Fast and accurate multi-class geospatial object detection with large-size remote sensing imagery using CNN and Truncated NMS
ISPRS Journal of Photogrammetry and Remote Sensing(2022)
摘要
Multi-class geospatial object detection with remote sensing imagery has broad prospects in urban planning, natural disaster warning, industrial production, military surveillance and other applications. Accuracy and efficiency are two common measures for evaluating object detection models, and it is often difficult to achieve both at the same time. Developing a practical remote sensing object detection algorithm that balances the accuracy and efficiency is thus a big challenge in the Earth observation community. Here, we propose a comprehensive high-speed multi-class remote sensing object detection method. Firstly, we obtain a multi-volume YOLO (You Only Look Once) v4 model for balancing speed and accuracy, based on a pruning strategy of the convolutional neural network (CNN) and the one-stage object detection network YOLO v4. Moreover, we apply the Manhattan-Distance Intersection of Union (MIOU) loss function to the multi-volume YOLO v4 to further improve the accuracy without additional computational burden.
更多查看译文
关键词
Multi-class geospatial object detection,Convolutional neural network,Truncated NMS,Manhattan-Distance IOU
AI 理解论文
溯源树
样例
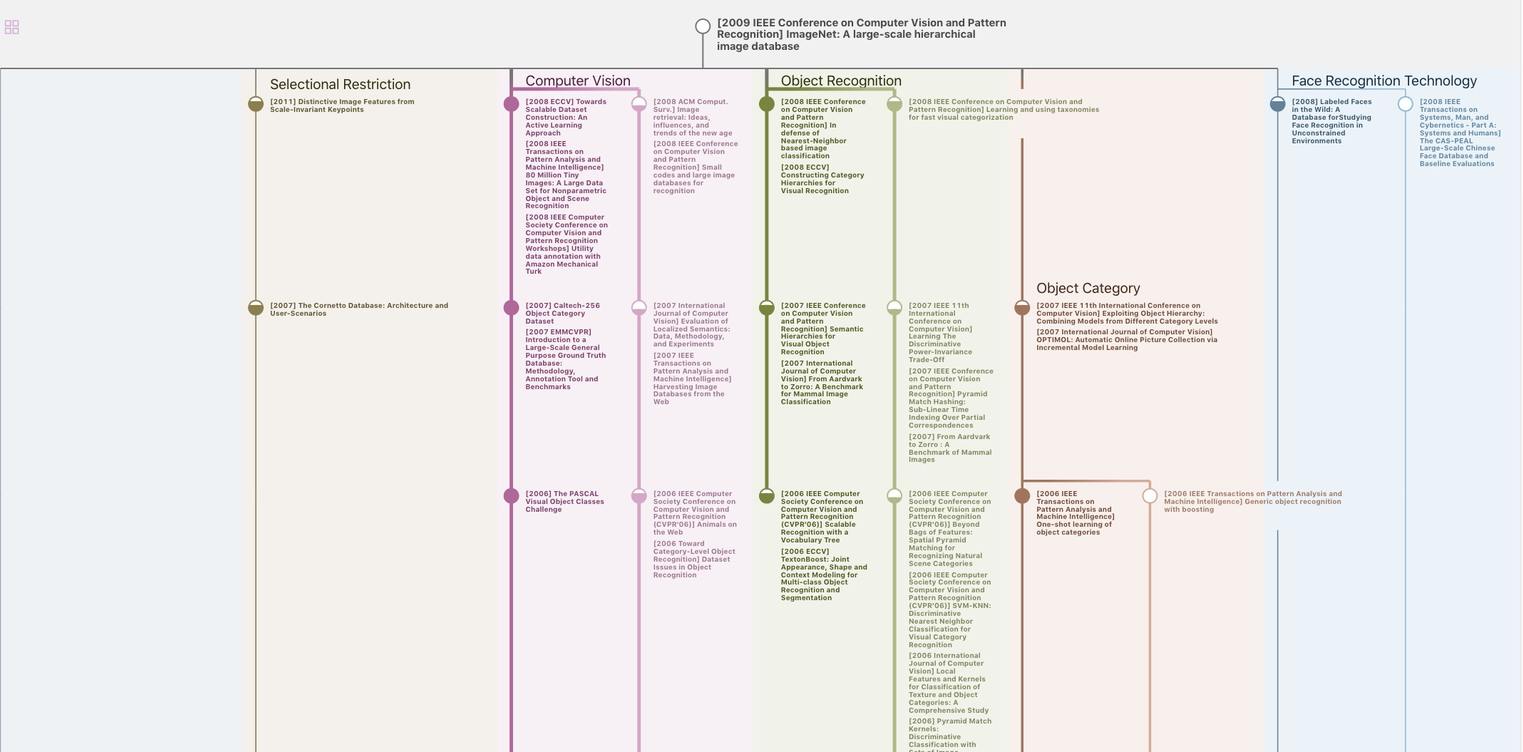
生成溯源树,研究论文发展脉络
Chat Paper
正在生成论文摘要