Efficient Data-Driven Network Functions
2022 30th International Symposium on Modeling, Analysis, and Simulation of Computer and Telecommunication Systems (MASCOTS)(2022)
摘要
Cloud environments require dynamic and adaptive networking policies. It is preferred to use heuristics over advanced learning algorithms in Virtual Network Functions (VNFs) in production because of high-performance constraints. This paper proposes Aquarius to passively yet efficiently gather observations and enable the use of machine learning to collect, infer, and supply accurate networking state information - without incurring additional signaling and management overhead. This paper illustrates the use of Aquarius with a traffic classifier, an auto-scaling system, and a load balancer - and demonstrates the use of three different machine learning paradigms - unsupervised, supervised, and reinforcement learning, within Aquarius, for inferring network state. Testbed evaluations show that Aquarius increases network state visibility and brings notable performance gains with low overhead.
更多查看译文
关键词
Virtual Network Functions,high performance network,data-driven,cloud,performance evaluation
AI 理解论文
溯源树
样例
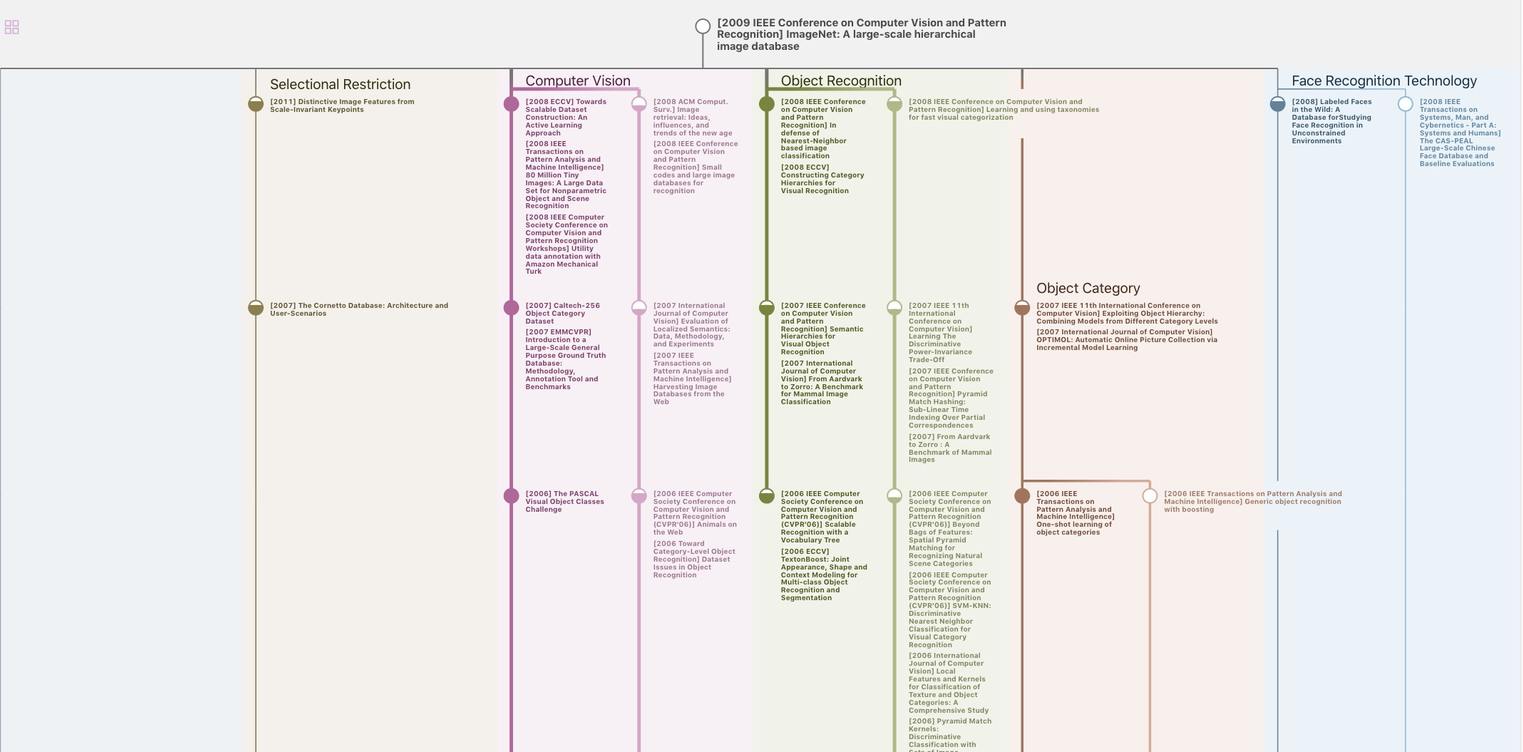
生成溯源树,研究论文发展脉络
Chat Paper
正在生成论文摘要