A Multi-channel Next POI Recommendation Framework with Multi-granularity Check-in Signals
ACM TRANSACTIONS ON INFORMATION SYSTEMS(2024)
摘要
Current study on next point-of-interest (POI) recommendation mainly explores user sequential transitions with the fine-grained individual-user POI check-in trajectories only, which suffers from the severe check-in data sparsity issue. In fact, coarse-grained signals (i.e., region- and global-level check-ins) in such sparse check-ins would also benefit to augment user preference learning. Specifically, our data analysis unveils that user movement exhibits noticeable patterns w.r.t. the regions of visited POIs. Meanwhile, the global all-user check-ins can help reflect sequential regularities shared by the crowd. We are, therefore, inspired to propose the MCMG: a Multi-Channel next POI recommendation framework with Multi-Granularity signals categorized from two orthogonal perspectives, i.e., fine-coarse grained check-ins at either POI/region level or local/global level. The MCMG is equipped with three modules, namely, global user behavior encoder, local multi-channel (i.e., region, category, and POI channels) encoder, and region-aware weighting strategy. Such design enables MCMG to be capable of capturing both fine- and coarse-grained sequential regularities as well as exploring the dynamic impact of multi-channel by differentiating the check-in patterns w.r.t. visited regions. Extensive experiments on four real-world datasets show that our MCMG significantly outperforms state-of-the-art next POI recommendation approaches.
更多查看译文
关键词
Next POI recommendation,graph neural network,self-attention,multi-channel encoder,multi-granularity,geographical region
AI 理解论文
溯源树
样例
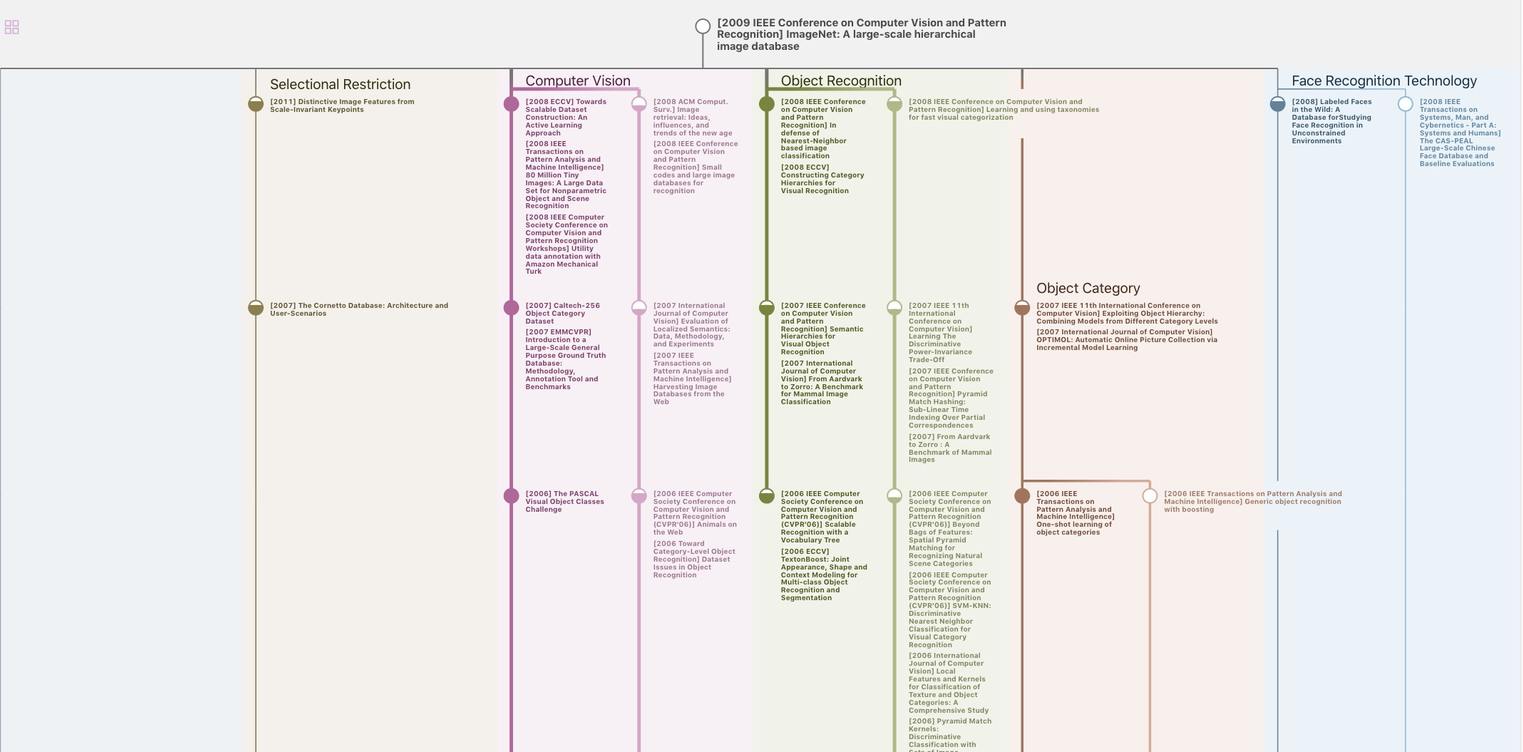
生成溯源树,研究论文发展脉络
Chat Paper
正在生成论文摘要