Saline soil moisture mapping using Sentinel-1A synthetic aperture radar data and machine learning algorithms in humid region of China's east coast
CATENA(2022)
摘要
Accurate and timely information on soil moisture (SM) is crucial for better understanding the hydrological and ecological processes in humid saline regions. This study investigated the potential of using Sentinel-1A Synthetic Aperture Radar (SAR) imagery and Machine Learning Algorithms for SM mapping in China's east coast. This study used the recursive feature elimination (RFE) technique to select optimum SAR indices from the back-scattering coefficients of the sampling date as well as the averaged backscattering coefficients of the neighbouring dates. The newly developed support vector regression (SVR) model provided more accurate SM estimation than that from the random forest regression (RFR) and multivariate linear regression (MLR) models. By using the leave-one-out cross-validation (LOOCV), the achieved coefficient of determination (R-2), root mean square error (RMSE), and the relative RMSE (RRMSE) in SM estimation were 0.77, 1.82%, and 10.29%, respectively. The SVR model was able to map the SM effectively over a large area under the condition that the information of soil surface roughness, soil salinity, and land cover types was unknown. Results from this study demonstrate that high-temporal-resolution Sentinel-1A SAR, with its free-accessibility, offers a great source for the SVR model to provide frequent and accurate SM mapping over coastal saline regions.
更多查看译文
关键词
Soil moisture estimation, Sentinel-1A, Synthetic aperture radar (SAR), Machine learning, Saline coastal region
AI 理解论文
溯源树
样例
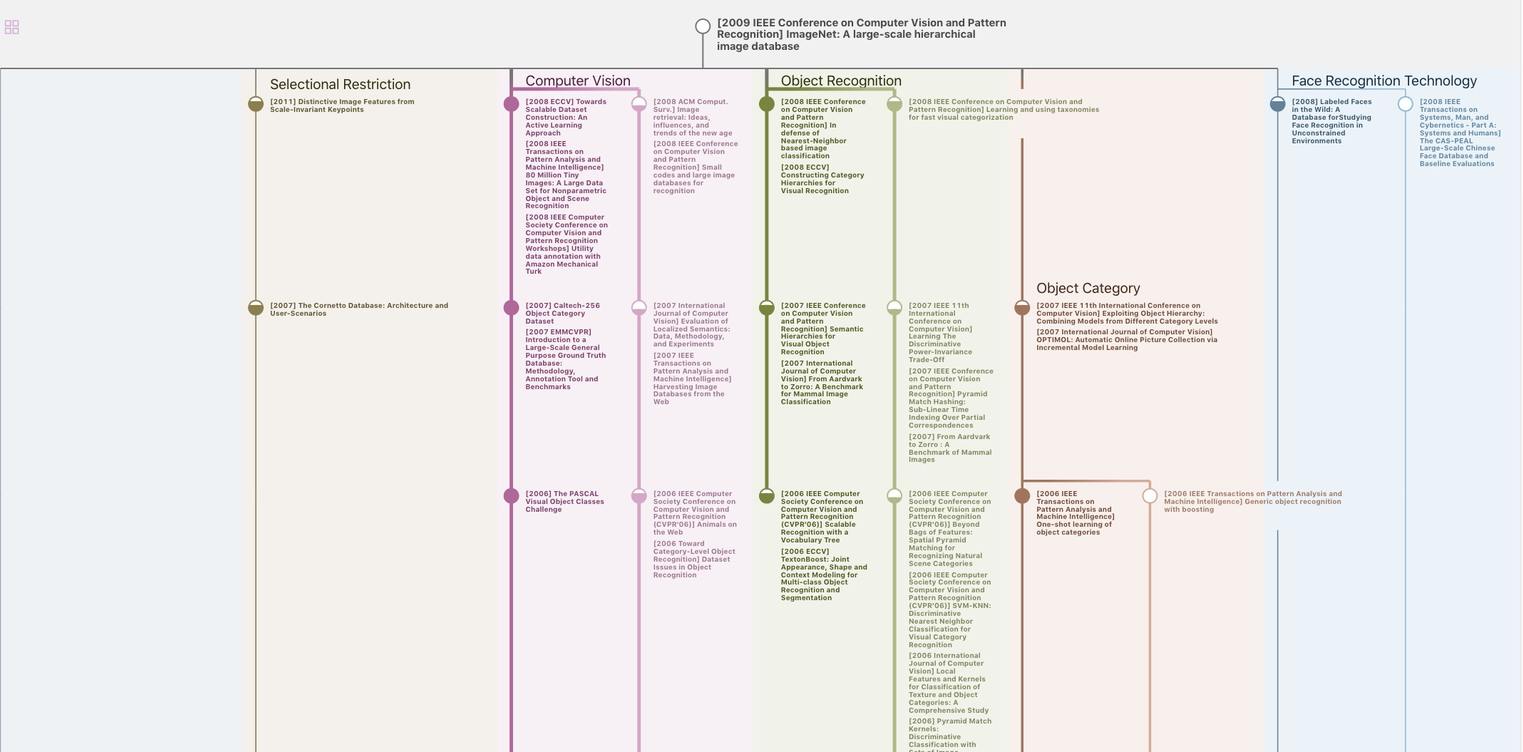
生成溯源树,研究论文发展脉络
Chat Paper
正在生成论文摘要