An Automatic Detection Approach for Wearing Safety Helmets on Construction Site based on YOLOv5
2022 IEEE 11th Data Driven Control and Learning Systems Conference (DDCLS)(2022)
摘要
The environment of construction site is generally complex and uncertain, and there may be different kinds of unexpected potential risks. Therefore, it is necessary for construction workers to wear safety helmets on construction site, which saves their lives on sudden accidents. The traditional way to handle helmet wearing issue is through manual inspection and video surveillance, which has poor efficiency and lacks immediate warning. To solve this issue, the authors propose a helmet wearing detection approach based on YOLOv5 and annotate a dataset with 3, 965 images. Then, the authors divide labelled dataset into training set (3, 108 images) and validation set (857 images) to train the neural network with the pre-training weight YOLOv5s.pt. Lastly, the authors use the trained neural network to predict real scene images collected from the construction site. Experimental results indicate that safety helmet wearing detection using the trained YOLOv5 network performs well in term of high precision rate, high recall rate, low training classification loss, and low training objectness loss. In addition, it is capable of detecting safety helmets precisely as well as making a fair distinction between different categories.
更多查看译文
关键词
Object detection,Safety helmet detection,YOLOv5,Deep learning
AI 理解论文
溯源树
样例
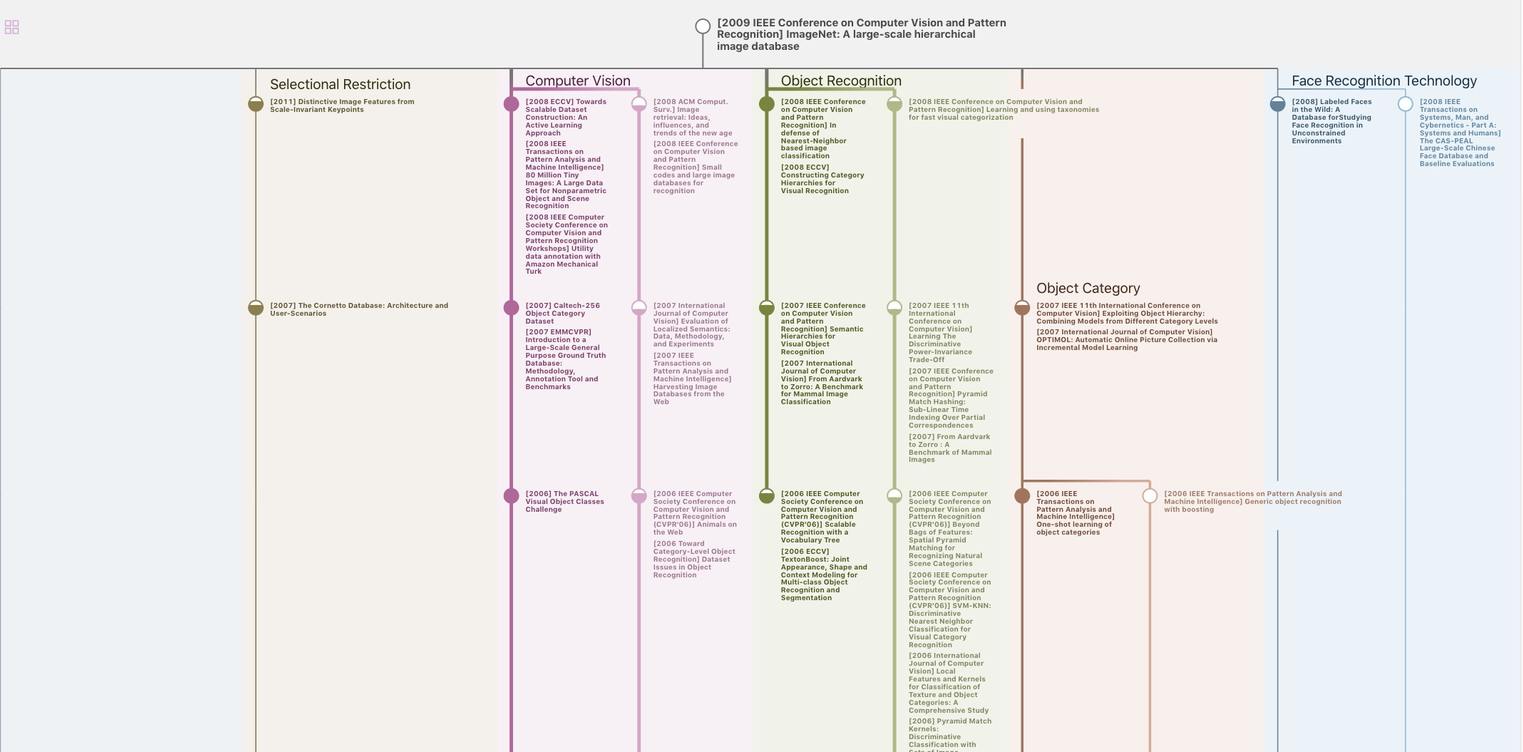
生成溯源树,研究论文发展脉络
Chat Paper
正在生成论文摘要