On accurate prediction of cloud workloads with adaptive pattern mining
JOURNAL OF SUPERCOMPUTING(2022)
摘要
Resource provisioning for cloud computing requires adaptive and accurate prediction of cloud workloads. However, existing studies in workload prediction have faced significant challenges in predicting time-varying cloud workloads of diverse trends and patterns, and the lack of accurate prediction often results in resource waste and violation of Service-Level Agreements (SLAs). We propose a bagging-like ensemble framework for cloud workload prediction with Adaptive Pattern Mining (APM). Within this framework, we first design a two-step method with various models to simultaneously capture the “low frequency” and “high frequency” characteristics of highly variable workloads. For a given workload, we further develop an error-based weights aggregation method to integrate the prediction results from multiple pattern-specific models into a final result to predict a future workload. We conduct experiments to demonstrate the efficacy of APM in workload prediction with various prediction lengths using two real-world workload traces from Google and Alibaba cloud data centers, which are of different types. Extensive experimental results show that APM achieves above 19.62% improvement over several classic and state-of-the-art workload prediction methods for highly variable real-world cloud workloads.
更多查看译文
关键词
Cloud computing,Workload prediction,Pattern mining,Ensemble method
AI 理解论文
溯源树
样例
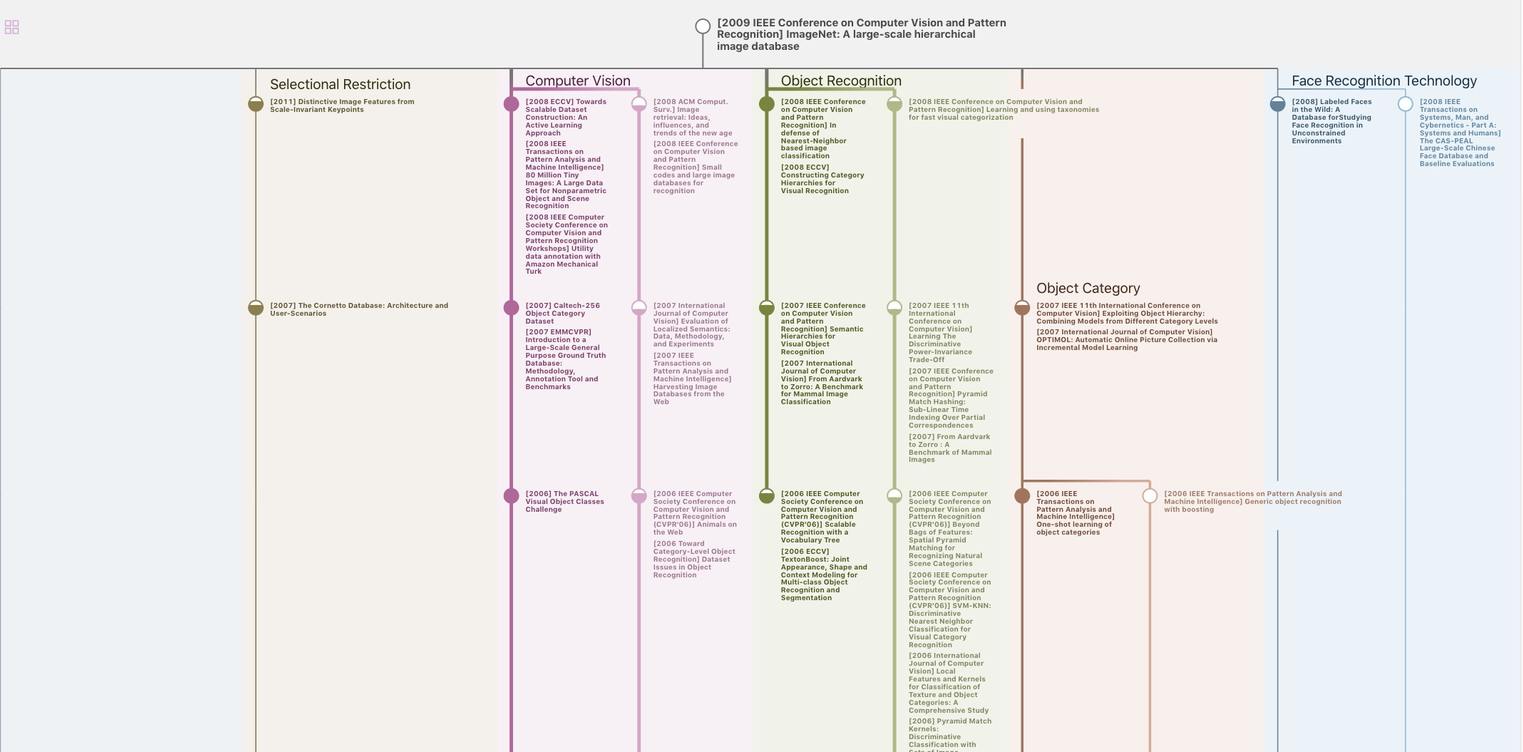
生成溯源树,研究论文发展脉络
Chat Paper
正在生成论文摘要