ReprBERT: Distilling BERT to an Efficient Representation-Based Relevance Model for E-Commerce
KDD '22: Proceedings of the 28th ACM SIGKDD Conference on Knowledge Discovery and Data Mining(2022)
摘要
Text relevance or text matching of query and product is an essential technique for e-commerce search engine, which helps users find the desirable products and is also crucial to ensuring user experience. A major difficulty for e-commerce text relevance is the severe vocabulary gap between query and product. Recently, neural networks have been the mainstream for the text matching task owing to the better performance for semantic matching. Practical e-commerce relevance models are usually representation-based architecture, which can pre-compute representations offline and are therefore online efficient. Interaction-based models, although can achieve better performance, are mostly time-consuming and hard to be deployed online. Recently BERT has achieved significant progress on many NLP tasks including text matching, and it is of great value but also big challenge to deploy BERT to the e-commerce relevance task. To realize this goal, we propose ReprBERT, which has the advantages of both excellent performance and low latency, by distilling the interaction-based BERT model to a representation-based architecture. To reduce the performance decline, we investigate the key reasons and propose two novel interaction strategies to resolve the absence of representation interaction and low-level semantic interaction. Finally, ReprBERT can achieve only about 1.5% AUC loss from the interaction-based BERT, but has more than 10% AUC improvement compared to previous state-of-the-art representation-based models. ReprBERT has already been deployed on the search engine of Taobao and serving the entire search traffic, achieving significant gain of user experience and business profit.
更多查看译文
关键词
distilling reprbert,relevance model,representation-based,e-commerce
AI 理解论文
溯源树
样例
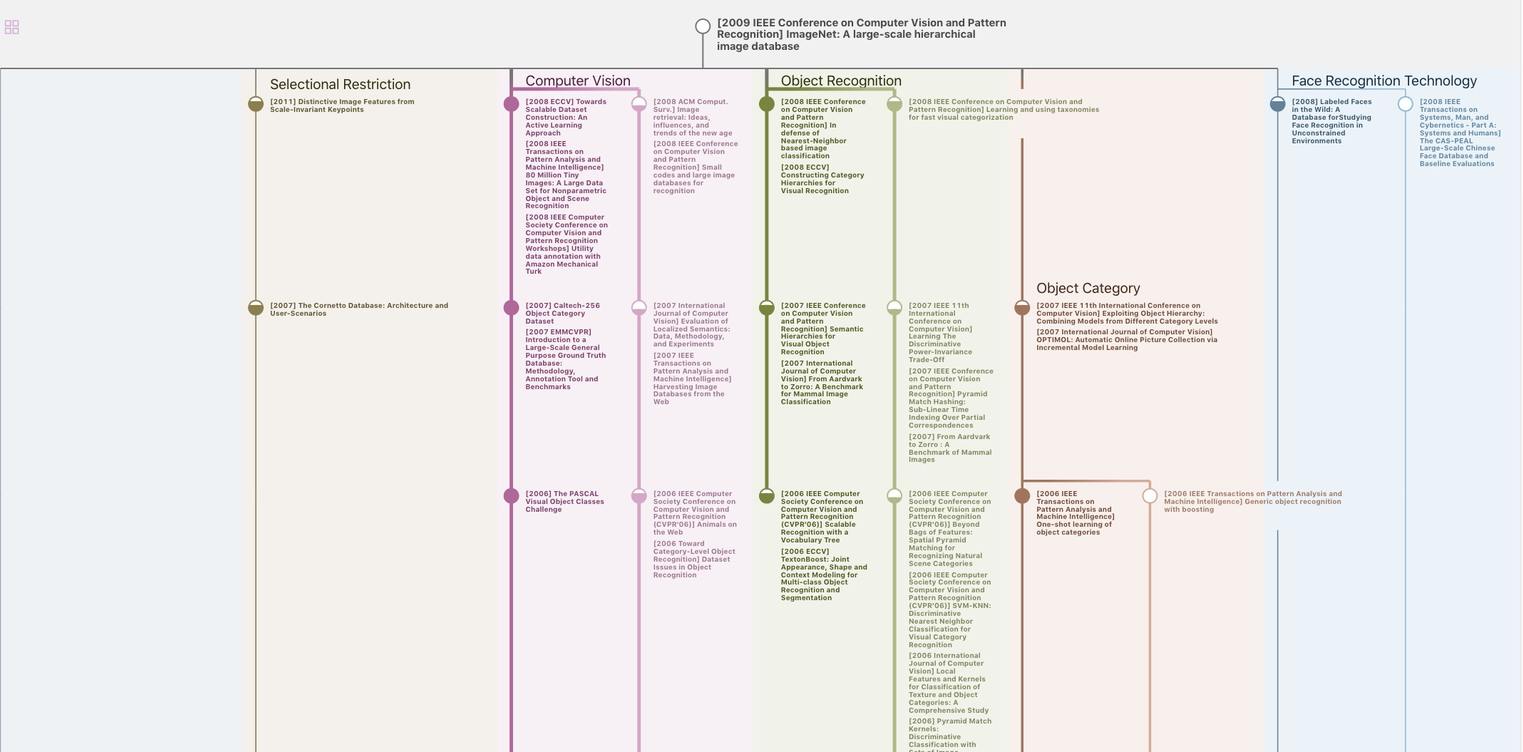
生成溯源树,研究论文发展脉络
Chat Paper
正在生成论文摘要