SemLoc: Accurate and Robust Visual Localization with Semantic and Structural Constraints from Prior Maps
IEEE International Conference on Robotics and Automation(2022)
摘要
Semantic information and geometrical structures of a prior map can be leveraged in visual localization to bound drift errors and improve accuracy. In this paper, we propose SemLoc, a pure visual localization system, for accurate localization in a prior semantic map. To tightly couple semantic and structure information from prior maps, a hybrid constraint is presented by using the Dirichlet distribution. Then, with the local landmarks and their semantic states tracked in the frontend, the camera poses and data associations are jointly optimized through Expectation-Maximization (EM) algorithm. We validate the effectiveness of our approach in both monocular and stereo modes on the public KITTI dataset. Experimental results demonstrate that our system can greatly reduce drift errors with an satisfying real-time performance. Compared with several state-of-the-art visual localization systems, the proposed framework achieves a competitive localization performance.
更多查看译文
关键词
SemLoc,robust visual localization,structural constraints,semantic information,geometrical structures,prior map,bound drift errors,pure visual localization system,prior semantic map,structure information,hybrid constraint,local landmarks,semantic states,state-of-the-art visual localization systems,competitive localization performance
AI 理解论文
溯源树
样例
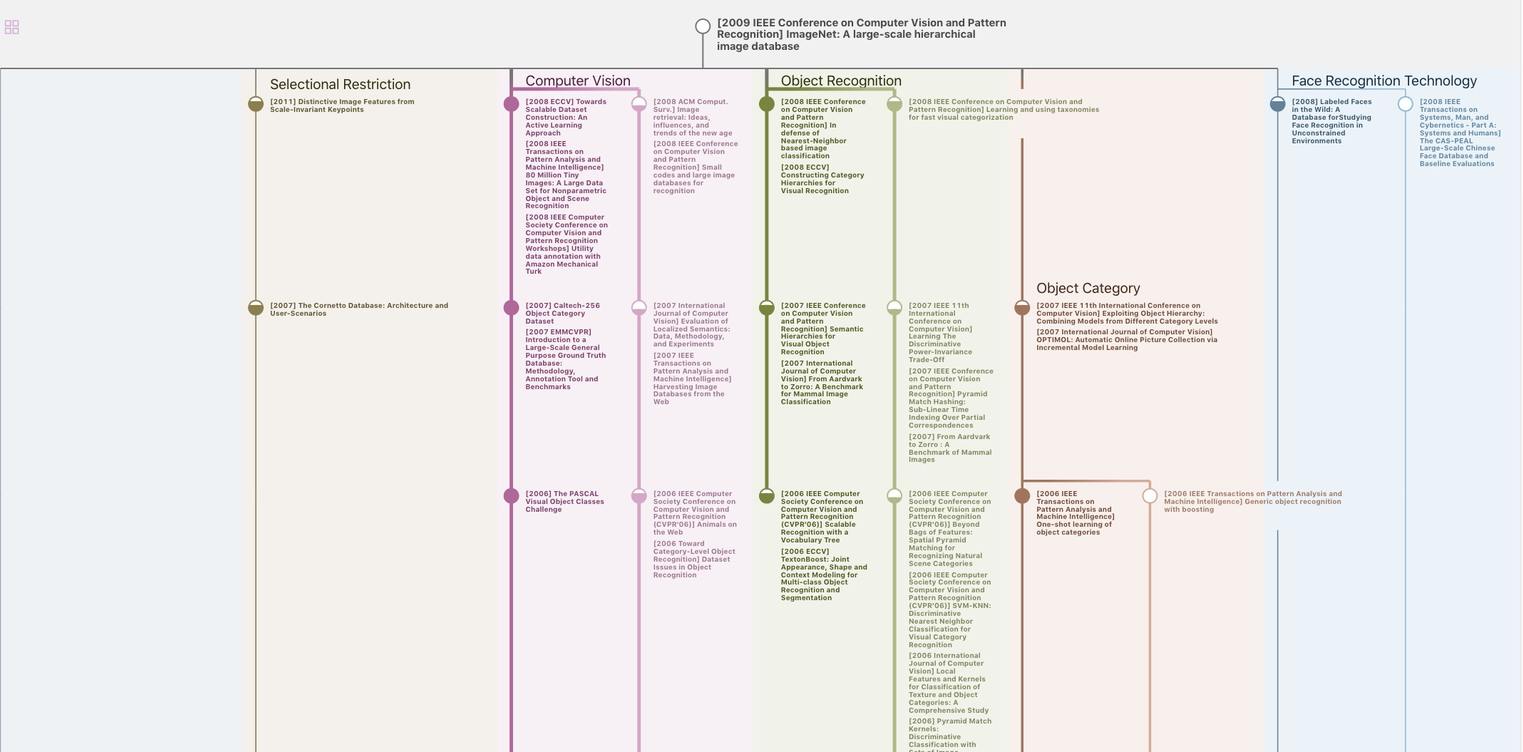
生成溯源树,研究论文发展脉络
Chat Paper
正在生成论文摘要