A Comparative Study of Generative Adversarial Networks for Text-to-Image Synthesis
INTERNATIONAL JOURNAL OF SOFTWARE SCIENCE AND COMPUTATIONAL INTELLIGENCE-IJSSCI(2022)
摘要
Text-to-picture alludes to the conversion of a textual description into a semantically similar image. The automatic synthesis of top-quality pictures from text portrayals is both exciting and useful at the same time. Current AI systems have shown significant advances in the field, but the work is still far from complete. Recent advances in the field of deep learning have resulted in the introduction of generative models that are capable of generating realistic images when trained appropriately. In this paper, the authors will review the advancements in architectures for solving the problem of image synthesis using a text description. They begin by studying the concepts of the standard GAN. How the DCGAN has been used for the task at hand is followed by the StackGAN which uses a stack of two GANs to generate an image through iterative refinement and StackGAN++ which uses multiple GANs in a tree-like structure making the task of generating images from the text more generalized. They look at the AttnGAN which uses an attentional model to generate sub-regions of an image based on the description.
更多查看译文
关键词
Deep Learning, GANs, Neural Networks, Text-to-Image
AI 理解论文
溯源树
样例
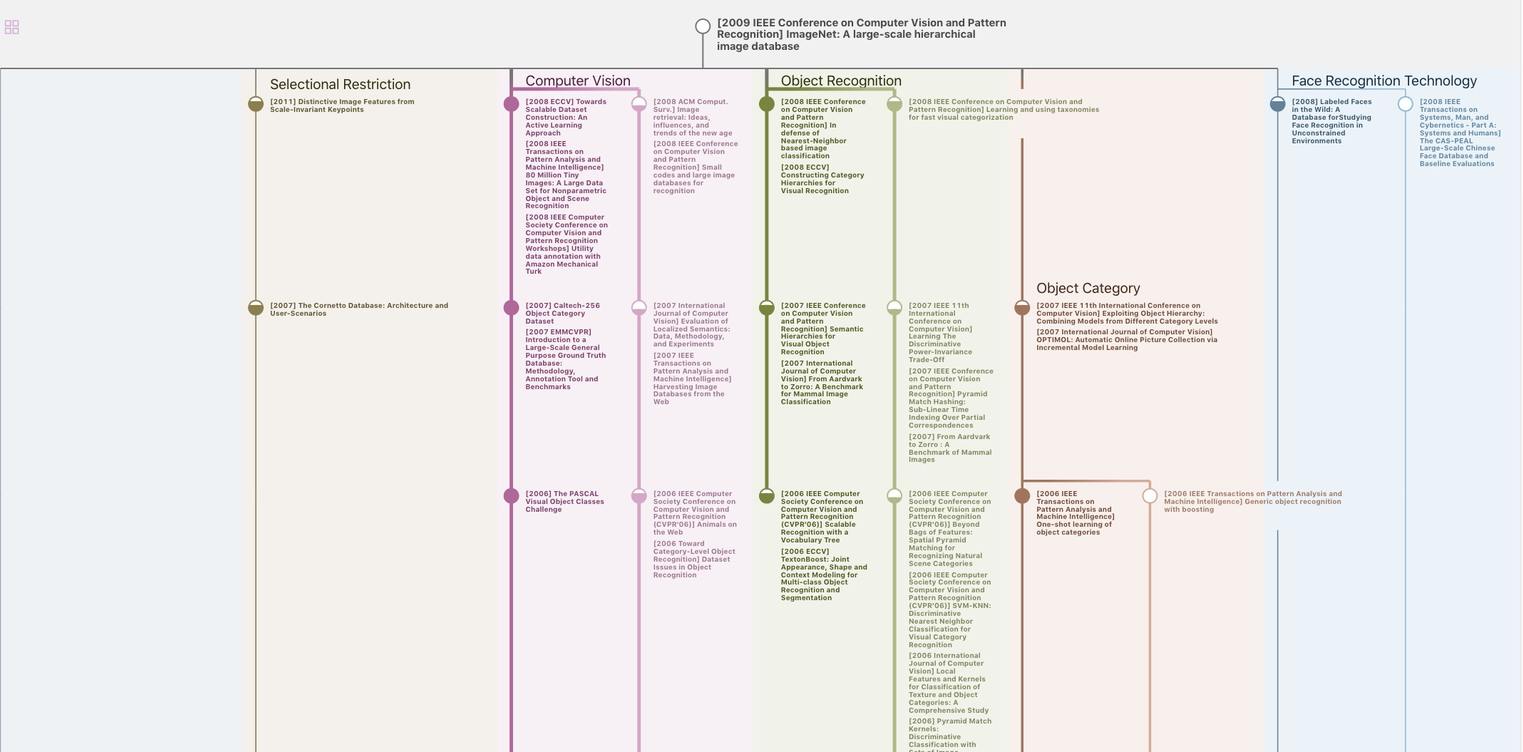
生成溯源树,研究论文发展脉络
Chat Paper
正在生成论文摘要