An Intelligent Vision-Based Nutritional Assessment Method for Handheld Food Items
IEEE TRANSACTIONS ON MULTIMEDIA(2023)
摘要
Dietary assessment has proven to be effective to evaluate the dietary intake of patients with diabetes and obesity. The traditional approach of accessing the dietary intake is to conduct a 24-hour dietary recall, a structured interview designed to obtain information on food categories and volume consumed by the participants. Due to unconscious biases in this kind of self-reporting approaches, many research studies have explored the use of vision-based approaches to provide accurate and objective assessments. Despite the promising results of food recognition by deep neural networks, there still exist several hurdles in deep learning-based food volume estimation ranging from domain shift between synthetic and raw 3D models, shape completion ambiguity and lack of large-scale paired training dataset. Therefore, this paper proposed an intelligent nutritional assessment approach via weakly-supervised point cloud completion, which aims to close the reality gap in 3D point cloud completion tasks and address the targeted challenges. Then the volume can be easily estimated from the completed representation of the food. Another major merit of our system is that it can be used to estimate the volume of handheld food items without requiring the constraints including placing the food items on a table or next to fiducial markers, which facilitates the implementation on both wearable and handheld cameras. Comprehensive experiments have been carried out on major benchmark datasets and self-constructed volume-annotated dataset respectively, in which the proposed method demonstrates comparable results with several strong fully-supervised baseline methods and shows superior completion ability in handling food volume estimation.
更多查看译文
关键词
Volume estimation,3D point cloud,weak supervision,domain adaptation,auxiliary classifier GAN
AI 理解论文
溯源树
样例
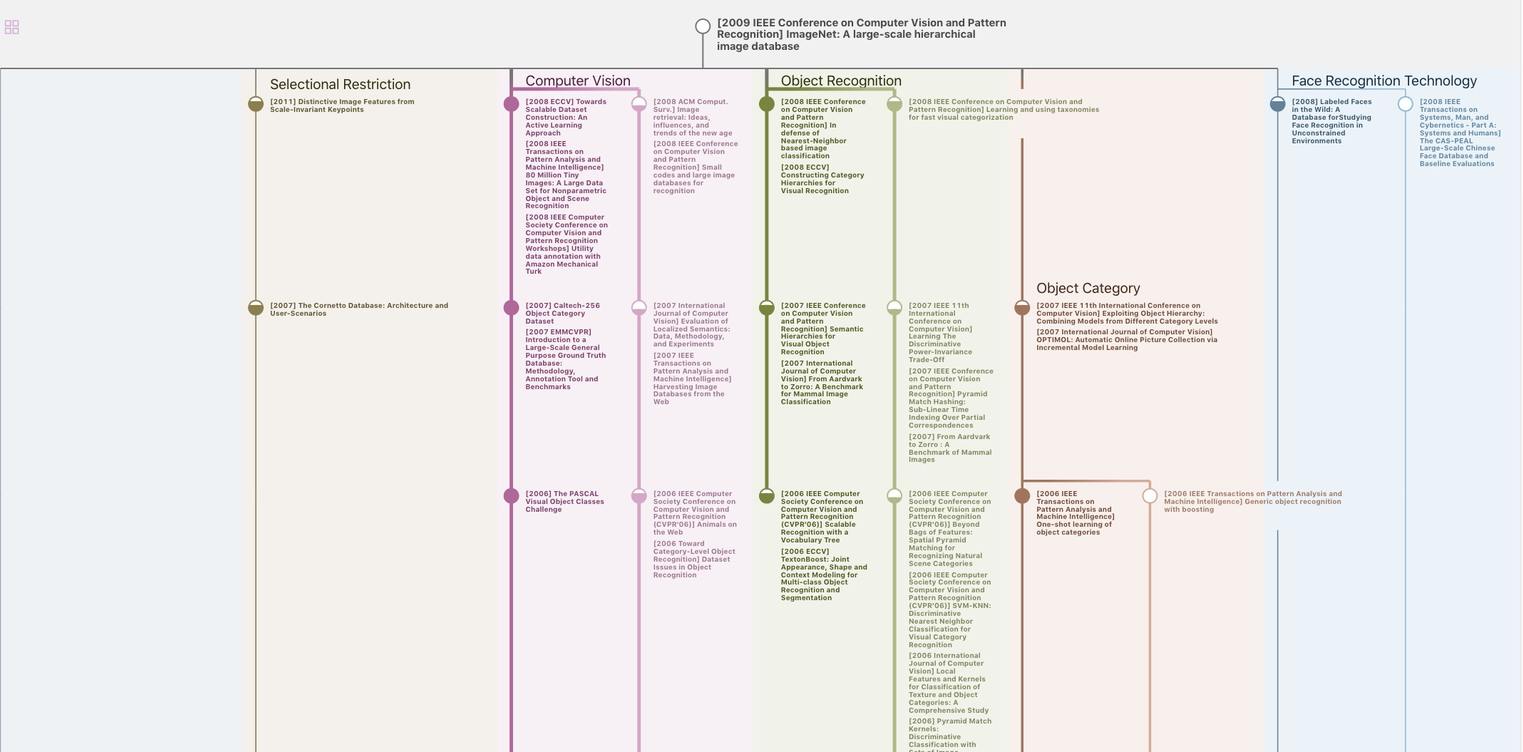
生成溯源树,研究论文发展脉络
Chat Paper
正在生成论文摘要