Adaptive Meta Transfer Learning with Efficient Self-Attention for Few-Shot Bearing Fault Diagnosis
NEURAL PROCESSING LETTERS(2022)
摘要
The success of these meta-learning methods in few-shot bearing fault diagnosis depends strictly on the assumption that the meta-training set and the meta-testing set share the same distribution, which inevitably leads to undesirable performance degradation in varying working conditions. In this paper, we propose a novel Adaptive Siamese-based Meta Transfer Learning Networks (ASTN) for cross-domain fault diagnosis with limited data. Our algorithm learns meta-knowledge from episodic metric meta-learning and further adapts the prior experience to the target domain with a transfer learning strategy. The experiment results demonstrate that our proposed approach outperforms a series of existing meta-learning methods with fewer auxiliary samples. Meanwhile, with the increment of the complexity in the meta-training set, the proposed ASTN achieves good cross-domain generalization.
更多查看译文
关键词
Bearing fault diagnosis,Meta-learning,Domain shift problem,Few-shot learning
AI 理解论文
溯源树
样例
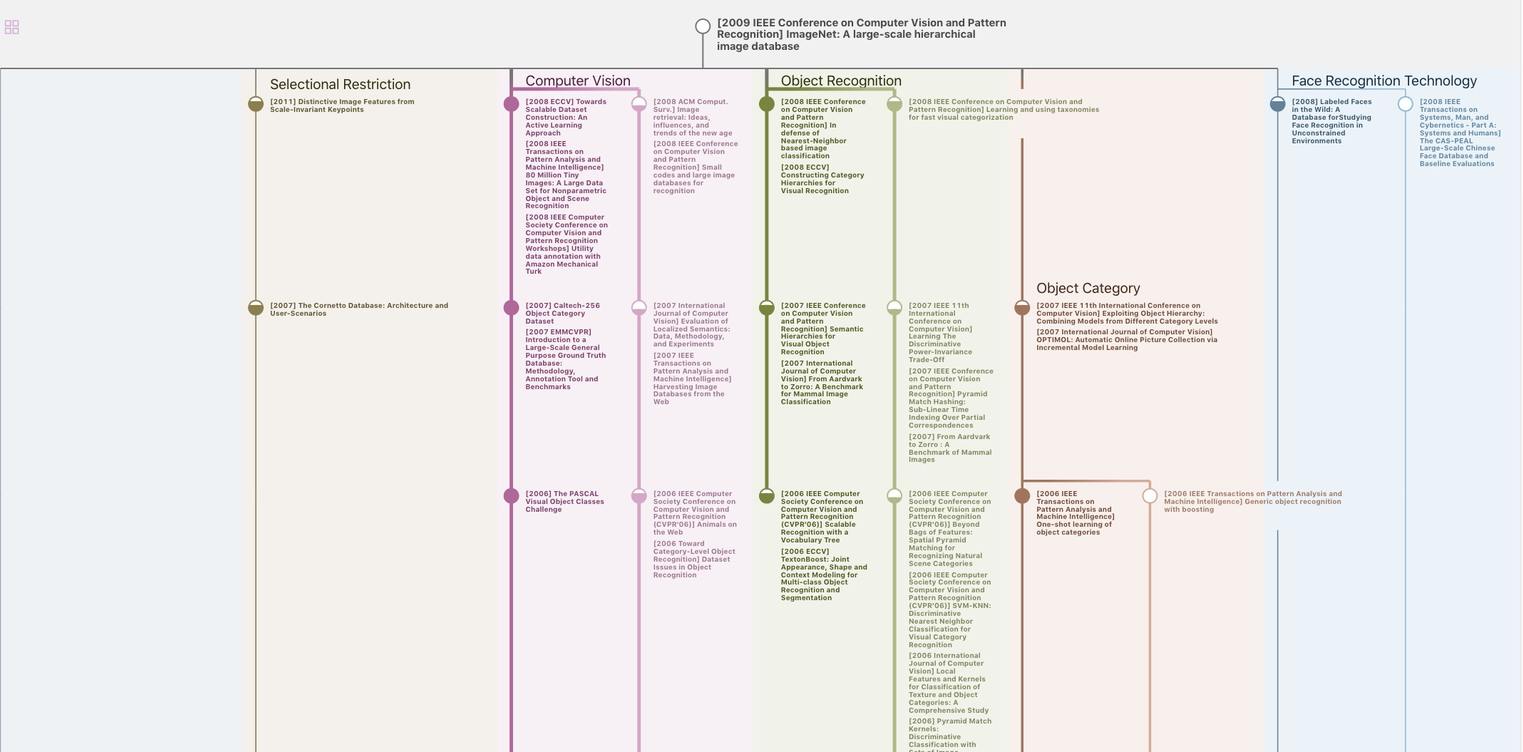
生成溯源树,研究论文发展脉络
Chat Paper
正在生成论文摘要