Induced Natural Language Rationales and Interleaved Markup Tokens Enable Extrapolation in Large Language Models
arxiv(2022)
摘要
The ability to extrapolate, i.e., to make predictions on sequences that are longer than those presented as training examples, is a challenging problem for current deep learning models. Recent work shows that this limitation persists in state-of-the-art Transformer-based models. Most solutions to this problem use specific architectures or training methods that do not generalize to other tasks. We demonstrate that large language models can succeed in extrapolation without modifying their architecture or training procedure. Our experimental results show that generating step-by-step rationales and introducing marker tokens are both required for effective extrapolation. First, we induce a language model to produce step-by-step rationales before outputting the answer to effectively communicate the task to the model. However, as sequences become longer, we find that current models struggle to keep track of token positions. To address this issue, we interleave output tokens with markup tokens that act as explicit positional and counting symbols. Our findings show how these two complementary approaches enable remarkable sequence extrapolation and highlight a limitation of current architectures to effectively generalize without explicit surface form guidance. Code available at https://github.com/MirelleB/induced-rationales-markup-tokens
更多查看译文
关键词
large language models,interleaved markup tokens enable
AI 理解论文
溯源树
样例
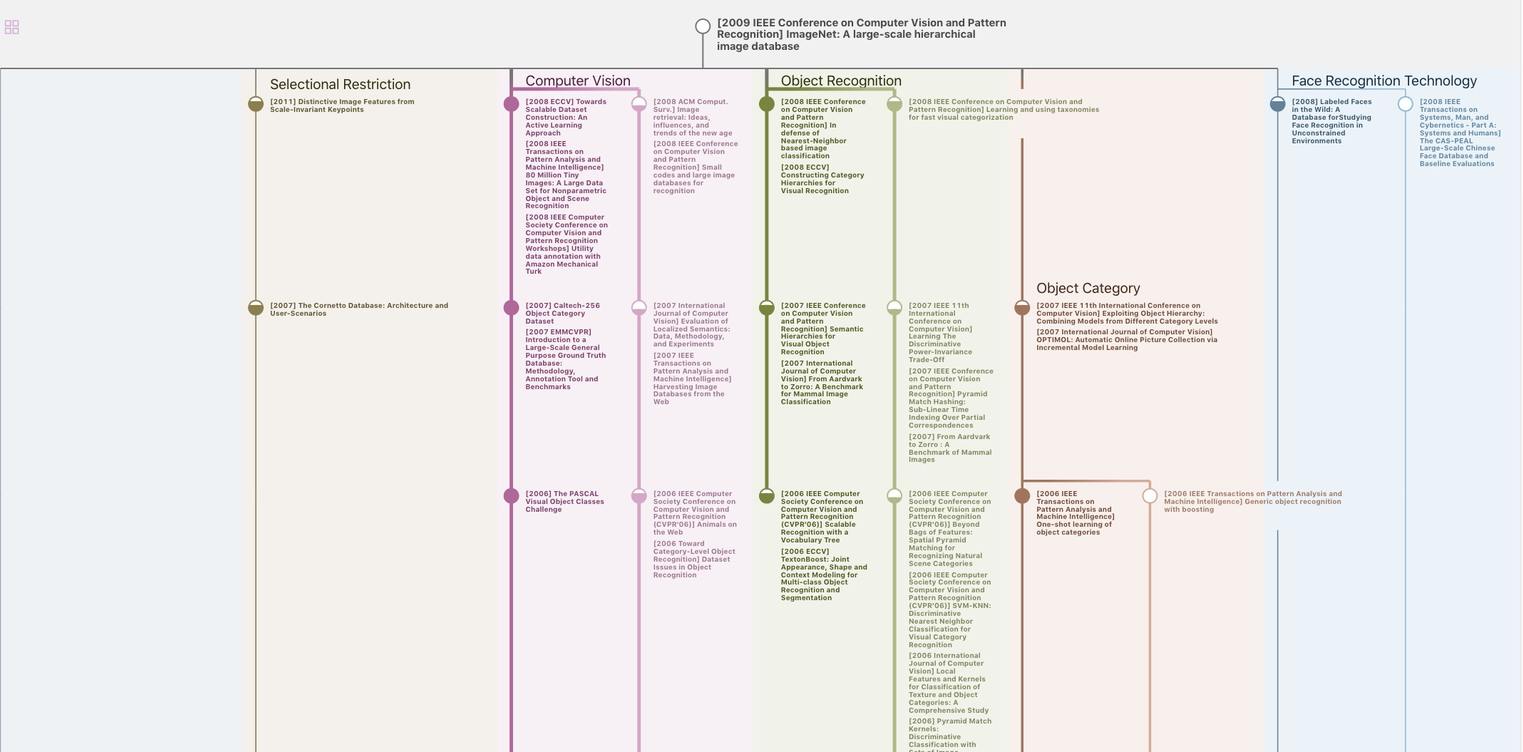
生成溯源树,研究论文发展脉络
Chat Paper
正在生成论文摘要