A hybrid deep reinforcement learning ensemble optimization model for heat load energy-saving prediction
Journal of Building Engineering(2022)
摘要
Accurate heat load forecasting is crucial to achieving feed-forward control and on-demand heat supply in the district heating system (DHS). However, accurate forecasting is difficult to achieve effective energy-saving because experimental data are often not optimal or the most energy-efficient. In this study, a hybrid heat load forecasting model with energy-saving consideration is proposed, which consists of similar sample selection approach, short-term forecasting model pool and deep reinforcement learning ensemble strategy. In similar sample selection module, a novel weighted Euclidean norm (EN) is used to select suitable similar samples set for the training samples set. In model pool module, six popular deterministic prediction models are constructed. For energy-saving consideration, the average of minimum and actual heat load is selected as the training target in the training samples set and similar samples set. In ensemble module, deep deterministic policy gradient (DDPG) is used to integrate the prediction results of the base predictors. In the case study, real data from a heat exchange station in Tianjin are used to perform the prediction of heat load 24 h, 72 h and 168 h, respectively. Experimental results show that: (a) The proposed hybrid model is able to capture the change of heat load. The average root mean square error for different prediction periods are 0.0825 MW, 0.1007 MW and 0.1152 MW, respectively; (b) The proposed hybrid model can reduce energy consumption. The energy-saving rate for different periods are 5.33%, 5.31% and 5.07%, respectively.
更多查看译文
关键词
District heating system,Heat load energy-saving prediction,Similar sample selection approach,Weighted Euclidean norm,Deep deterministic policy gradient
AI 理解论文
溯源树
样例
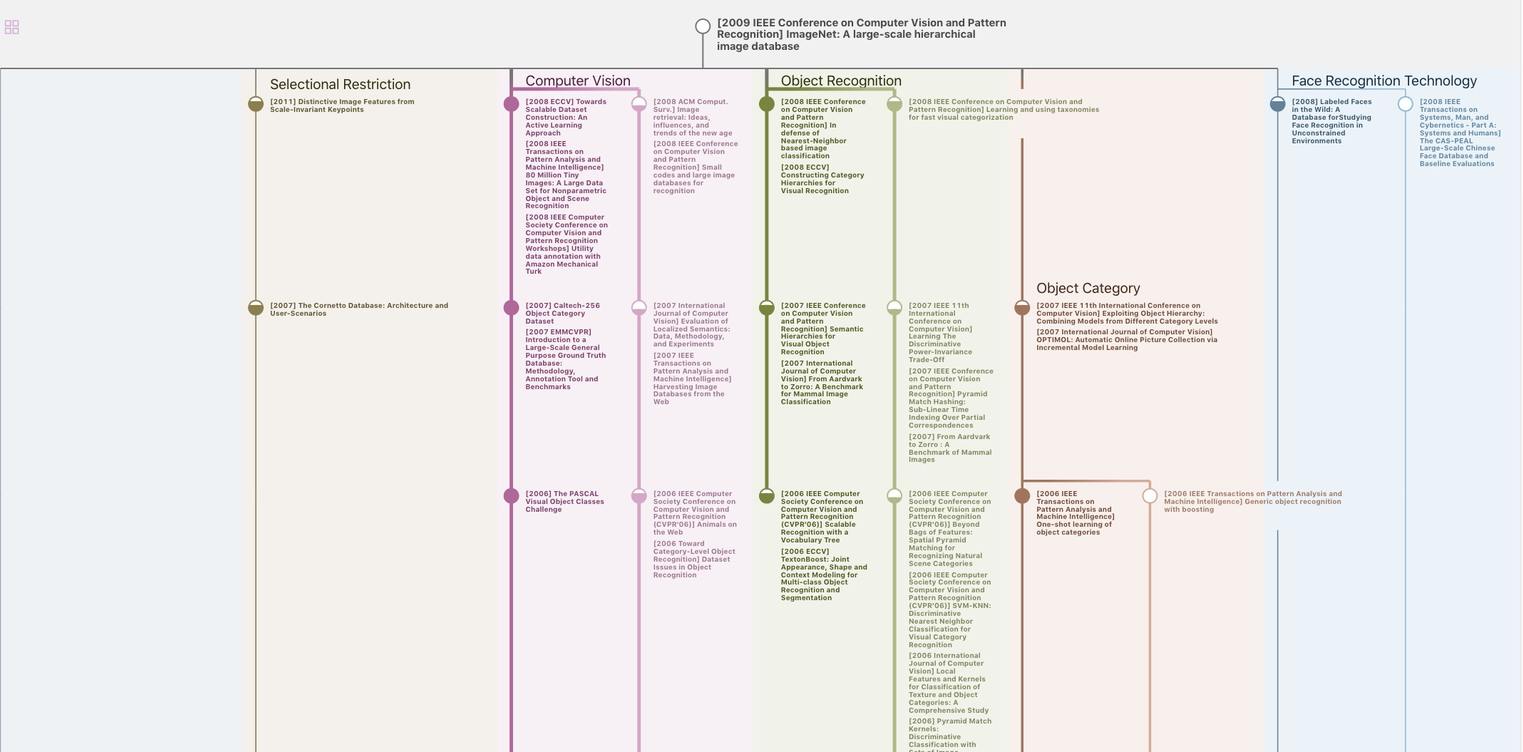
生成溯源树,研究论文发展脉络
Chat Paper
正在生成论文摘要