Lost in Context? On the Sense-Wise Variance of Contextualized Word Embeddings
IEEE-ACM TRANSACTIONS ON AUDIO SPEECH AND LANGUAGE PROCESSING(2024)
摘要
Contextualized word embeddings in language models have given much advance to NLP. Intuitively, sentential information is integrated into the representation of words, which can help model polysemy. However, context sensitivity also leads to the variance of representations, which may break the semantic consistency for synonyms. Previous works that investigate contextualized sensitivity focus on the token level representations, while we are taking a deeper dive into exploring representations at the fine-grained sense level. In particular, we quantify how much the contextualized embeddings of each word sense vary across contexts in typical pre-trained models, the results show that contextualized embeddings can be highly consistent across contexts, even for two different words with the same sense. In addition, part-of-speech, number of word senses, and sentence length have an influence on the variance of sense representations. Interestingly, we find that word representations are position-biased, where the first words in different contexts tend to be more similar. We analyze such a phenomenon and also propose a prompt-augmentation method to alleviate such bias in distance-based word sense disambiguation settings. Finally, we investigate the influence of sense-level pre-training on the performance of different downstream tasks, results show that such external tasks can improve the sense- and syntactic-related tasks, while not necessarily benefiting general language understanding tasks.
更多查看译文
关键词
Language models,contextualized word embeddings,sense-wise variance,position bias
AI 理解论文
溯源树
样例
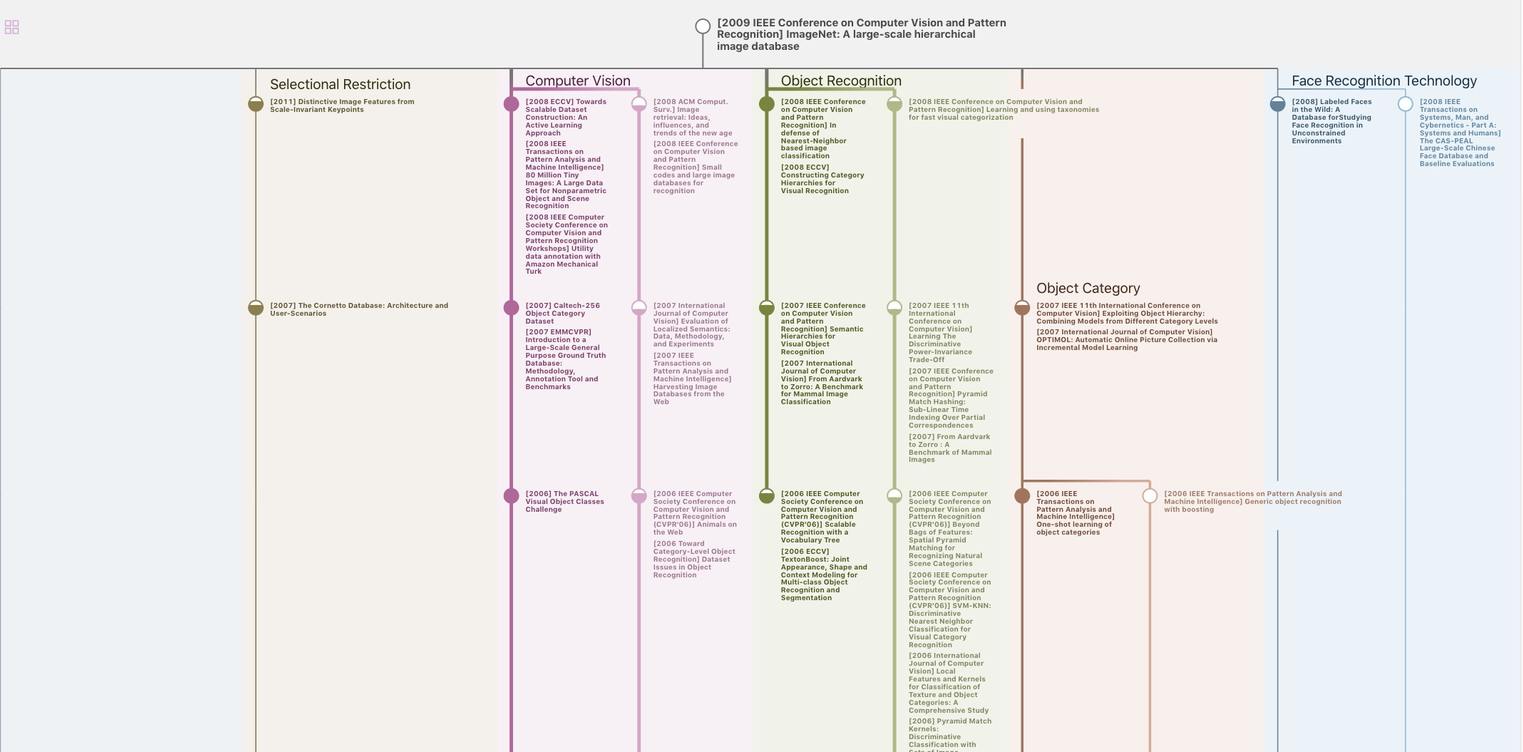
生成溯源树,研究论文发展脉络
Chat Paper
正在生成论文摘要