L3: Accelerator-Friendly Lossless Image Format for High-Resolution, High-Throughput DNN Training.
European Conference on Computer Vision(2022)
摘要
The training process of deep neural networks (DNNs) is usually pipelined with stages for data preparation on CPUs followed by gradient computation on accelerators like GPUs. In an ideal pipeline, the end-to-end training throughput is eventually limited by the throughput of the accelerator, not by that of data preparation. In the past, the DNN training pipeline achieved a near-optimal throughput by utilizing datasets encoded with a lightweight, lossy image format like JPEG. However, as high-resolution, losslessly-encoded datasets become more popular for applications requiring high accuracy, a performance problem arises in the data preparation stage due to low-throughput image decoding on the CPU. Thus, we propose L3, a custom lightweight, lossless image format for high-resolution, high-throughput DNN training. The decoding process of L3 is effectively parallelized on the accelerator, thus minimizing CPU intervention for data preparation during DNN training. L3 achieves a 9.29\(\times \) higher data preparation throughput than PNG, the most popular lossless image format, for the Cityscapes dataset on NVIDIA A100 GPU, which leads to 1.71\(\times \) higher end-to-end training throughput. Compared to JPEG and WebP, two popular lossy image formats, L3 provides up to 1.77\(\times \) and 2.87\(\times \) higher end-to-end training throughput for ImageNet, respectively, at equivalent metric performance.
更多查看译文
关键词
DNN training,Data preparation,Image processing
AI 理解论文
溯源树
样例
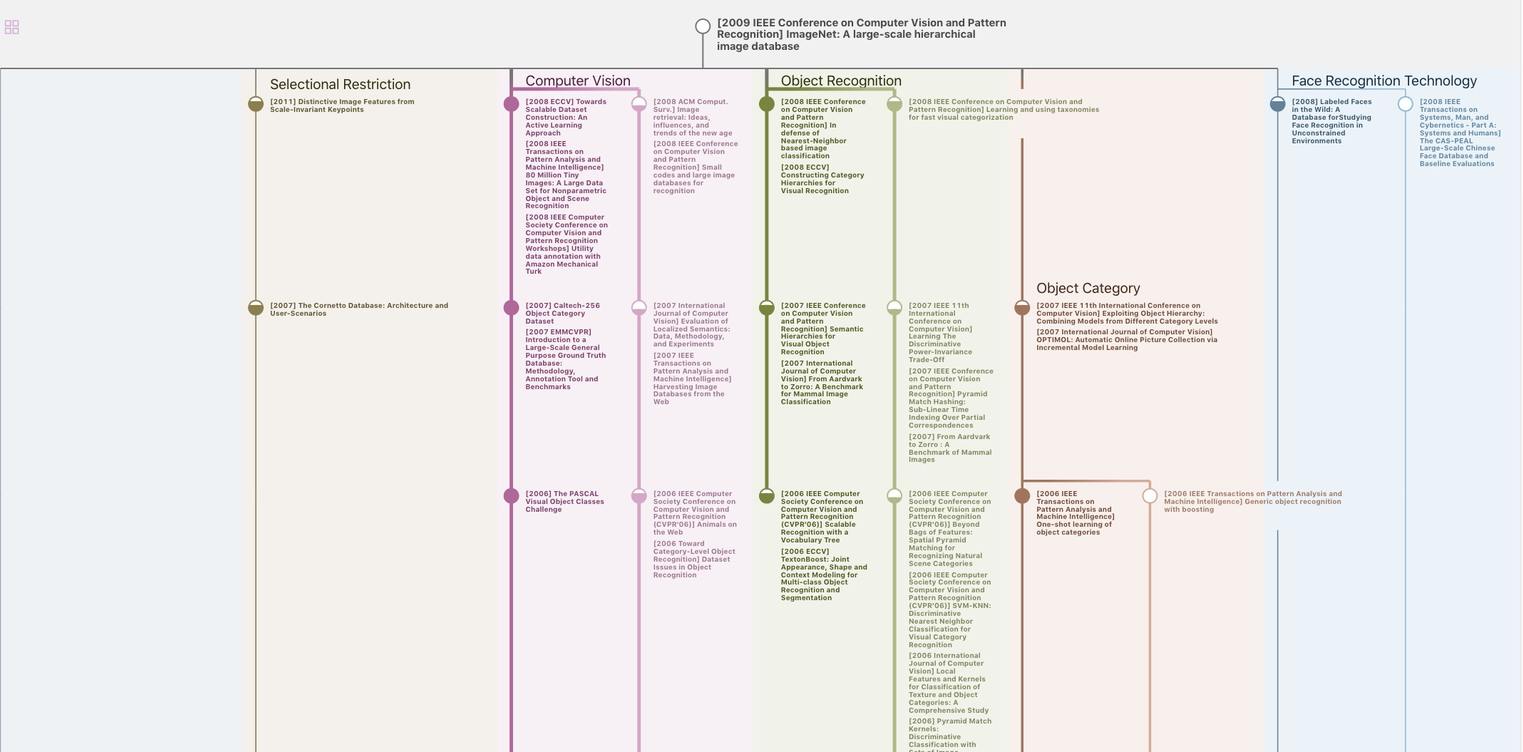
生成溯源树,研究论文发展脉络
Chat Paper
正在生成论文摘要