Bayesian transfer learning with active querying for intelligent cross-machine fault prognosis under limited data
Mechanical Systems and Signal Processing(2023)
摘要
Most existing deep learning (DL)-based health prognostic methods assume that the training and testing datasets are from identical machines operating under similar conditions requiring massive labelled condition monitoring (CM) data to guarantee the prediction accuracy and generalisation capacity. However, these strict restrictions significantly hinder the deployment of the dl-based prognostic methods in real industries. In this paper, a Bayesian semi-supervised transfer learning with active querying-based intelligent fault prognostic framework is developed for remaining useful life (RUL) prediction across completely different machines under limited data. The proposed method strategically integrates the advantages of transfer learning (TL) and active learning in the Bayesian deep learning (BDL) framework. In the proposed framework, Bayesian neural networks with Monte Carlo dropout inference are utilised to quantify RUL prediction uncertainty, which is further leveraged to develop an active querying-based training data selection mechanism. Moreover, TL is simultaneously embedded into the BDL framework to relieve data distribution discrepancies existing among the completely different machines. The experimental verifications from open-sourced bearing datasets and lab testing-based lithium-ion battery degradation datasets demonstrate that the proposed framework can effectively and reliably achieve bi-directional transfer fault prognostic tasks under limited labelled CM data in target domain. Finally, generalisation and superiority of the proposed method are also validated by comparing with other state-of-the-art methods.
更多查看译文
关键词
Transfer learning,Domain adaptation,Remaining useful life prediction,Transfer across different machines,Prognosis,Rolling bearings
AI 理解论文
溯源树
样例
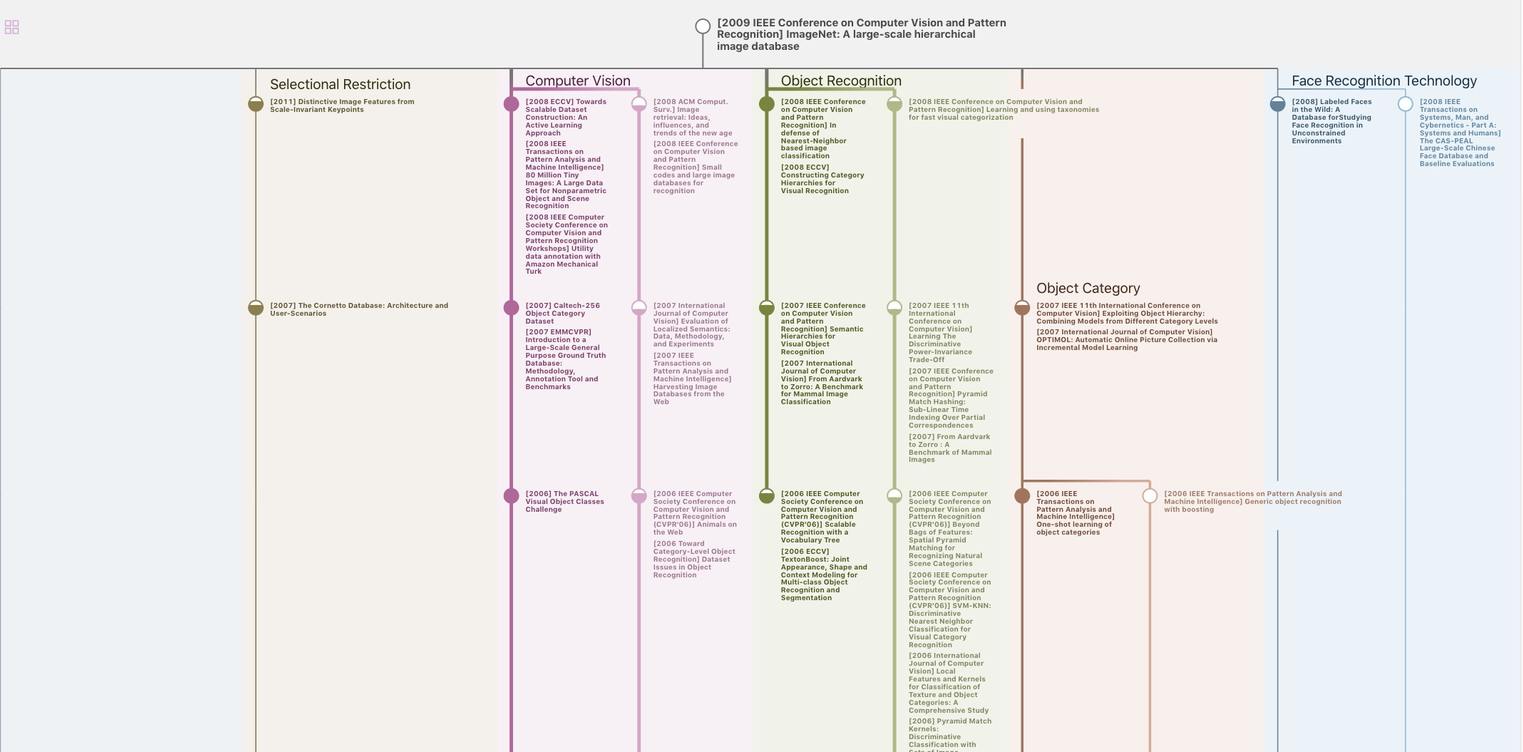
生成溯源树,研究论文发展脉络
Chat Paper
正在生成论文摘要