Generalization in Distant Regions of a Rule-Described Category Space: a Mixed Exemplar and Logical-Rule-Based Account
Computational Brain & Behavior(2022)
摘要
An important question in the cognitive-psychology of category learning concerns the manner in which observers generalize their trained category knowledge at time of transfer. In recent work, Conaway and Kurtz (Conaway and Kurtz, Psychonomic Bulletin & Review 24:1312–1323, 2017) reported results from a novel paradigm in which participants learned rule-described categories defined over two dimensions and then classified test items in distant transfer regions of the category space. The paradigm yielded results that challenged the predictions from both exemplar-based and logical-rule-based models of categorization but that the authors suggested were as predicted by a divergent auto-encoder (DIVA) model (Kurtz, Psychonomic Bulletin & Review 14:560–576, 2007, Kurtz, Psychology of learning and motivation, Academic Press, New York, 2015). In this article, we pursue these challenges by conducting replications and extensions of the original experiment and fitting a variety of computational models to the resulting data. We find that even an extended version of the exemplar model that makes allowance for learning-during-test (LDT) processes fails to account for the results. In addition, models that presume a mixture of salient logical rules also fail to account for the findings. However, as a proof of concept, we illustrate that a model that assumes a mixture of strategies across subjects—some relying on exemplar-based memories with LDT, and others on salient logical rules—provides an outstanding account of the data. By comparison, DIVA performs considerably worse than does this LDT-exemplar-rule mixture account. These results converge with past ones reported in the literature that point to multiple forms of category representation as well as to the role of LDT processes in influencing how observers generalize their category knowledge.
更多查看译文
关键词
Categorization, Generalization, Exemplar models, Rule models, Computational modeling
AI 理解论文
溯源树
样例
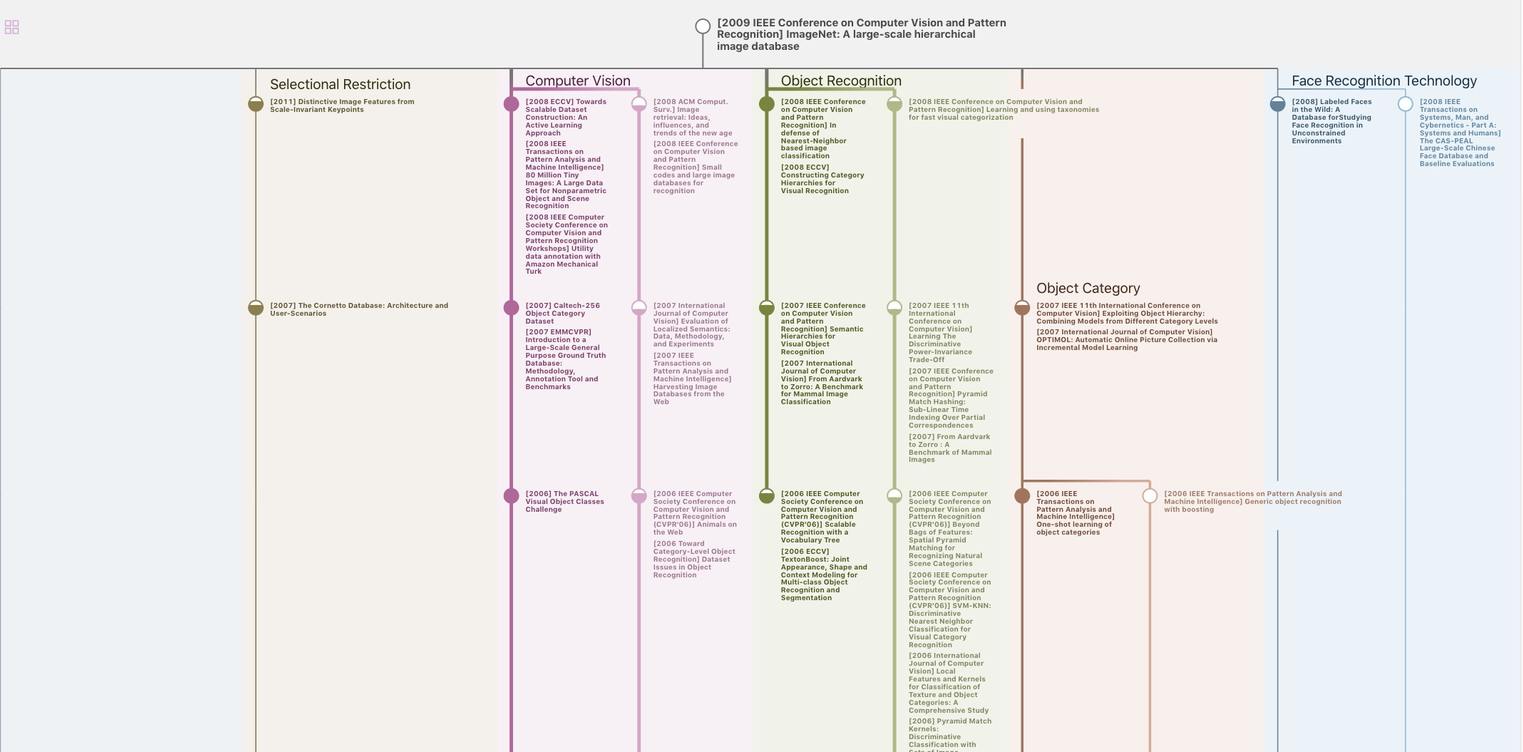
生成溯源树,研究论文发展脉络
Chat Paper
正在生成论文摘要