Interventional Recommendation with Contrastive Counterfactual Learning for Better Understanding User Preferences
arxiv(2022)
摘要
Recently, there has been a surging interest in formulating recommendations in the context of causal inference. The studies regard the recommendation as an intervention in causal inference and frame the users' preferences as interventional effects to improve recommender systems' generalization. Many studies in the field of causal inference for recommender systems have been focusing on utilizing propensity scores from the causal community that reduce the bias while inducing additional variance. Alternatively, some studies suggest the existence of a set of unbiased data from randomized controlled trials while it requires to satisfy certain assumptions that may be challenging in practice. In this paper, we first design a causal graph representing recommender systems' data generation and propagation process. Then, we reveal that the underlying exposure mechanism biases the maximum likelihood estimation (MLE) on observational feedback. In order to figure out users' preferences in terms of causality behind data, we leverage the back-door adjustment and do-calculus, which induces an interventional recommendation model (IREC). Furthermore, considering the confounder may be inaccessible for measurement, we propose a contrastive counterfactual learning method (CCL) for simulating the intervention. In addition, we present two extra novel sampling strategies and show an intriguing finding that sampling from counterfactual sets contributes to superior performance. We perform extensive experiments on two real-world datasets to evaluate and analyze the performance of our model IREC-CCL on unbiased test sets. Experimental results demonstrate our model outperforms the state-of-the-art methods.
更多查看译文
AI 理解论文
溯源树
样例
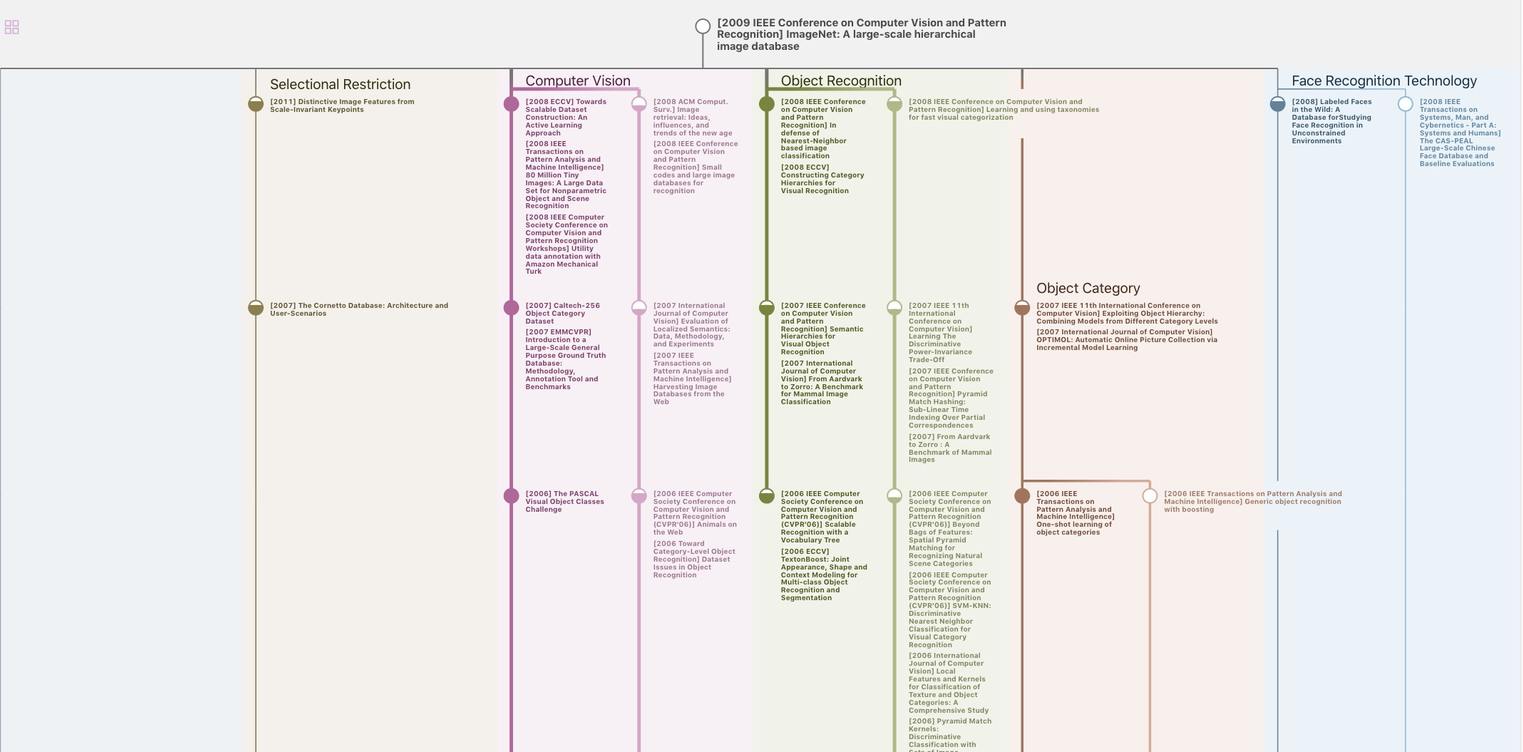
生成溯源树,研究论文发展脉络
Chat Paper
正在生成论文摘要