training of collision warning model under resource-constrained Mobile Edge Computing (MEC), according to the relationship between the above parameters and SFLAAP training delay, a model for minimizing the total training delay is established, and it is transformed into a Markov Decision Process (MDP). Finally, in the established MDP, the Asynchronous Advantage Actor-Critic (A3C) algorithm is employed to determine adaptively the optimal training parameters, thereby reducing the training completion time of the collision warning model. The simulation results show that the proposed algorithm can effectively reduce the total training delay and ensure prediction accuracy.
更多查看译文
关键词
Collision warning,Federated Learning(FL),Mobile Edge Computing(MEC),Asynchronous Advantage Actor-Critic(A3C)
AI 理解论文
溯源树
样例
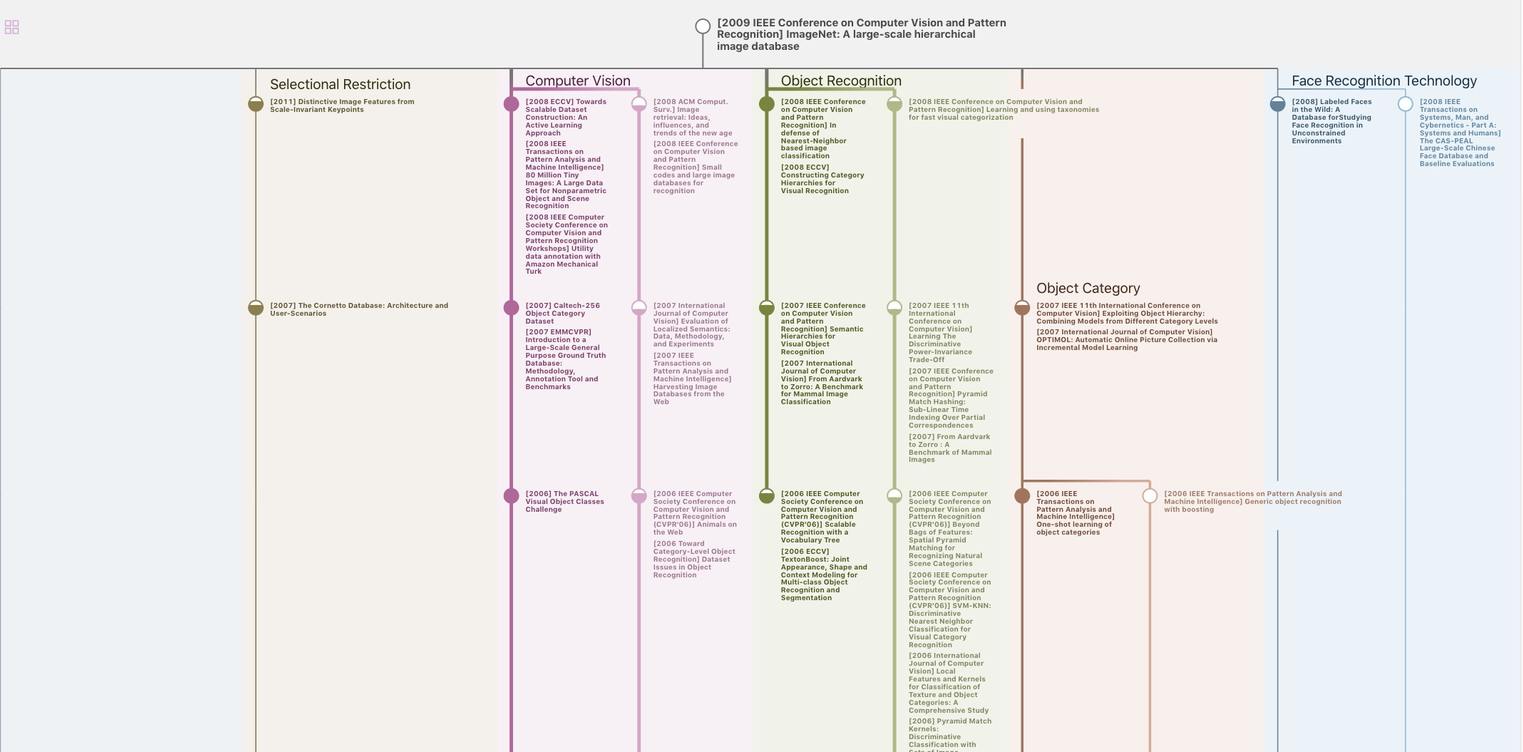
生成溯源树,研究论文发展脉络
Chat Paper
正在生成论文摘要