Partially Relaxed Masks for Knowledge Transfer Without Forgetting in Continual Learning
ADVANCES IN KNOWLEDGE DISCOVERY AND DATA MINING, PAKDD 2022, PT I(2022)
摘要
The existing research on continual learning (CL) has focused mainly on preventing catastrophic forgetting. In the task-incremental learning setting of CL, several approaches have achieved excellent results, with almost no forgetting. The goal of this work is to endow such systems with the additional ability to transfer knowledge when the tasks are similar and have shared knowledge to achieve higher accuracy. Since the existing system HAT is one of most effective task-incremental learning algorithms, this paper extends HAT with the aim of both objectives, i.e., overcoming catastrophic forgetting and transferring knowledge among tasks without introducing additional mechanisms into the architecture of HAT. The current study finds that task similarity, which indicates knowledge sharing and transfer, can be computed via the clustering of task embeddings optimized by HAT. Thus, we propose a new approach, named "partially relaxed masks" (PRM), to exploit HAT's masks to not only keep some parameters from being modified in learning subsequent tasks as much as possible to prevent forgetting but also enable remaining parameters to be updated to facilitate knowledge transfer. Extensive experiments demonstrate that PRM performs competitively compared with the latest baselines while also requiring much less computation time.
更多查看译文
关键词
Continual learning, Task similarity, Catastrophic forgetting
AI 理解论文
溯源树
样例
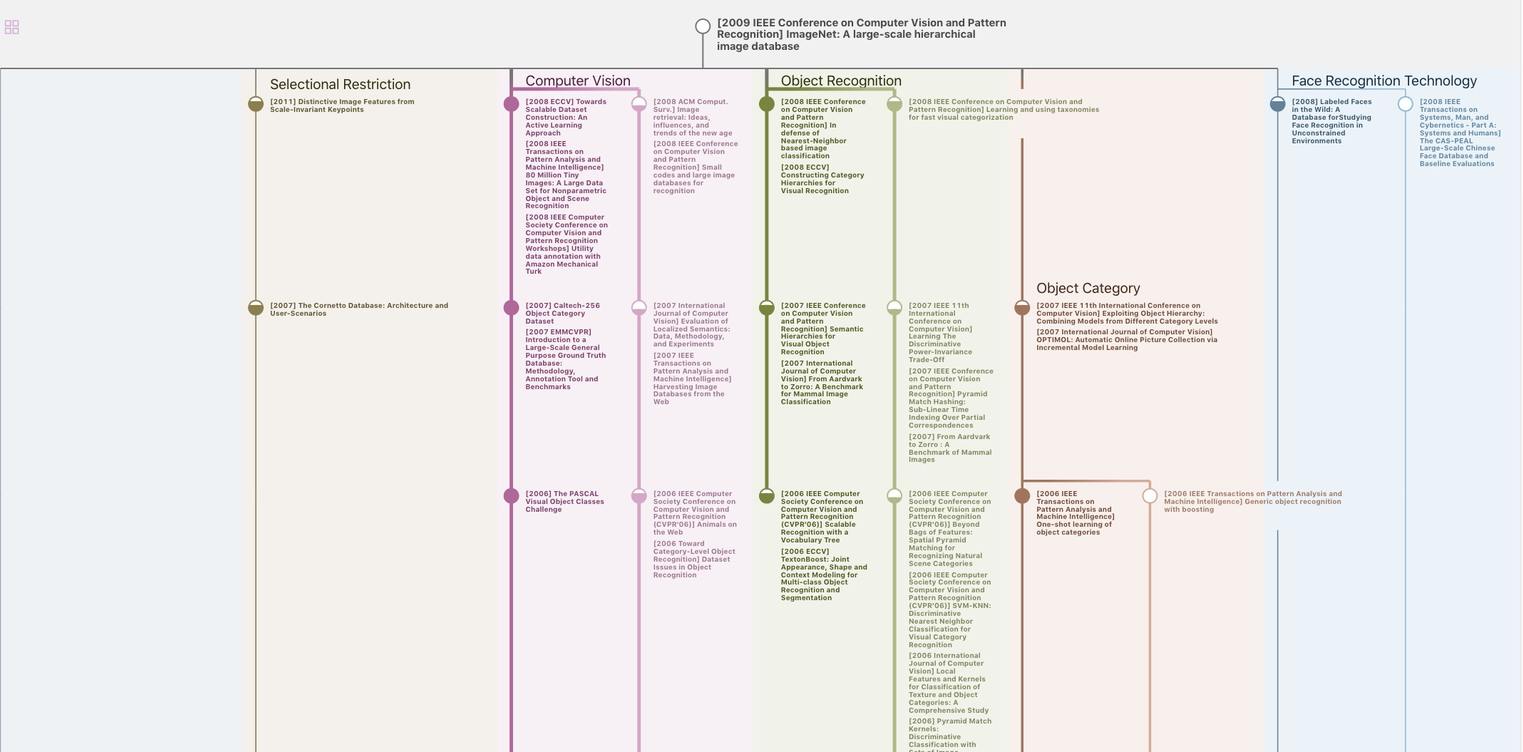
生成溯源树,研究论文发展脉络
Chat Paper
正在生成论文摘要