From adversarial examples to data poisoning instances: utilizing an adversarial attack method to poison a transfer learning model
ICC 2022 - IEEE International Conference on Communications(2022)
摘要
Despite the wide-ranging applicability of machine learning methods, they are vulnerable to security attacks, such as evasion attacks and triggerless data poisoning attacks. An evasion attack occurs at the inference time when an attacker feeds in an adversarial example, a malicious perturbed input that appears the same as its untampered copy to a human oracle. In contrast, a triggerless data poisoning attack occurs at training time. An attacker tries to subvert learning with injected poisoned instances. In this research, we focus on a special sub-category of data poisoning attacks, namely triggerless clean-label targeted data poisoning attacks. This type of attacks is more realistic in the sense that it does not require an attacker to have the ability to change the label of any training instance. That is, attackers can successfully attack the training process with a poison instance that is correctly labeled by a human oracle. We propose a simple but effective way to alter an adversarial attack method into a triggerless clean-label targeted data poisoning attack method with a remarkable attack success rate. Furthermore, our proposed method only requires the injection of a single poison instance to manipulate a transfer learning model to misclassify an untampered targeted instance. We compare our method with a popular one-shot attack and show that our method is easier to be used as we do not need to tune for a hyperparameter such as a similarity coefficient.
更多查看译文
关键词
Machine Learning (ML),Deep Learning (DL),Transfer Learning (TL),Security,Data Poisoning Attack
AI 理解论文
溯源树
样例
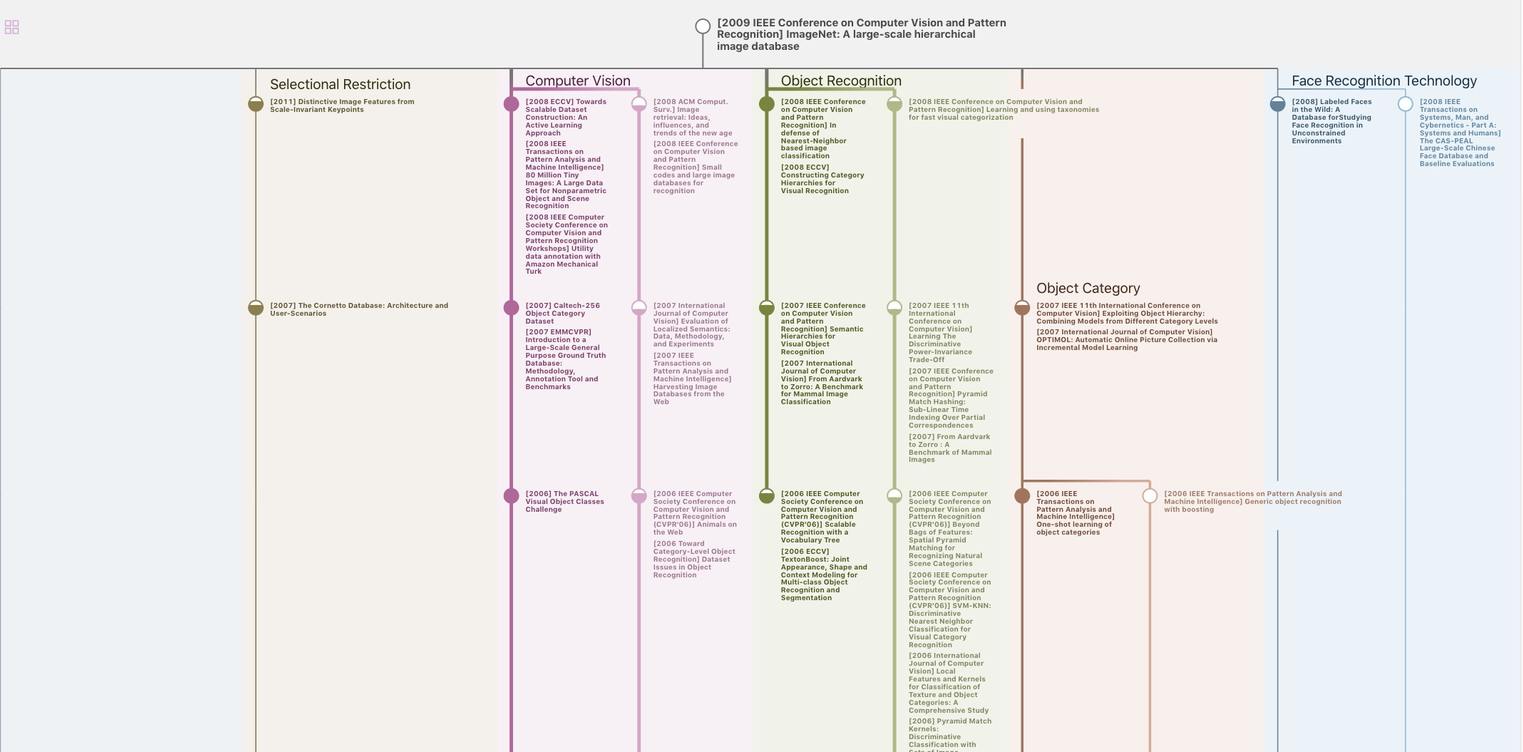
生成溯源树,研究论文发展脉络
Chat Paper
正在生成论文摘要