Hilti-Oxford Dataset: A Millimeter-Accurate Benchmark for Simultaneous Localization and Mapping
IEEE Robotics and Automation Letters(2023)
摘要
Simultaneous Localization and Mapping (SLAM) is being deployed in real-world applications, however many state-of-the-art solutions still struggle in many common scenarios. A key necessity in progressing SLAM research is the availability of high-quality datasets and fair and transparent benchmarking. To this end, we have created the Hilti-Oxford Dataset, to push state-of-the-art SLAM systems to their limits. The dataset has a variety of challenges ranging from sparse and regular construction sites to a 17th century neoclassical building with fine details and curved surfaces. To encourage multi-modal SLAM approaches, we designed a data collection platform featuring a lidar, five cameras, and an IMU (Inertial Measurement Unit). With the goal of benchmarking SLAM algorithms for tasks where accuracy and robustness are paramount, we implemented a novel ground truth collection method that enables our dataset to accurately measure SLAM pose errors with millimeter accuracy. To further ensure accuracy, the extrinsics of our platform were verified with a micrometer-accurate scanner, and temporal calibration was managed online using hardware time synchronization. The multi-modality and diversity of our dataset attracted a large field of academic and industrial researchers to enter the second edition of the Hilti SLAM challenge, which concluded in June 2022. The results of the challenge show that while the top three teams could achieve an accuracy of 2 cm or better for some sequences, the performance dropped off in more difficult sequences.
更多查看译文
关键词
Data sets for SLAM,SLAM,mapping
AI 理解论文
溯源树
样例
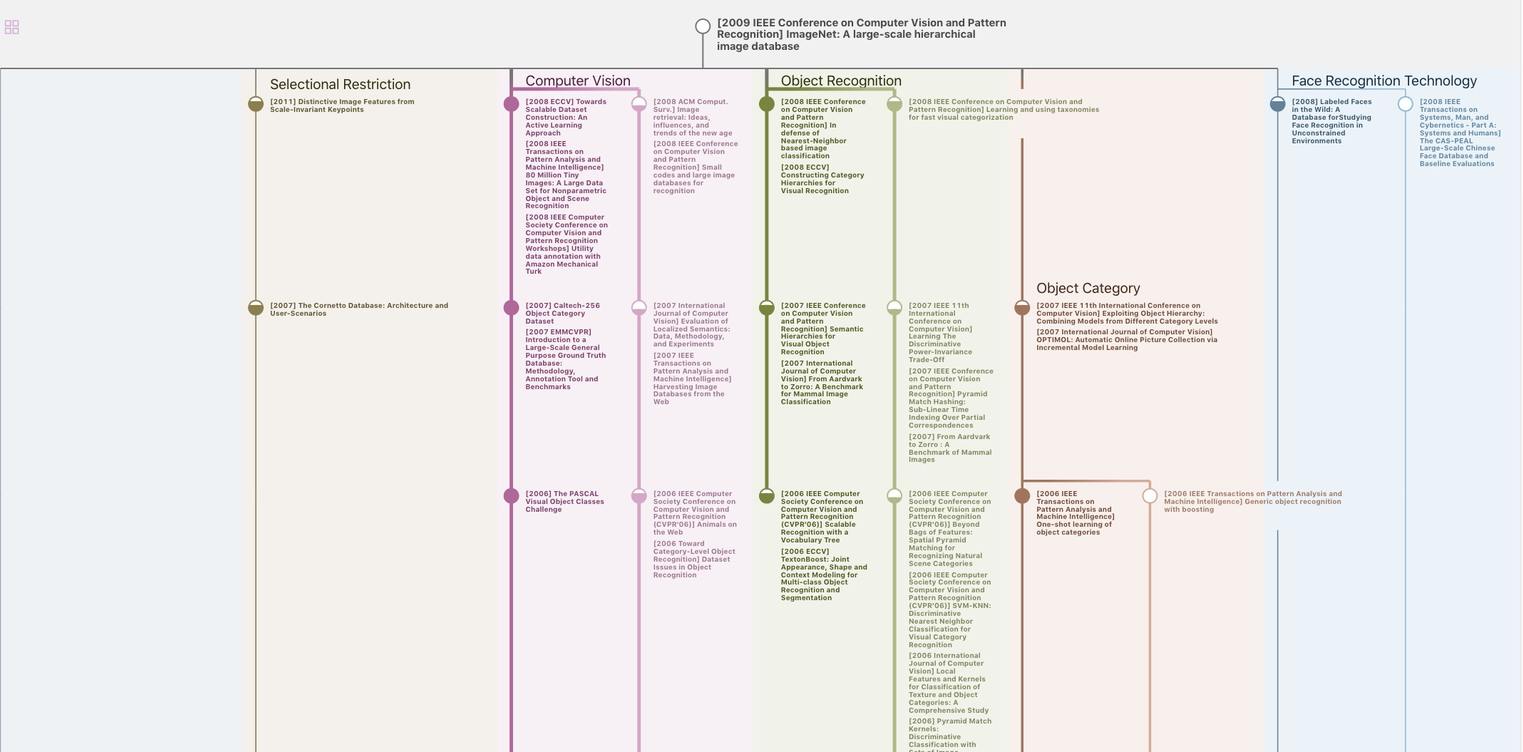
生成溯源树,研究论文发展脉络
Chat Paper
正在生成论文摘要