Fuse and Mix: MACAM-Enabled Analog Activation for Energy-Efficient Neural Acceleration.
ICCAD(2022)
摘要
Analog computing has been recognized as a promising low-power alternative to digital counterparts for neural network acceleration. However, conventional analog computing is mainly in a mixed-signal manner. Tedious analog/digital (A/D) conversion cost significantly limits the overall system's energy efficiency. In this work, we devise an efficient analog activation unit with magnetic tunnel junction (MTJ)-based analog content-addressable memory (MACAM), simultaneously realizing nonlinear activation and A/D conversion in a fused fashion. To compensate for the nascent and therefore currently limited representation capability of MACAM, we propose to mix our analog activation unit with digital activation dataflow. A fully differential framework, SuperMixer, is developed to search for an optimized activation workload assignment, adaptive to various activation energy constraints. The effectiveness of our proposed methods is evaluated on a silicon photonic accelerator. Compared to standard activation implementation, our mixed activation system with the searched assignment can achieve competitive accuracy with $>$60% energy saving on A/D conversion and activation.
更多查看译文
关键词
acceleration,macam-enabled,energy-efficient
AI 理解论文
溯源树
样例
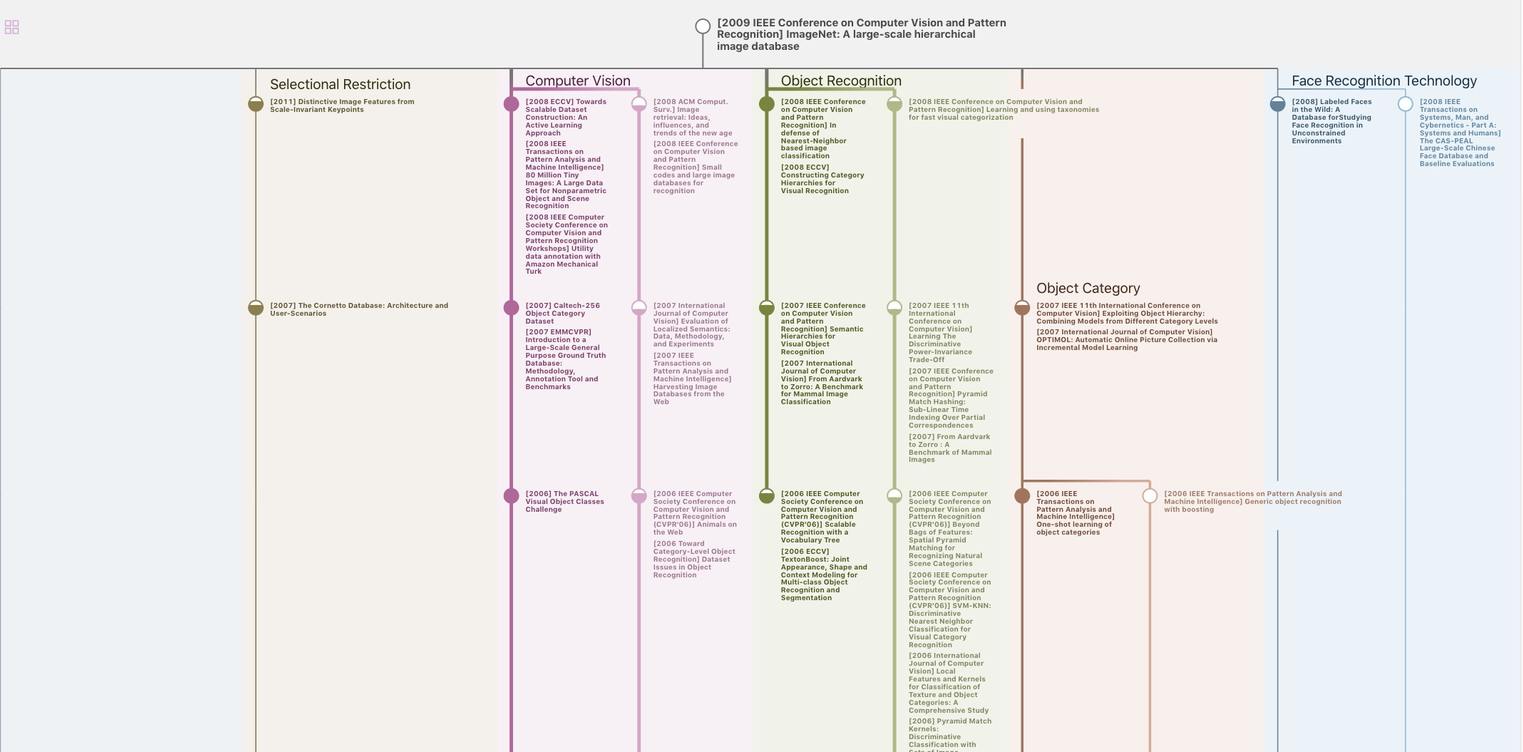
生成溯源树,研究论文发展脉络
Chat Paper
正在生成论文摘要