Privacy-Preserving Outsourced Logistic Regression on Encrypted Data from Homomorphic Encryption
SECURITY AND COMMUNICATION NETWORKS(2022)
摘要
Logistic regression is a data statistical technique, which is used to predict the probability that an event occurs. For some scenarios where the storage capabilities and computing resources of the data owner are limited, the data owner wants to train the logistic regression model on the cloud service provider, while the high sensitivity of training data requires effective privacy protection methods that enable efficient model training without exposing information about the training data to untrusted cloud service providers. Recently, several works have used cryptographic techniques to implement privacy-preserving logistic regression in such application scenarios. However, on large-scale training datasets, the existing works still have the problems of long model training time and poor model performance. To solve these problems, based on the homomorphic encryption (HE), we propose an efficient privacy-preserving outsourced logistic regression ((POLR)-O-2) on encrypted training data, which enables data owners to utilize the powerful storage and computing resources of cloud service providers for logistic regression analysis without exposing data privacy. Furthermore, the proposed scheme can pack multiple messages into one ciphertext and perform the same arithmetic evaluations on multiple plaintext slots by using the batching technique and single instruction multiple data (SIMD) mechanism in HE. On three public training datasets, the experimental results show that, compared with the existing schemes, the proposed scheme has better performance in terms of the encryption and decryption time of the data owner, the storage of encrypted training data, and the training time and accuracy of the model.
更多查看译文
AI 理解论文
溯源树
样例
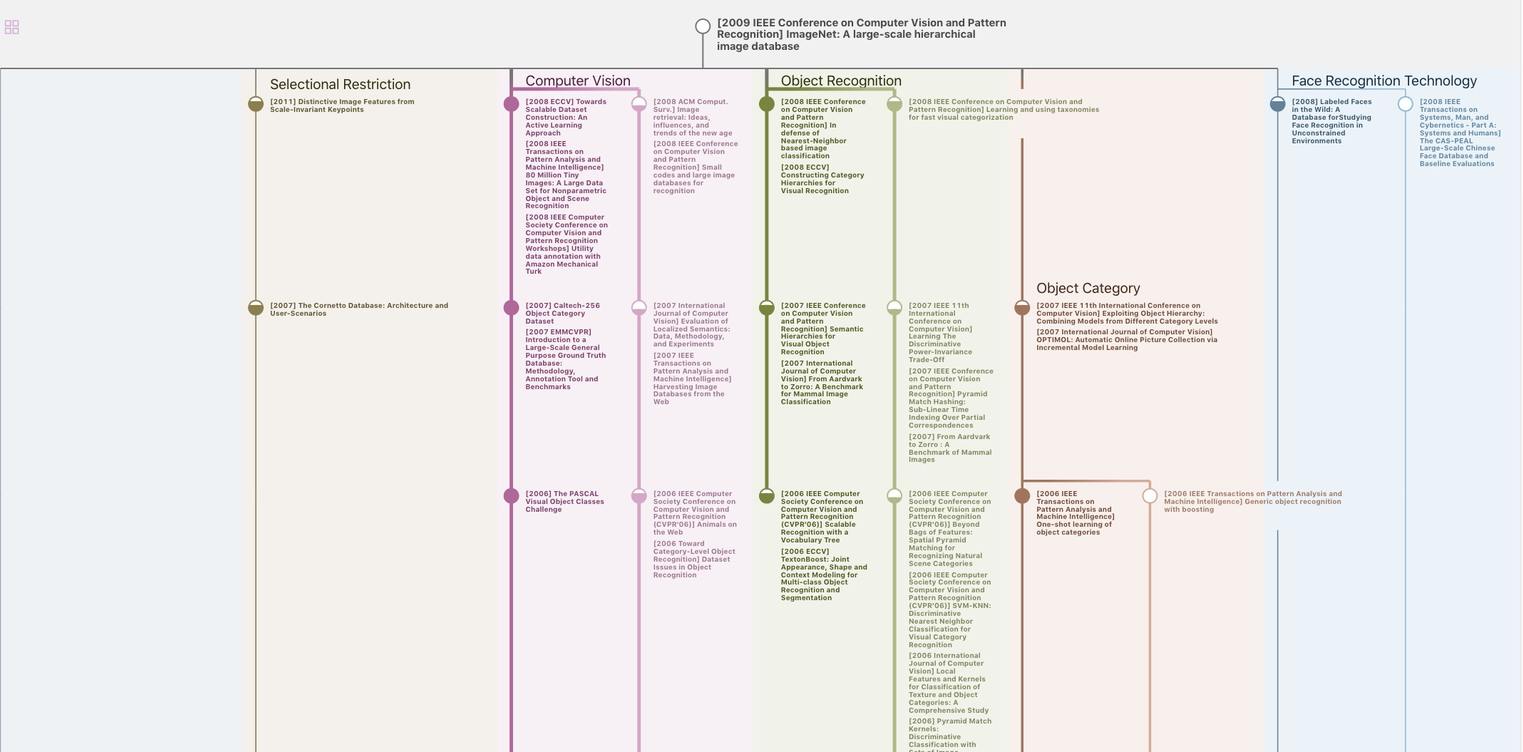
生成溯源树,研究论文发展脉络
Chat Paper
正在生成论文摘要