Towards making the most of NLP-based device mapping optimization for OpenCL kernels
2022 IEEE International Conference on Omni-layer Intelligent Systems (COINS)(2022)
摘要
Nowadays, we are living in an era of extreme device heterogeneity. Despite the high variety of conventional CPU architectures, accelerator devices, such as GPUs and FPGAs, also appear in the foreground exploding the pool of available solutions to execute applications. However, choosing the appropriate device per application needs is an extremely challenging task due to the abstract relationship between hardware and software. Automatic optimization algorithms that are accurate are required to cope with the complexity and variety of current hardware and software. Optimal execution has always relied on time-consuming trial and error approaches. Machine learning (ML) and Natural Language Processing (NLP) has flourished over the last decade with research focusing on deep architectures. In this context, the use of natural language processing techniques to source code in order to conduct autotuning tasks is an emerging field of study.In this paper, we extend the work of Cummins et al., namely Deeptune, that tackles the problem of optimal device selection (CPU or GPU) for accelerated OpenCL kernels. We identify three major limitations of Deeptune and, based on these, we propose four different DNN models that provide enhanced contextual information of source codes. Experimental results show that our proposed methodology surpasses that of Cummins et al. work, providing up to 4% improvement in prediction accuracy.
更多查看译文
关键词
Machine Learning,Natural Language Processing,OpenCL,Heterogeneous Systems
AI 理解论文
溯源树
样例
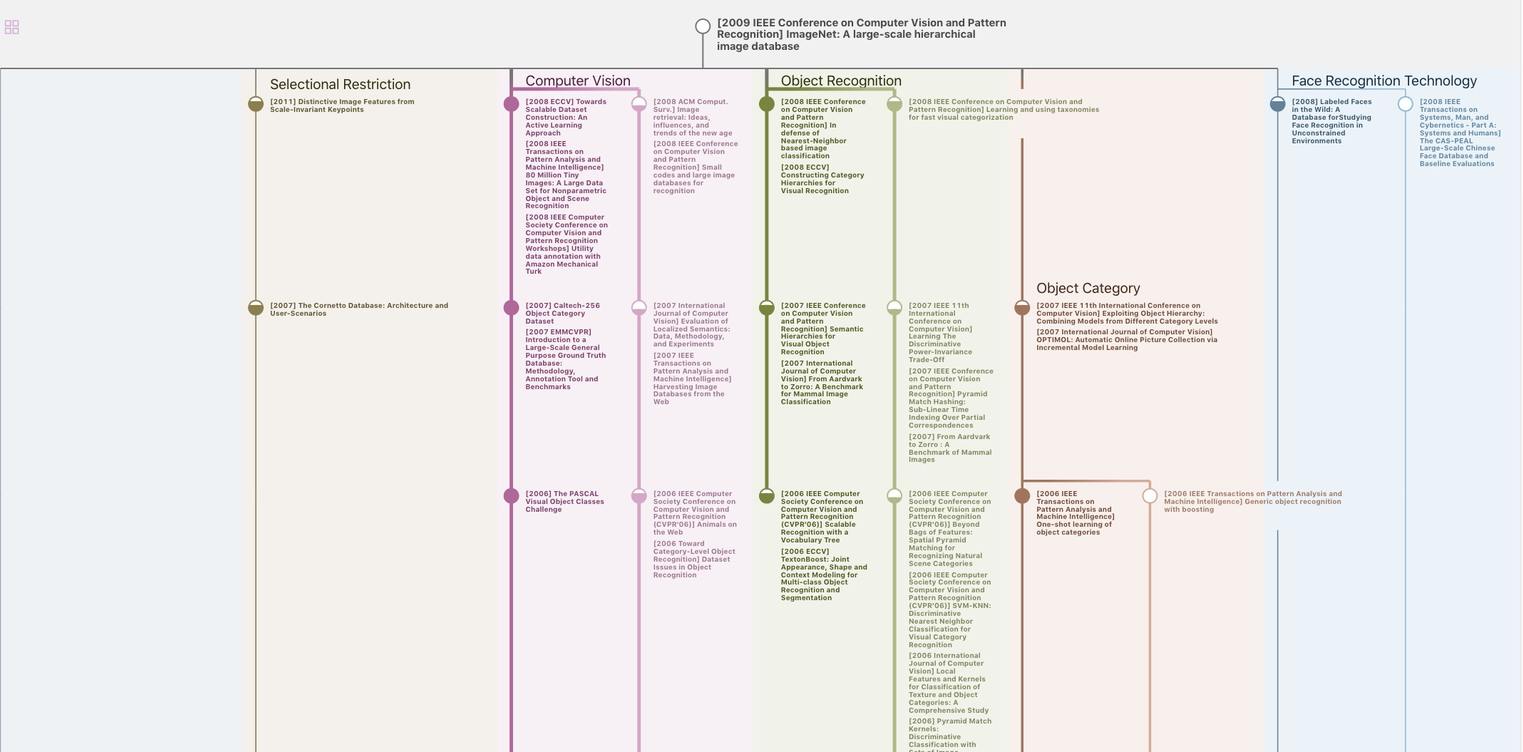
生成溯源树,研究论文发展脉络
Chat Paper
正在生成论文摘要