FashionVQA: A Domain-Specific Visual Question Answering System
arxiv(2022)
摘要
Humans apprehend the world through various sensory modalities, yet language is their predominant communication channel. Machine learning systems need to draw on the same multimodal richness to have informed discourses with humans in natural language; this is particularly true for systems specialized in visually-dense information, such as dialogue, recommendation, and search engines for clothing. To this end, we train a visual question answering (VQA) system to answer complex natural language questions about apparel in fashion photoshoot images. The key to the successful training of our VQA model is the automatic creation of a visual question-answering dataset with 168 million samples from item attributes of 207 thousand images using diverse templates. The sample generation employs a strategy that considers the difficulty of the question-answer pairs to emphasize challenging concepts. Contrary to the recent trends in using several datasets for pretraining the visual question answering models, we focused on keeping the dataset fixed while training various models from scratch to isolate the improvements from model architecture changes. We see that using the same transformer for encoding the question and decoding the answer, as in language models, achieves maximum accuracy, showing that visual language models (VLMs) make the best visual question answering systems for our dataset. The accuracy of the best model surpasses the human expert level, even when answering human-generated questions that are not confined to the template formats. Our approach for generating a large-scale multimodal domain-specific dataset provides a path for training specialized models capable of communicating in natural language. The training of such domain-expert models, e.g., our fashion VLM model, cannot rely solely on the large-scale general-purpose datasets collected from the web.
更多查看译文
关键词
168 million samples,207 thousand images,answer complex natural language questions,domain-expert models,domain-specific visual question answering system,fashion photoshoot images,fashion VLM model,human expert level,large-scale multimodal domain-specific dataset,machine learning systems,model surpasses,multimodal richness,optimal visual question-answering systems,predominant communication channel,question-answer pairs,sensory modalities,successful training,training specialized models,visual language models,visual question-answering dataset,visual question-answering system,visually-dense information,VQA model
AI 理解论文
溯源树
样例
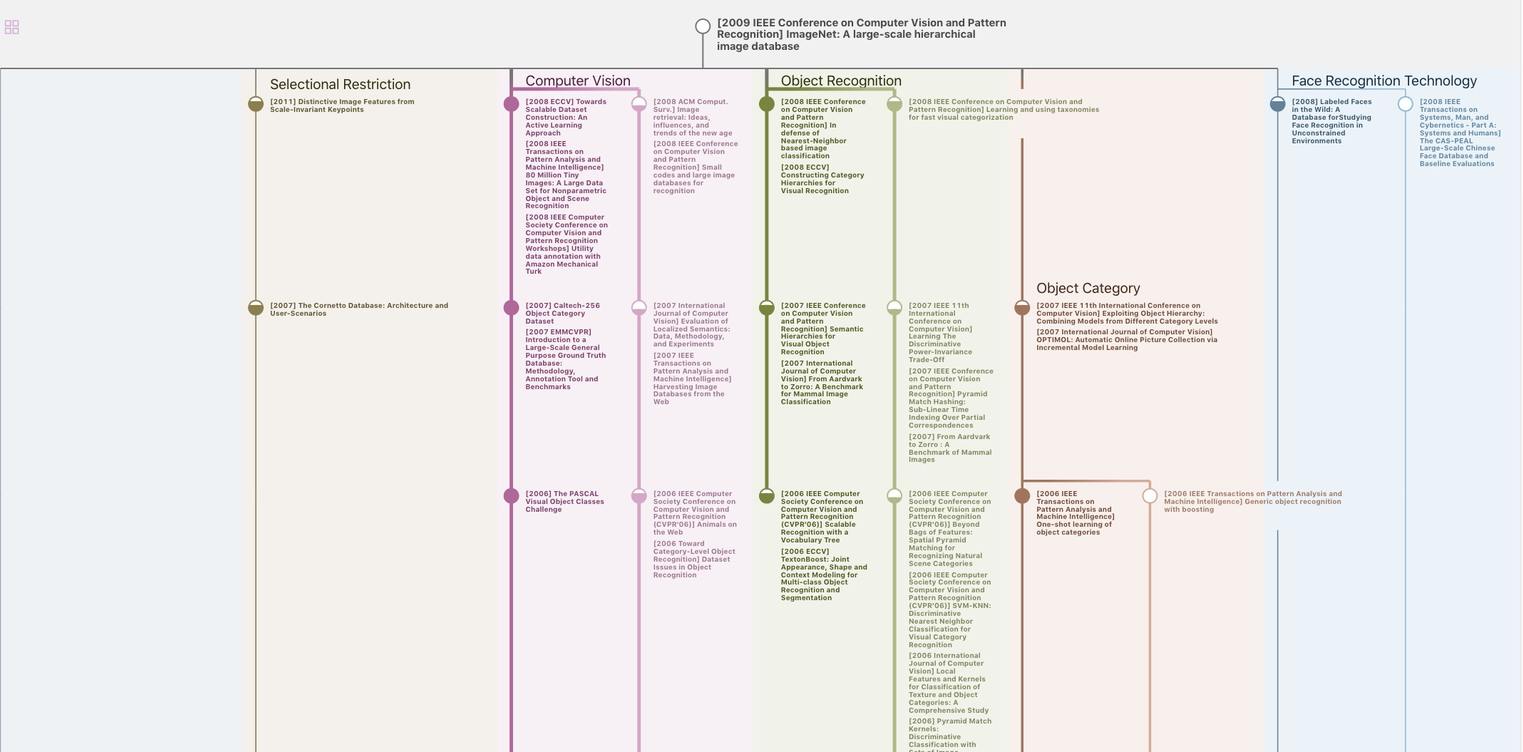
生成溯源树,研究论文发展脉络
Chat Paper
正在生成论文摘要