Superpixel Spectral-Spatial Feature Fusion Graph Convolution Network for Hyperspectral Image Classification
IEEE TRANSACTIONS ON GEOSCIENCE AND REMOTE SENSING(2022)
摘要
Recently, convolutional neural networks (CNNs) have demonstrated impressive capabilities in the representation and classification of hyperspectral remote sensing images. Traditional CNNs require massive data to sufficiently train the network. To tackle this problem, graph convolutional network (GCN) has been introduced for hyperspectral image classification. GCN methods usually construct the graph from either spectral or spatial domain, which has not adequately explored the information in the joint spectral-spatial domain. In this article, we propose a superpixel spectral-spatial feature fusion graph convolution network for hyperspectral image classification (S3FGCN). S3FGCN can comprehensively use information in spectral, spatial, and spectral-spatial domains with limited data. Moreover, to enhance the performance, we explore a shared weights' GCN in the spectral-spatial domain. To further improve the efficiency, superpixels are used to construct the adjacency matrix. Finally, dynamic sampling is adopted to make the model focus more on difficult samples. In the experiments on four datasets, S3FGCN demonstrates better accuracy compared with the state-of-the-art hyperspectral image classification methods.
更多查看译文
关键词
Hyperspectral imaging,Feature extraction,Image segmentation,Data mining,Convolution,Computational modeling,Training,Dynamic sampling,graph convolutional network (GCN),hyperspectral image classification,spectral-spatial feature fusion
AI 理解论文
溯源树
样例
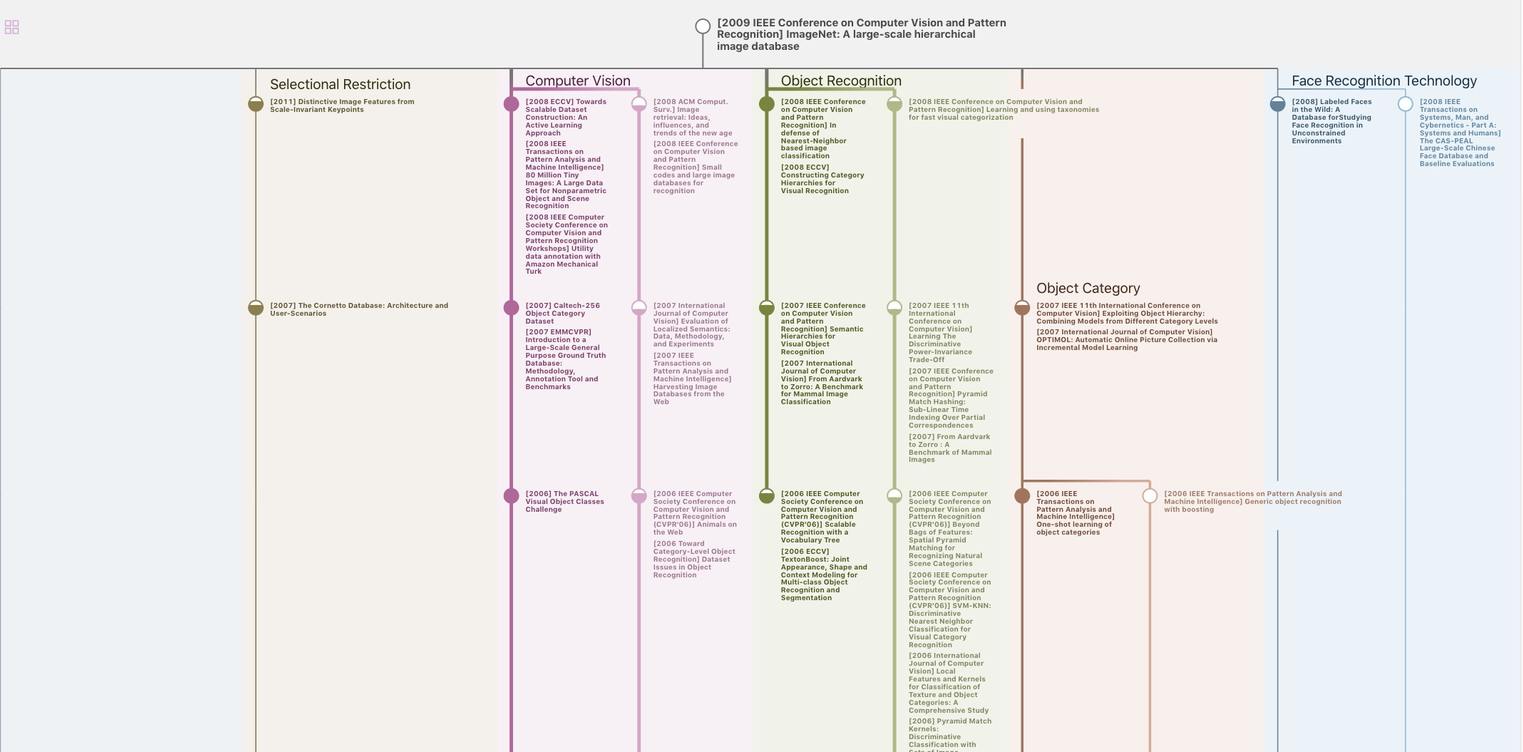
生成溯源树,研究论文发展脉络
Chat Paper
正在生成论文摘要