Automated real-space lattice extraction for atomic force microscopy images
arxiv(2023)
摘要
Analyzing atomically resolved images is a time-consuming process requiring solid experience and substantial human intervention. In addition, the acquired images contain a large amount of information such as crystal structure, presence and distribution of defects, and formation of domains, which need to be resolved to understand a material's surface structure. Therefore, machine learning techniques have been applied in scanning probe and electron microscopies during the last years, aiming for automatized and efficient image analysis. This work introduces a free and open source tool (AiSurf: Automated Identification of Surface Images) developed to inspect atomically resolved images via scale-invariant feature transform and clustering algorithms. AiSurf extracts primitive lattice vectors, unit cells, and structural distortions from the original image, with no pre-assumption on the lattice and minimal user intervention. The method is applied to various atomically resolved non-contact atomic force microscopy images of selected surfaces with different levels of complexity: anatase TiO2(101), oxygen deficient rutile TiO2(110) with and without CO adsorbates, SrTiO3(001) with Sr vacancies and graphene with C vacancies. The code delivers excellent results and is tested against atom misclassification and artifacts, thereby facilitating the interpretation of scanning probe microscopy images.
更多查看译文
关键词
machine learning,clustering algorithm,unsupervised learning,computer vision,atomic force microscopy,scanning probe microscopy,surface science
AI 理解论文
溯源树
样例
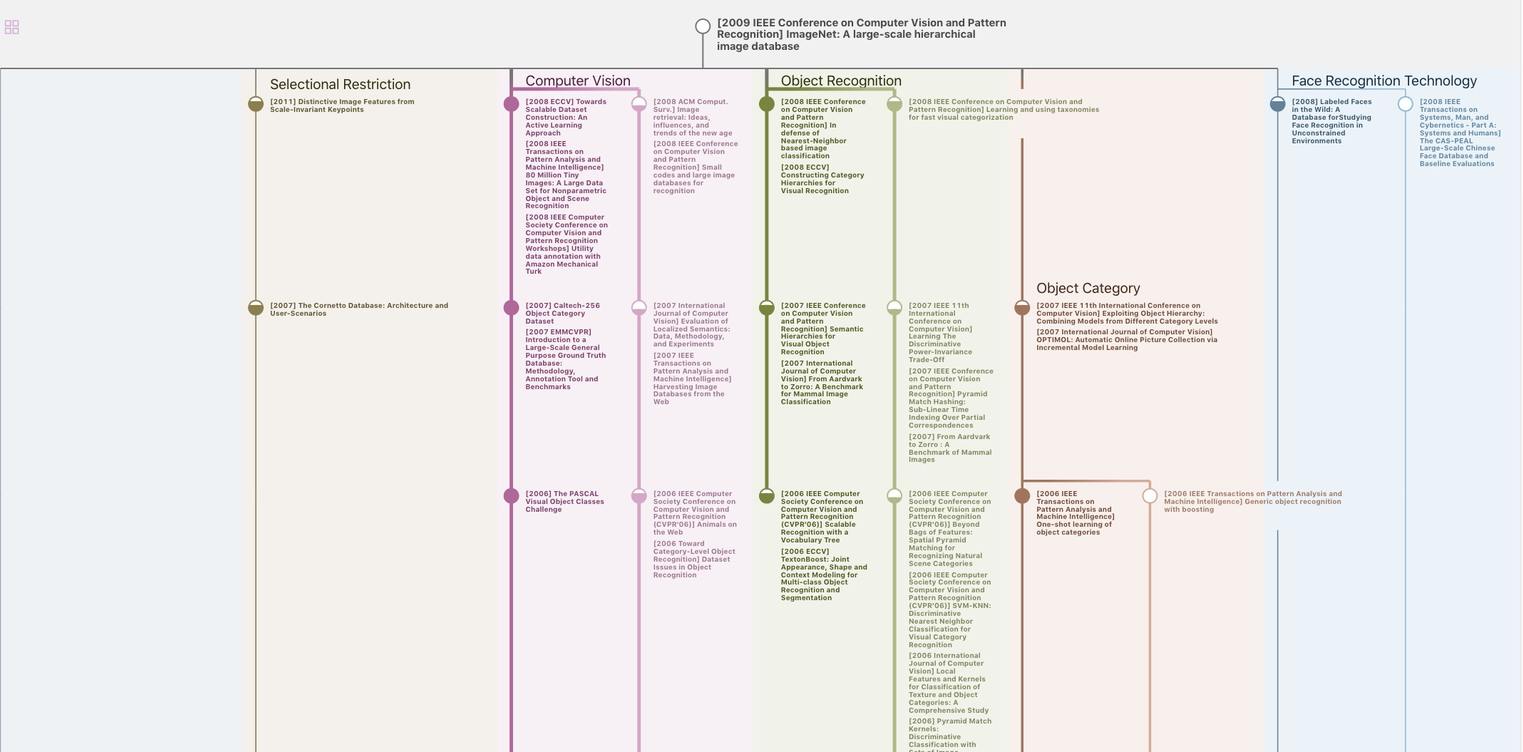
生成溯源树,研究论文发展脉络
Chat Paper
正在生成论文摘要