Advances in Exploratory Data Analysis, Visualisation and Quality for Data Centric AI Systems
KDD '22: Proceedings of the 28th ACM SIGKDD Conference on Knowledge Discovery and Data Mining(2022)
摘要
It is widely accepted that data preparation is one of the most time-consuming steps of the machine learning (ML) lifecycle. It is also one of the most important steps, as the quality of data directly influences the quality of a model. In this tutorial, we will discuss the importance and the role of exploratory data analysis (EDA) and data visualisation techniques to find data quality issues and for data preparation, relevant to building ML pipelines. We will also discuss the latest advances in these fields and bring out areas that need innovation. To make the tutorial actionable for practitioners, we will also discuss the most popular open-source packages that one can get started with along with their strengths and weaknesses. Finally, we will discuss on the challenges posed by industry workloads and the gaps to be addressed to make data-centric AI real in industry settings.
更多查看译文
关键词
exploratory data analysis,visualisation,data analysis
AI 理解论文
溯源树
样例
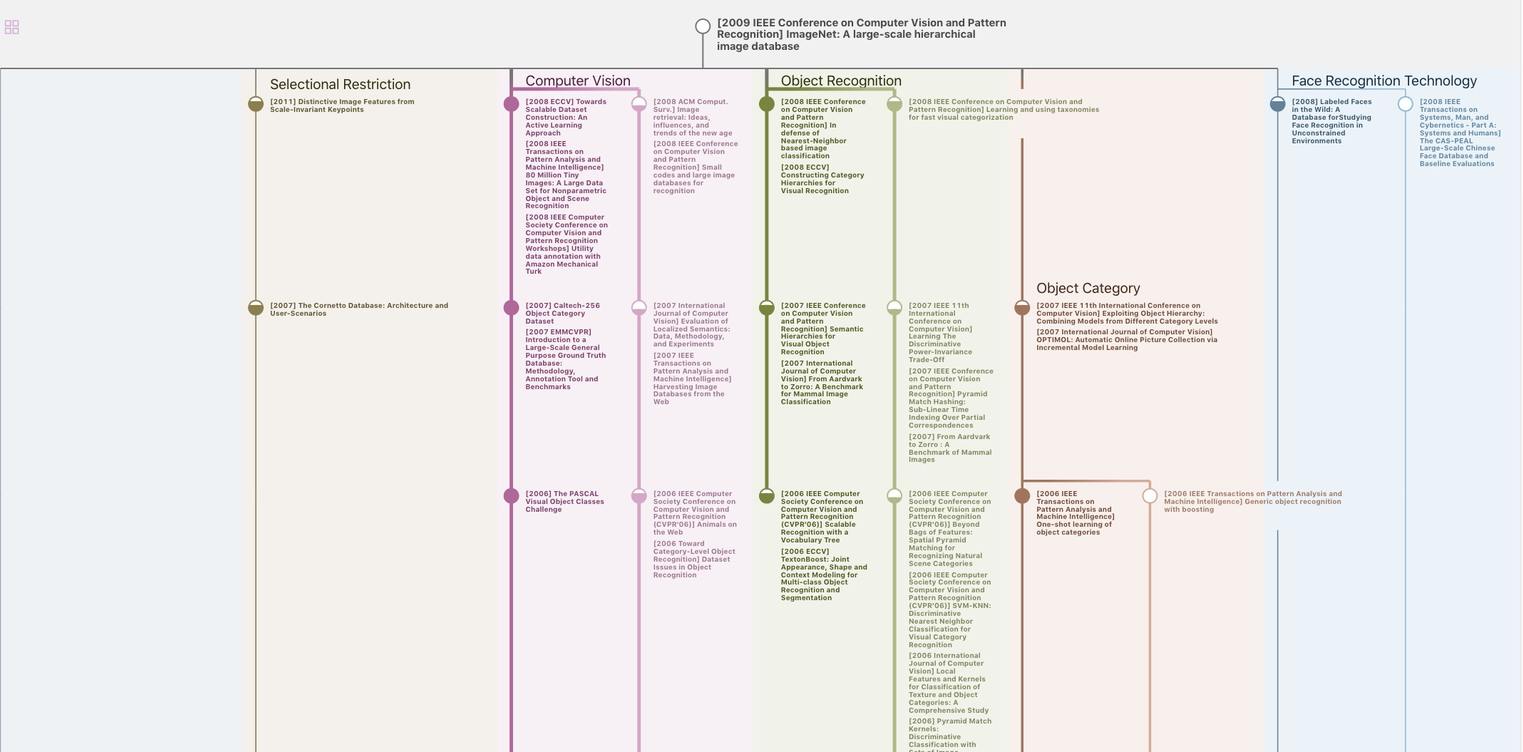
生成溯源树,研究论文发展脉络
Chat Paper
正在生成论文摘要