Particle-Filter-Based State Estimation for Delayed Artificial Neural Networks: When Probabilistic Saturation Constraints Meet Redundant Channels
IEEE TRANSACTIONS ON NEURAL NETWORKS AND LEARNING SYSTEMS(2024)
摘要
In this brief, the state estimation problem is investigated for a class of randomly delayed artificial neural networks (ANNs) subject to probabilistic saturation constraints (PSCs) and non-Gaussian noises under the redundant communication channels. A series of mutually independent Bernoulli distributed white sequences are introduced to govern the random occurrence of the time delays, the saturation constraints, and the transmission channel failures. A comprehensive redundant-channel-based communication mechanism is constructed to attenuate the phenomenon of packet dropouts so as to enhance the quality of data transmission. To compensate for the influence of randomly occurring time delays, the corresponding occurrence probability is exploited in the process of particle generation. In addition, an explicit expression of the likelihood function is established based on the statistical information to account for the impact of PSCs and redundant channels. By virtue of the modified operations of particle propagation and weight update, a particle-filter-based state estimation algorithm is proposed with mild restriction on the system type. Finally, an illustrative example with Monte Carlo simulations is provided to demonstrate the effectiveness of the developed state estimation scheme.
更多查看译文
关键词
State estimation,Neurons,Delay effects,Probabilistic logic,Channel estimation,Biological neural networks,Probability density function,Delayed artificial neural networks (ANNs),non-Gaussian noises,particle filter,probabilistic saturation constraints (PSCs),redundant communication channels
AI 理解论文
溯源树
样例
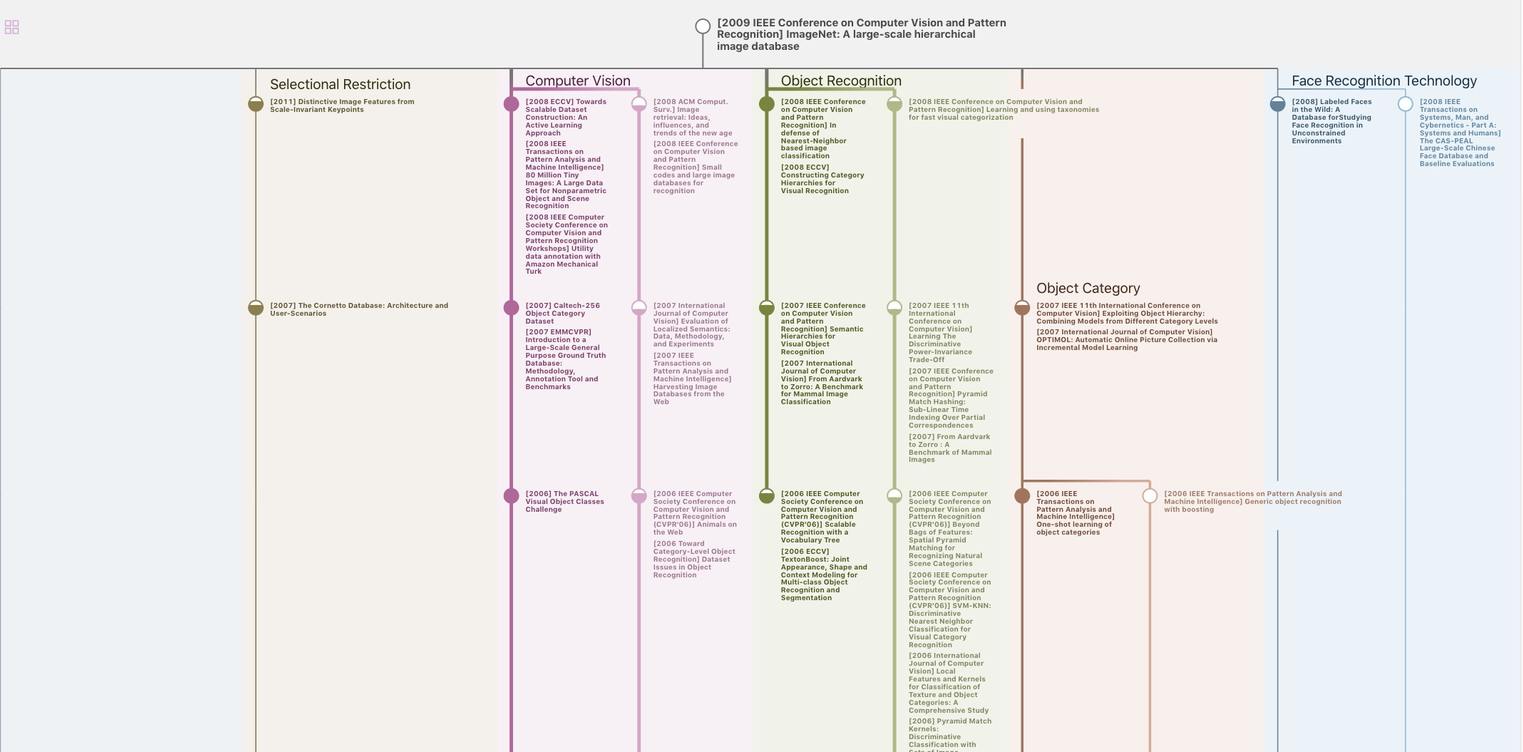
生成溯源树,研究论文发展脉络
Chat Paper
正在生成论文摘要