Racial Fairness in Precision Medicine: Pediatric Asthma Prediction Algorithms
AMERICAN JOURNAL OF HEALTH PROMOTION(2023)
摘要
Purpose: Quantify and examine the racial fairness of two widely used childhood asthma predictive precision medicine algorithms: the asthma predictive index (API) and the pediatric asthma risk score (PARS). Design: Apply the API and PARS and evaluate model performance overall and when stratified by race. Setting: Cincinnati, OH, USA. Subjects: A prospective birth cohort of 590 children with clinically measured asthma diagnosis by age seven. Measures: Model diagnostic criteria included sensitivity, specificity, positive predictive value (PPV), and negative predictive value (NPV). Analysis: Significant differences in model performance between Black and white children were considered to be present if the P-value associated with a t-test based on 100 bootstrap replications was less than.05. Results: Compared to predictions for white children, predictions for Black children using the PARS had a higher sensitivity (. 88 vs.57), lower specificity (.55 vs.83), higher PPV (. 42 vs.33), but a similar NPV (.93 vs.93). Within the API and compared to predictions for white children, predictions for Black children had a higher sensitivity (.63 vs.53), similar specificity (.81 vs.80), higher PPV (. 54 vs.28), and lower NPV (.86 vs.92). Conclusions: Overall, racial disparities in model diagnostic criteria were greatest for sensitivity and specificity in the PARS, but racial disparities existed in three of the four criteria for both the PARS and the API.
更多查看译文
关键词
racial minority,fairness,asthma,precision medicine,pediatric
AI 理解论文
溯源树
样例
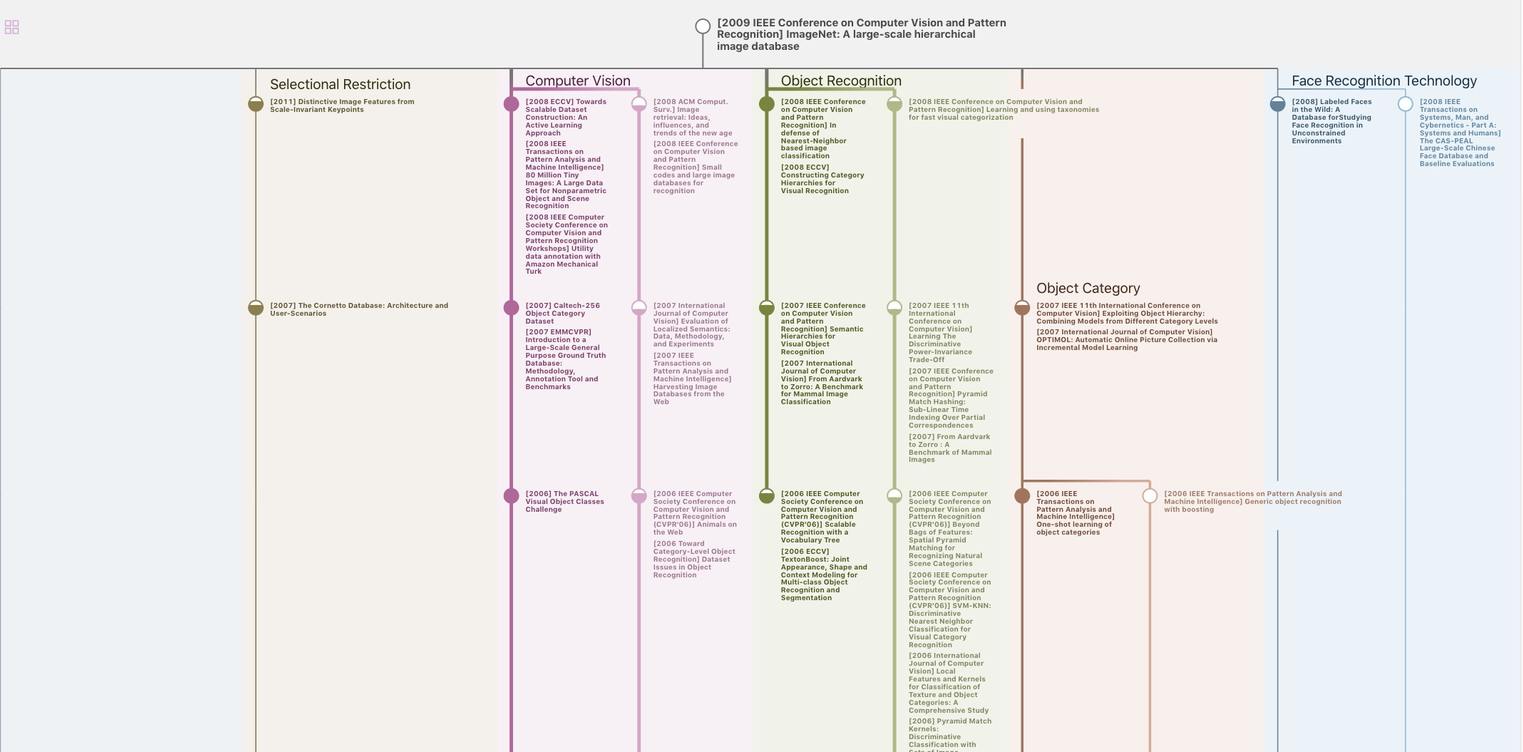
生成溯源树,研究论文发展脉络
Chat Paper
正在生成论文摘要