Music Separation Enhancement with Generative Modeling
arxiv(2022)
摘要
Despite phenomenal progress in recent years, state-of-the-art music separation systems produce source estimates with significant perceptual shortcomings, such as adding extraneous noise or removing harmonics. We propose a post-processing model (the Make it Sound Good (MSG) post-processor) to enhance the output of music source separation systems. We apply our post-processing model to state-of-the-art waveform-based and spectrogram-based music source separators, including a separator unseen by MSG during training. Our analysis of the errors produced by source separators shows that waveform models tend to introduce more high-frequency noise, while spectrogram models tend to lose transients and high frequency content. We introduce objective measures to quantify both kinds of errors and show MSG improves the source reconstruction of both kinds of errors. Crowdsourced subjective evaluations demonstrate that human listeners prefer source estimates of bass and drums that have been post-processed by MSG.
更多查看译文
关键词
separation,music,enhancement,modeling
AI 理解论文
溯源树
样例
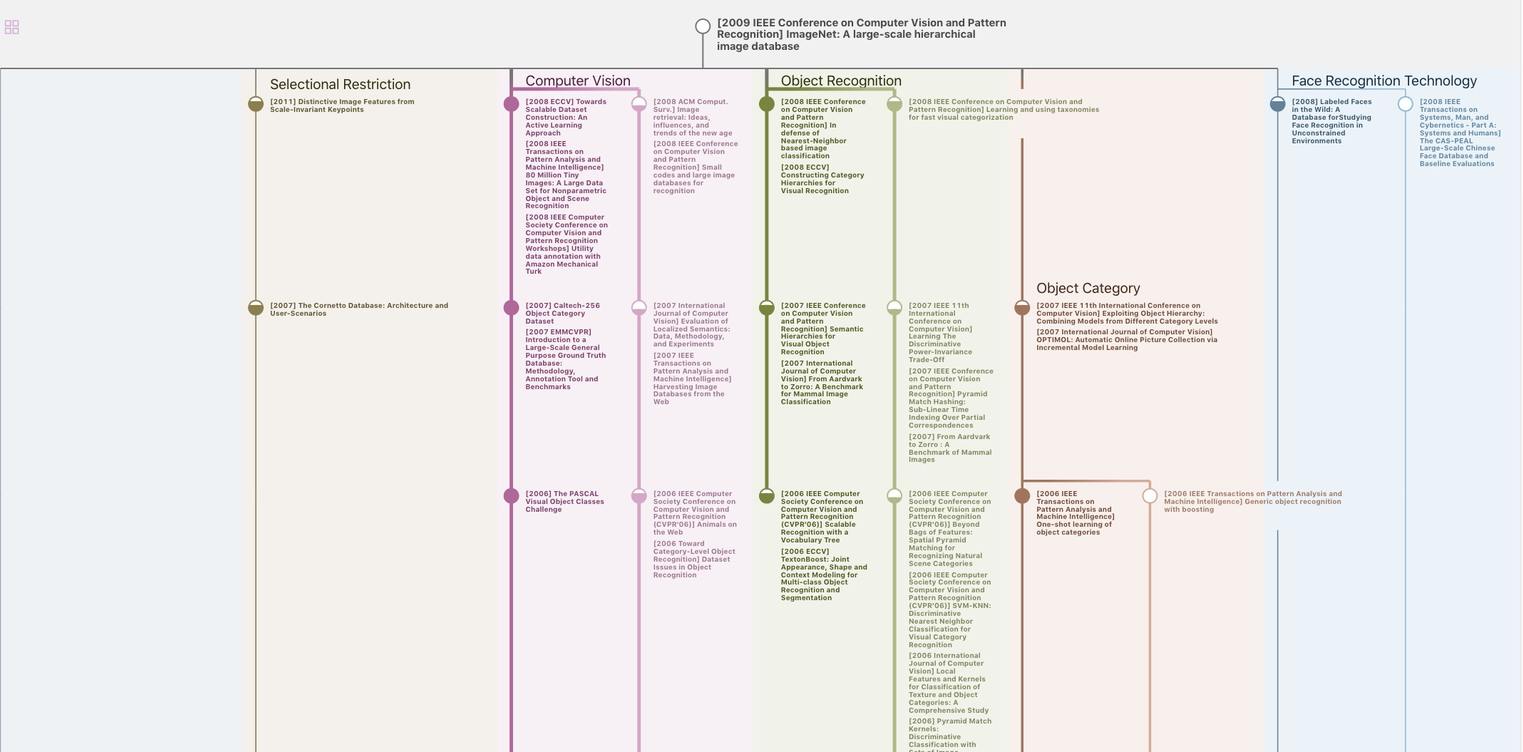
生成溯源树,研究论文发展脉络
Chat Paper
正在生成论文摘要